AI For Financial Document Processing In 2024
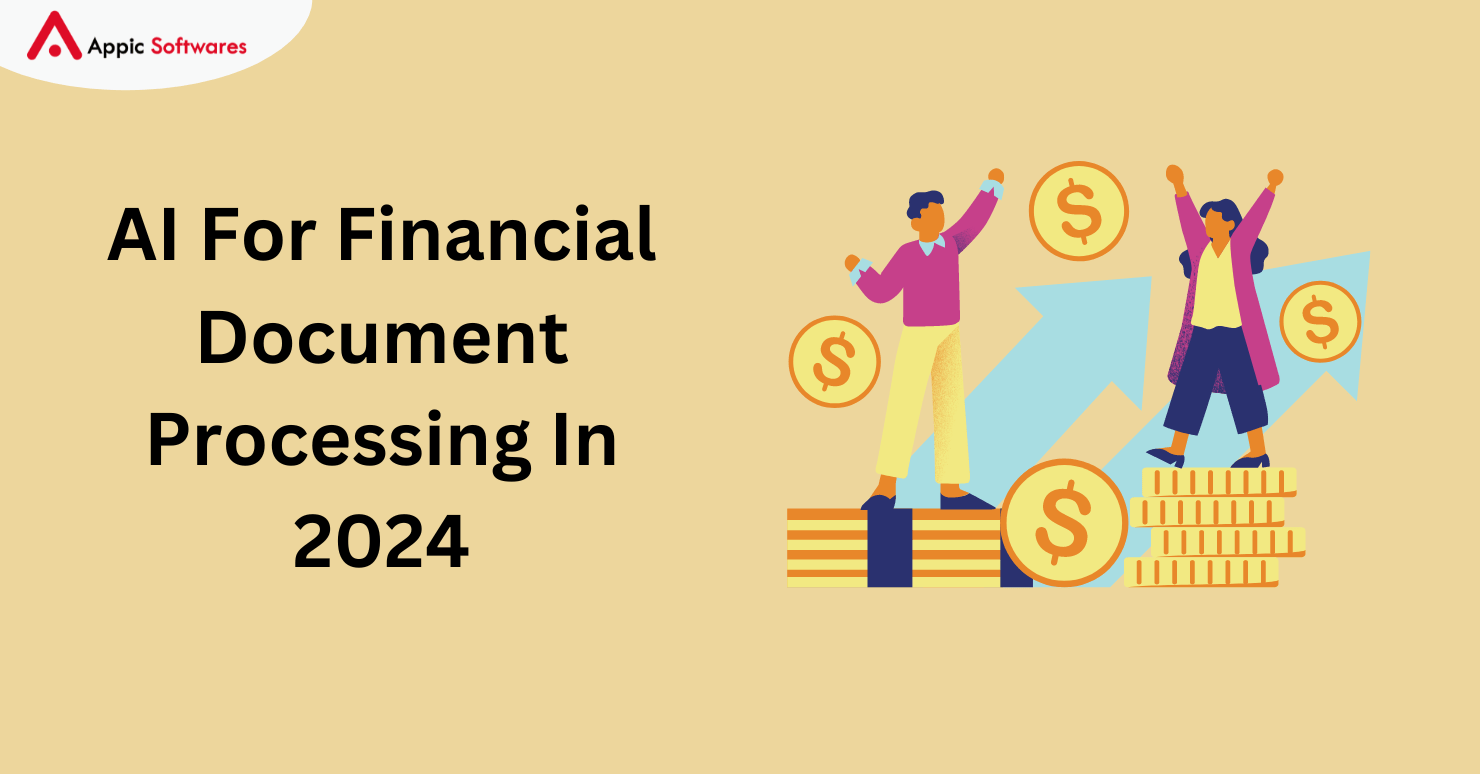
Financial institutions face a significant difficulty in the dynamic world of modern finance, where data plays a crucial role: successfully managing a steady influx of papers that saturate their everyday operations. These records include complex yearly reports as well as comprehensive transaction logs. Quickly retrieving important data from this massive archive of documents is not only essential, but also strategically critical. This is the exact point at which artificial intelligence (AI) becomes a dependable instrument to improve the processing of financial documents.
Extracting significant numbers, spotting irregularities, and guaranteeing regulatory compliance are just a few of the many facets involved in processing financial documents. The inherent time-consuming and error-proneness of traditional manual procedures makes them extremely inefficient when compared to the capabilities of artificial intelligence. Artificial Intelligence provides a more streamlined and effective method, freeing up human resources for more significant initiatives in financial organizations.
Generative AI is being used more and more by hedge funds and other money managers to process documents. According to a recent poll, about half of these funds use ChatGPT for work-related reasons. Of them, more than 70% make use of this technology to accomplish things like write marketing content or summarize lengthy documents and reports.
Artificial Intelligence (AI) facilitates innovation and growth in financial organizations by freeing up human resources to concentrate on strategic projects by automating repetitive operations. Moreover, AI models’ scalability guarantees that enterprises can adjust to evolving document structures and requirements, putting them at the forefront of operational excellence.
This thorough tutorial highlights the critical role that AI plays in improving document processing by outlining the procedures for creating AI models for financial document analysis. Along with these topics, it covers industry best practices and the advantages of intelligent document processing.
- The difficulties of managing a lot of financial documents
- 10 Best AI Tools for Accounting 2024
- What is AI-powered financial document processing?
- Enhances customer service, manages emotions, and gathers relevant information
- Applications of AI models in financial document processing
- How can an AI model for processing financial documents be built?
- Best practices for building AI models in financial document processing
- AI models’ advantages in processing financial documents
The difficulties of managing a lot of financial documents
Keeping up with ever-increasing document stacks presents a huge challenge for institutions in the fast-paced world of finance. Financial firms struggle with difficulties that affect productivity, compliance, and decision-making, whether they are working with digital files or paper documents. Even a small error can cost the business and the client a lot of money. Difficulties with document management, like processing delays or inaccuracies, can result in missed investment opportunities or fines from the government for noncompliance with regulations. Let’s examine the major difficulties they encounter:
- Document retrieval complexity: Finding individual files gets harder as there are more documents in existence. When vital information is needed, financial teams find it difficult to locate it, which causes delays in decision-making and lowers overall productivity. Operational efficiency suffers in the absence of well-designed retrieval mechanisms. Establishing consistent processing procedures is difficult due to the variety of document formats and structures, which exacerbates the problem of managing this data overload.
- Extended document process cycle: Before a document is approved, it goes through a number of phases, including processing, review, and approval. Extended cycles reduce response in a timely manner and impede operational efficiency. The financial team faces additional hurdles as a result of outdated Document Management Systems (DMS) that are unable to properly automate and streamline procedures.
- Adherence to regulations: Another level of complication arises from navigating the complicated web of regulatory obligations. Paying close attention to detail is necessary to ensure compliance with constantly changing standards and laws, as noncompliance may have legal repercussions.
- Integration difficulties It takes skill to integrate document management systems with current technologies and processes in a seamless manner. Data silos are caused by compatibility problems, which impede the efficient exchange of information between departments. Comprehensive analysis, compatibility tests, and middleware implementations are necessary for successful integration. To ensure a coherent operating environment, new technologies and legacy systems must work together seamlessly and share data.
- Data security and privacy are important issues since financial data is sensitive. Strong security measures are needed to ensure that sensitive data is safeguarded throughout the document lifecycle. There are constant issues in ensuring safe storage, enforcing access rules, encrypting data, and stopping data breaches. Accessibility and secrecy need to be balanced in fintech organizations.
- Scalability: As document quantities rise at an exponential rate, scalability is becoming an increasingly important issue. In order to manage increased data traffic, sustain performance, and prevent bottlenecks during peak hours, systems need to scale smoothly. Maintaining operational efficiency requires ensuring scalability.
- Variety and velocity of data: Financial data can be found in a variety of formats, including unstructured, semi-structured, and structured, making it difficult to manage its diversity and extract insightful information. The issue is further complicated by the fact that this data is being generated so quickly that processing must happen quickly. Managing a variety of quickly changing financial data requires the use of adaptive tools and real-time processing capabilities to strike a compromise between accuracy and speed.
10 Best AI Tools for Accounting 2024
Sorting through all the possibilities is the most difficult element of picking an AI solution for accounting. It has been reduced to the top 10 tools for 2024.
Using this list, you may evaluate each tool according to its best features, restrictions, cost, and user reviews to help you make the best decision.
1. ClickUp
A cloud-based business management tool called ClickUp Accounting was created to make bookkeeping procedures easier. So that you may concentrate on the bigger picture, ClickUp Brain can handle accounts, produce reports that can be shared, and serve as your virtual personal assistant.
Natural language processing is used by ClickUp Brain, an AI-powered virtual assistant, to assist with a variety of tasks, including project details, financial management, client check-ins, and meeting updates. Numerous AI technologies covering every facet of your accounting system have been developed by us.
Additionally, you can study and create spreadsheets related to money using ClickUp Docs.
For instance, you may manage your invoices, sales records, income, and projected revenue with the ClickUp Accounting Template. Utilize resource tracking and stay on top of accounts receivable and payable (AR/AP) to enhance overall financial performance.
With over 1,000 pre-made connectors available, ClickUp allows you to manage all of your tools in a single, easily-customizable Dashboard.
The best features of ClickUp
- Select from hundreds of templates to assist with project management, financial flow management, budgeting, and more.
- Utilize ClickUp Brain’s AI capabilities to update sales forecasting forecasts, establish audit policies, communicate with clients, and summarize financial planning meetings.
- Select from more than 100 ClickUp Automations to automate repetitive back-office processes.
- ClickUp’s ChatGPT Prompts for Finance can assist you in making well-informed decisions and enhancing the financial health of your company.
- To improve workflow, switch between several views, make personalized dashboards for each team member, and employ project time tracking.
ClickUp restrictions
- With ClickUp’s abundance of features and functionalities, some users can experience a learning curve. Fortunately, we’ve covered this with free video tutorials for practically everything.
- You cannot use ClickUp Brain with the Free Forever plan.
ClickUp rates
- Free Without Ends Forever: $7 a month for each user
- Business: $12 per user per month; Enterprise: Get in touch for a quote.
- ClickUp Brain costs $5 per member every month on all premium Workspace plans.
ClickUp evaluations and ratings
- G2: 8.800+ reviews, 4.7/5
- Capterra: 3.800+ reviews, 4.7/5
2. Vic.ai
Vic.ai is an AI-powered application for processing invoices that features sophisticated machine learning algorithms and high accuracy rates. Without the need for templates or unique rules, it automates nearly every part of billing using strong algorithms that have been trained on millions of bills.
Vic.ai can extract vital information from bills, identify duplication, and automate the approval process after an invoice is posted. By designating which worker needs to go over each stage of the invoice approval procedure, it also helps your team stay on task.
Best features of Vic.ai
- Utilize financial data synchronization through connections with top enterprise resource planning (ERP) applications. Vic.ai can detect and flag duplicate invoices and other accounting errors, reducing human error.
- Value-added tax (VAT) and other taxes should be automatically recognized, coded, and calculated to increase accuracy and tax compliance.
- Using insights and analytics based on the most recent data from your accounting company, make better financial decisions.
Vic.ai’s limits
- There is no obvious pricing information, and some users claim that the tool is pricey for small firms and independent accountants.
- Reviews point out that more choices for sorting and reporting are needed to increase efficiency.
- Vic.ai cost
- To receive a price quote, customers must get in touch with Vic.ai and supply business information.
Reviews and ratings for Vic.ai
- G2: 20+ reviews, 4.8/5
- Capterra: Not at all
3. Bill
Bill is a cloud-based application that streamlines AP and AR procedures. It is intended for companies who wish to expedite the billing and invoicing process, such as accounting firms.
Additionally, users are granted complimentary access to Divvy From Bill, an automated program for managing expenses and credit. Divvy provides tools for managing expenditures and budgets, along with credit lines up to $15 million.
Bill’s best attributes
- Automate bill payment and approval processes using workflows and smart rules.
- With greater visibility, manage expenditure across teams, projects, departments, and vendors.
- Earn cash-back incentives while using Divvy credit lines to make qualifying purchases.
- Easily handle several clients while processing AP invoices, approvals, and payments.
Bill restrictions
- may impose fees for specific services and transactions.
- Not all currencies, payment methods, or credit cards are supported by Bill.
- The bill price
- Cost per user: $40 for Essential users; $55 for Teams; $79 for Corporation users; inquire about pricing for Enterprise users.
Reviews and ratings for bills
- G2: 4.3 out of 600+ ratings
- Capterra: 200+ reviews, 4.2 out of 5.
4. Indy
The AI workflow and administrative tool Indy is intended for self-employed individuals. It enables independent contractors to design contracts, send invoices, make proposals, and—most importantly—get paid.
- Bonus: How to Request Payments from a Client in a Professional Manner
With Indy, you can manage your business from a single, easy-to-use dashboard, save information, negotiate the terms of your contract, and track your time for straightforward payment.
Indianapolis’s best attributes
- Benefit from unrestricted access to basic functions and restricted access to enhanced functionality by subscribing to the free plan.
- Use ChatGPT technology to have the Indy AI writing assistant aid you in creating proposals and contracts.
- Utilize the app’s interface with Google Calendar and Zapier to streamline your workflow (premium plan only)
- To guarantee tax compliance, set up periodic invoices, provide a variety of payment methods, determine tax rates, and monitor each transaction.
limits in Indy
- Three customers maximum for users of the free plan
- A few user evaluations mention errors that occur when time is manually tracked.
Indianapolis prices
- Free Pro: $12 per user each month Indie evaluations and ratings
- G2: Not applicable
- Capterra: 4.7 out of 100 ratings
5. Zeni
Zeni automates planning, spending, and accounting procedures with AI to improve financial operations. It offers real-time financial data analysis to enhance company choices by fusing artificial intelligence (AI) with human expertise to produce the most useful data.
Utilize Zeni to automate the tedious bookkeeping and everyday spending tracking processes.
Zeni’s best attributes
- Use the one-page view to gain a top-down understanding of your financial operation.
- Examine quarterly, annual, and monthly reports to monitor financial development and spot patterns.
- Take the information that needs to be extracted from receipts and send it to a specific email address for record-keeping and aggregation.
- Automatic updates and notifications can help numerous teams communicate and share information more effectively.
Zeni restrictions
- According to certain user reviews, there should be additional instructions on how to evaluate and process data in order to make better decisions.
- Could be costly for certain independent contractors, business owners, and startups
Zeni cost
- Starter: $549 per month, paid yearly at that time
- Growth: $799 payable annually per month
- Enterprise: Get in touch for a quote
Reviews and ratings for Zeni
- G2: 20+ reviews, 4.7/5
- Capterra: Not at all
6. Docyt
The goal of the AI-powered bookkeeping software Docyt is to automate accounting and back-office duties. Real-time reporting can help you get insight and maintain financial control over every facet of your company.
Additionally, Docyt enables you to construct unique vaults for various projects or businesses and store all important financial data and documents in one safe location.
Top features of Docyt
- Various approaches to meet different needs, include computerized bookkeeping for huge enterprises and expense management
- The mobile app offers safe, convenient financial tools and information that is easy to use and browse while on the go.
- Utilize the spending monitoring tool to monitor and manage the cash flow and budget of your company.
- Integration to deliver industry-specific reporting with the majority of the major POS and PMS systems
Docyt restrictions
- Slow response times and trouble contacting customer service are mentioned in a few consumer evaluations.
- A few users have mentioned that further capabilities are needed to help with accounting project management.
Docyt cost
- $50/month for revenue reconciliation and $50/month for expense management
- $50 per month for corporate credit card management
- Mini: $149 per month
- $149/month for insight; $299/month for impact
- Advanced: $499 per month
- Enterprise: $999 per month
- CFO & Accounting Firm: Get in touch for a quote.
Reviews and ratings for Docyt
- G2: Not applicable
- Capterra’s rating is 4.6 out of 30.
7. Gridlex
Gridlex Sky is part of the unified suite of business tools known as Gridlex. Gridlex developed Sky, an accounting, expense, and ERP program, to streamline financial operations.
Manage all accounting, spending, and ERP tasks with Gridlex Sky’s customized automations and AI-powered insights. Invoicing, billing, payroll, general ledger management, and other tasks can be managed by Sky.
The finest features of Gridlex
- Users of Gridlex Sky are also granted access to Gridlex Zip, a CRM and customer care help desk product, as well as HR software Gridlex Ray.
- With automated calculations for profitability, revenue, and expenses, you may do away with the requirement for manual labor and lower the possibility of human error.
- Using an easy-to-use interface, utilize the cost management tool to arrange and maintain receipts and expense claims.
- Reduce administrative processing by automating reimbursements and cost approvals.
Gridlex constraints
- On well-known platforms, the absence of user reviews makes it challenging to evaluate the overall user experience.
- On the first price tier, advanced capabilities like revenue recognition automation are not offered.
- Gridlex cost
- $10/month to begin with; $30/month to grow each user; scale: contact for pricing
Gridlex reviews and ratings
- G2: Not applicable
- Capterra: Not at all
8. Booke
Booke is a solution for bookkeeping automation that streamlines routine accounting duties. It provides real-time data extraction and error reconciliation using AI technology. The AI gets better the more you use it.
With Booke’s user-friendly messaging interface, you can get quicker client responses and cut down on time-consuming back and forth communication.
Best characteristics of Booke
- Use AI to handle coding issues, classify transactions, interact with customers, and streamline your workflow.
- Boost productivity by automating month-end closing duties with AI.
- Identify and resolve issues quickly with Booke’s sophisticated error detection tools.
- Your financial data can be synced with popular apps like Xero and QuickBooks through integration.
- Booke restrictions
- Deficit of user reviews on well-known platforms makes it challenging to base decisions on typical consumer experiences.
Five customers at least for every price range
- Booke’s price
- Clever: $10 per month for each client; $20 per month for premium
- Business: Get in touch for a quote.
- Artificial Intelligence Bookkeeper Robot: Get in touch for a quote.
Reviews and ratings for books
- G2: Not applicable
- Capterra: Not at all
9. Blue Dot
Utilizing proprietary technology, Blue Dot is an AI tax compliance tool that assists businesses in maintaining tax compliance. Reduce tax exposure for consumer-style spending and have a comprehensive understanding of all employee-initiated transactions.
To locate whatever information you require for your company, use the tax knowledge base. You may also use external data by using natural language processing.
Best aspects of Blue Dot
- To determine and compute any qualifying or eligible VAT spending, use VAT Box.
- Allow AI to be used by the Taxable Employee Benefits feature to find and examine wage tax data.
- Streamline your spending management process and allow Blue Dot’s exclusive AI-powered suite enforce tax regulations and checks to keep your company compliant.
- Produce corporate income tax reports with as little human input required to assure compliance as possible.
Blue Dot restrictions
- Because there aren’t many user evaluations on well-known sites, it might be difficult to acquire an overall sense of customer experiences.
- Blue Dot pricing without any visible pricing data
- To obtain quotations and price details, customers need to get in touch with Blue Dot and schedule a demo.
Reviews and ratings from Blue Dot
- G2: Not applicable
- Capterra: Not at all
10. Truewind
Truewind is an AI-powered tool for bookkeeping and finance that helps startups and small enterprises. It offers precise monthly reporting and financial solutions that are specifically designed for your sector.
Additionally, users get access to Truewind’s skilled concierge team to guarantee accuracy and openness.
Best characteristics of Truewind
- Make timely, well-informed business decisions with effective bookkeeping and quicker monthly close times.
- Speak with and collaborate with certified public accountants (CPAs), who can help you with all of your company’s financial procedures.
- Maintain correct back-office processes with little human intervention so that you can concentrate on expanding your business.
- Reduce the complexity of bookkeeping procedures by integrating them with the tools you use to handle finances with ease.
Limitations of Truewind
- Absence of user reviews on well-known review sites
- No pricing or subscription information is displayed
- Truewind costing
- Customers who would need price information must arrange a demo with Truewind.
Reviews and ratings for Truewind
- G2: Not applicable
- Capterra: Not at all
What is AI-powered financial document processing?
AI-powered document processing is a crucial tool in the financial sector that is revolutionizing how companies manage different financial documents and extract insights from them. With this method, information embedded in different financial documents is automatically extracted, interpreted, and processed using artificial intelligence. Improving decision-making processes, accuracy, and efficiency in financial institutions is the aim.
Following the start of the pandemic in March 2020, banks saw a significant increase in forbearance instances, or a greater proportion of clients having trouble making their repayments. This flood caused a significant amount of paperwork to pile up, which in turn made bank employees’ tasks heavier and their level of aggravation higher. Intelligent document processing driven by AI effectively handled this data rush, relieving bank employees of some of their workload.
AI-driven document analysis leverages intelligence and automation to mine data for insightful information. AI document processing is using cutting-edge technologies to automate important processes related to handling a variety of documents. As an illustration,
- Machine Learning (ML): ML algorithms are skilled at identifying document structures, extracting pertinent information, and classifying material because they have been trained on historical data.
- Natural Language Processing (NLP): NLP is the process of extracting meaning from unstructured text, identifying entities (names, dates, quantities), and understanding context. It makes it possible for machines to comprehend and translate human language.
- Optical Character Recognition (OCR): OCR technology transforms handwritten text or scanned images into machine-readable formats. It is a fundamental component of digitizing physical documents. It is essential in converting hard copy papers into digital data that can be used immediately since it can identify characters, numbers, and symbols.
Enhances customer service, manages emotions, and gathers relevant information
The use of AI models in document processing has grown in importance in the financial services industry. These models improve decision-making, expedite processes, and change the way financial organizations manage massive amounts of data. Let’s examine the main features of AI models used in applications for processing financial documents.
AI-driven data extraction
Complex machine learning models are replacing optical character recognition (OCR) methods. Better accuracy is guaranteed by these models’ ability to distinguish characters and contextual cues. They are able to retrieve intricate financial information from a variety of documents, including contracts, invoices, and bank statements. AI-powered data extraction becomes a critical tool as finance organizations want to automate operations.
LLMs for streamlining processes
Large Language Models (LLMs) have becoming more popular, such as ChatGPT. OpenAI claims that these AI systems can comprehend and interact with human language, resulting in up to 56% more efficiency while performing worker tasks. Tasks can be enhanced, automated, and transformed with the use of generative AI in document processing.
Deep learning for improved judgment
AI’s deep learning subset imitates how the human brain processes information. Multiple-layer neural networks process data and get better over time. Deep learning is used in fintech to automate difficult processes including risk assessment, fraud detection, and customer experience analysis. Deep learning models give smart solutions that protect against risks and streamline processes by studying trends.
Applications for processing financial documents are changing as a result of AI models, which increase security, accuracy, and efficiency. In the years to come, we may anticipate more discoveries and game-changing solutions as the sector adopts these innovations.
Applications of AI models in financial document processing
Processing financial documents is essential to several sectors, including accounting, banking, and insurance. Now let’s examine the vital uses of AI models in the processing of financial documents:
Processing of invoices
The Institute of Finance & Management (IOFM) states that there is a wide variation in the expenses related to processing invoices, ranging from $1 to $21. AI model integration has resulted in a revolutionary change in invoice processing. In the past, handling bills from suppliers, clients, or vendors was a labor-intensive, error-prone task that took a lot of time. The introduction of AI has changed important components of the process and brought about increased efficiency and accuracy.
AI algorithms extract relevant data from invoices, including line items, amounts, vendor details, dates, and invoice numbers. These models are able to extract and comprehend the structured data from unstructured text by using natural language processing (NLP) techniques.
The legitimacy of invoices can then be confirmed by AI models by cross-referencing them with purchase orders, delivery receipts, and current information. It is possible to mark any anomalies or discrepancies for additional examination. Furthermore, by matching invoices with matching purchase orders and receipts, AI models may automatically reconcile bills and make sure that the amounts billed and the expected values match.
The ability of AI to detect errors adds a proactive risk management layer. AI quickly highlights frequent problems early in the process, such as duplicate invoices or missing information. This strengthens financial compliance and avoids payment errors.
Workflows driven by AI simplify the entire cycle of processing invoices. AI models can handle repeated jobs, eliminating manual intervention and speeding up the process. Examples of these tasks include receipt scanning and approval workflows.
processing of forms online
In the course of banking and finance operations, which include things like opening, closing, and cash withdrawals, customers are almost always asked to fill out forms. The traditional method of filling out forms on paper has a greater chance of errors and takes a lot of time. Digitalization and a revolutionary solution are introduced when AI is integrated with document processing systems.
Customers can interact with financial services through user-friendly online portals as the entire process smoothly transfers to digital platforms by utilizing AI for intelligent document processing (IDP). The process is made simpler overall by this digital transformation, which turns data into formats that are simple to understand, such as text, tables, and graphs. These formats’ machine-readable quality makes it simple for AI systems to find and process the data, setting the stage for streamlined and effective corporate processes.
This transition from paper-based to online form processing greatly speeds up the workflow overall and improves accuracy by lowering human data entry errors. Customers gain from an easier-to-use interface, and businesses maximize their operational effectiveness—a major development in financial operations.
Management and evaluation of contracts
A complete solution for managing and reviewing contract and agreement documents is provided by AI-driven financial document processing. The improvement of efficiency, accuracy, and compliance in financial processes is made possible by this dual functionality.
AI models assist in extracting important data from agreements and contracts. These models are able to extract important terms and recognize milestones, deliverables, payment schedules, and warranties, termination, confidentiality, and dispute resolution agreements. This thorough data extraction creates the groundwork for a sophisticated analysis of the documents.
Contract and agreement review error margins are decreased by integrating AI models. AI-powered document processing serves as a watchful steward, always checking to see if the terms of agreements and contracts are being followed, pointing out any inconsistencies, and guaranteeing preventative action.
Tracking important components, such as milestones, renewal dates, and compliance requirements, can be made easier by digitizing contracts and agreements. Financial institutions are guaranteed to follow contract requirements pro-actively thanks to automated alerts. Process efficiency is greatly increased by the simplified and automated method provided by AI models. Organizations can use technology to manage mundane but important review procedures while reallocating valuable human resources to strategic priorities.
Information retrieval
Within the processing of financial documents, information extraction is a crucial operation where accuracy in data extraction is essential. The set of features that advanced AI models offer greatly improves the effectiveness and precision of this extraction process. As examples, consider:
Extraction of structured data
AI models utilize methods such as Optical Character Recognition (OCR) to transform documents that are scanned or based on images into text that can be read by machines. Important data, like dates, sums, names, and addresses, can be extracted from initially unstructured text using this approach. In accounting, for example, extracting information from invoices such as line items, due dates, and invoice numbers becomes a streamlined process.
Recognition of Entities
Artificial intelligence (AI) models are particularly good at locating and recognizing individual entities—people, companies, places, or goods—in financial documentation. For a complete analysis and compliance, it is imperative to recognize key entities such as corporate names, stock symbols, and legal entities.
Event retrieval
AI models are capable of accurately identifying events or activities that are specified in documents, like project deadlines, payment terms, and contract milestones. This feature helps companies stay on top of things and efficiently handle their responsibilities.
Labeling semantic roles
Through sentence analysis and action recognition of contract provisions, AI enhances comprehension by identifying subjects, objects, and actions. Understanding the context and relationships between entities is aided by this nuanced approach.
Template-driven extraction
Artificial intelligence ensures consistency and accuracy in data extraction by using specified templates to retrieve specific information. For example, financial ratio extraction from annual reports becomes a dependable and methodical operation.
Cross-document connection
AI makes it easier to connect related data from different documents, such a contract’s revisions or a purchase order’s to an invoice. Cross-document linking promotes better decision-making and improves data integrity.
Fintech companies can also easily adjust AI models to retrieve information particular to their domain. By matching the extraction method with the unique requirements and subtleties of the financial realm, this modification guarantees relevance and accuracy. The financial document processing industry is completely changed by AI-powered information extraction, which helps businesses handle enormous volumes of data effectively, make wise judgments, and stay compliant in the constantly changing financial world.
Classification of financial documents
Sorting through various documents is a crucial activity in financial operations that takes time and accuracy. When this procedure is automated, AI models become a revolutionary tool that elevates accuracy and efficiency.
Financial documents come in many different forms: purchase orders, bank statements, contracts, tax forms, invoices, and more. AI models do exceptionally well in identifying these documents according to their content, layout, and purpose, offering a complete solution for managing a wide range of paperwork.
The core of this method consists of supervised machine learning models that are equipped with labeled examples of various document categories. To efficiently identify and categorize, the model extracts information from documents such as keywords, patterns, and structural aspects. AI models, for instance, recognize pertinent characteristics in papers and adjust their comprehension to fit particular categories:
Features for invoices could include total amounts, vendor names, and invoice numbers.
- Contracts: important components that direct the classification process are clauses, signatures, and effective dates.
The model assigns a probability score to each document category using widely used algorithms such as neural networks, decision trees, and support vector machines. Precise classification is based on the anticipated class, which is the highest-scoring category.
A powerful solution that simplifies AI-powered document management, improves accuracy, and guarantees the quick retrieval of vital financial data is AI-driven financial document classification.
Search and synthesis of financial documents
One particularly useful tool in the search and synthesis of financial documents is artificial intelligence (AI). In addition to improving document retrieval, this revolutionary technology is essential for cross-document synthesis, semantic comprehension, and customized search criteria.
retrieval of documents
AI-driven search engines make it easier to find documents in large libraries. Users may quickly look up particular terms, dates, or entities in a variety of financial records. They can expedite information access by locating tax forms from a specific year or retrieving all contracts associated with a particular provider.
Semantic comprehension
AI models demonstrate a sophisticated semantic comprehension of search terms. They produce pertinent results by taking synonyms, similar phrases, and variants into account. A search for “interest rates,” for example, turns up documents stating “loan rates,” demonstrating the technology’s sophisticated understanding.
Synthesis of cross-documents
Artificial Intelligence surpasses mere document retrieval by amalgamating data from several sources. It summarizes, emphasizes important details, and finds recurring themes. For analysts who are looking for insights without having to perform the laborious task of reviewing each document separately, this cross-document synthesis is quite helpful.
Personalization
The flexibility to alter search parameters to suit individual requirements is advantageous to users. AI adjusts to user choices and gradually increases search accuracy. The user experience is improved by the customisation that this customization brings.
In conclusion, AI-driven document synthesis and search are strong instruments that enable businesses to effectively manage risks, make well-informed decisions, and preserve financial transparency.
Financial reporting that is automated
AI-powered automated financial reporting is revolutionizing how organizations handle data and make decisions. In the data journey, artificial intelligence (AI) has a central role, combining data from many sources, such as transaction records and accounting software.
Then, when AI populates templates with pertinent financial data and lets users select particular metrics and visuals, personalizing reports becomes simple. AI is a conscientious financial analyst; it computes, examines patterns, and identifies important metrics.
AI-generated visuals, such as graphs, charts, and tables, make complex financial data easier to understand. Scheduling report generation at desired intervals and making it simple to distribute them to stakeholders is another aspect of automation. AI provides predictive insights that go beyond historical analysis and support strategic choices based on anticipated financial patterns.
Automated financial reporting powered by AI simplifies procedures, minimizes manual labor, and delivers timely, data-driven insights, enabling businesses to make wise decisions.
Client orientation
The introduction of AI technologies brings about a significant transformation in customer onboarding, a critical stage for financial organizations. This smooth onboarding process for new customers includes multiple aspects that contribute to the overall experience.
Creditworthiness is evaluated by AI using transaction patterns, credit ratings, and historical data. Automated credit checks speed up account access and loan approvals. AI models also evaluate investing preferences and risk tolerance. Personalized investment suggestions are based on this data.
By automating the creation of accounts, AI reduces paperwork and human data entry. Clients can use mobile apps or the internet to open accounts. Chatbots customize conversations according on user profiles. Chatbots assist users with tasks, respond to inquiries, and offer account information. Customer input can be analyzed by AI algorithms to find problems and potential areas for improvement.
Artificial intelligence (AI) facilitates client onboarding, improves security, and provides individualized experiences, laying the groundwork for enduring partnerships.
How can an AI model for processing financial documents be built?
We covered the function of AI and a wider range of AI applications in financial document processing in the earlier sections. The process of creating an AI model for processing financial documents is walked through in this section.
formulation of problems
Building an AI model for processing financial documents requires a thorough understanding of the business requirements and the formulation of the problem at hand. Important elements are:
- Describe the particular issue: Clearly state the issue that you are trying to resolve. Is it precise contract analysis, information extraction from invoices, or customer onboarding with predetermined parameters? For each of these, a separate strategy could be needed.
- Limitations and extent: Recognize the limitations of your issue. Do you handle a wider variety of documents, or are you concentrating on a particular kind (such as purchase orders, invoices)?
- Metrics for success: Establish success criteria in line with corporate objectives. Establish metrics to gauge the performance of your financial document processing model, such as accuracy, recall, or processing speed.
- Business goals and requirements: Seek to comprehend current issues from a domain-specific perspective. Several important things to think about are:
- Interviews with stakeholders: Communicate with stakeholders to learn about their problems, such as accountants, compliance officers, or business analysts. Which current workflow inefficiencies are present in document processing?
- Impact on business: Calculate the effects of document processing automation. Will it lower expenses, increase customer satisfaction, or speed up and improve accuracy of processes?
- Requirements for compliance and law: Take into account regulatory requirements (such as GDPR and HIPAA) and compliance guidelines that apply to financial data.
Gathering and preparing data
- Data collection: Start by gathering a variety of financial records. These consist of purchase orders, bank statements, tax papers, and invoices. Your model’s generalization will be better with a more diverse sample.
- Data purification: Utilize established formats, deal with missing values, and eliminate noise. Scanner pictures can be converted into machine-readable text using OCR software.
- Data augmentation: If your dataset is little, think about expanding it to replicate real-world situations by adding noise, rotating, or cropping it.
Annotation and labeling
Conventional labeling may not be necessary for generative models. Training data for generative AI applications may include samples of the kind of content you would like the model to produce.
- Labels for ground truth: Label important details (such the invoice date, total amount, and vendor name) on each document by hand. Your ground truth for supervised learning is this labeled data.
- Tools for annotations: To make labeling easier, make use of platforms or tools for annotation.
Extraction of features
Feature extraction is the following stage, which includes:
- Text features extraction: Take pertinent text out of the papers. Textual material can be represented using methods like Word2Vec or TF-IDF (Term Frequency-Inverse Document Frequency).
- Arrangement and spatial aspects: Take note of spatial linkages (such as the closeness of pertinent fields) and layout details (such as the placement of text blocks and font sizes).
- Domain expertise: Utilize subject expertise to produce useful features.
- Special characteristics: Recognize the special characteristics of financial papers, such as statements, receipts, and invoices.
Model choice
When creating an AI model for the examination of financial documents, model selection is a crucial stage.
Select the proper machine learning algorithms (such as regression, extraction, or classification) for the given problem.
Think about fine-tuning pre-trained models (like Tesseract and BERT) for financial data.
Select or create a generative model architecture that is appropriate for producing text. Transformer-based models, Long Short-Term Memory (LSTM) networks, or Recurrent Neural Networks (RNNs) might be useful. Choose an architecture that meets your unique requirements based on the type of data you have and the type of financial documents you have:
- neural networks with recurrence (RNNs)
- Perfect for text in financial records or other sequential data.
- Ideal for recording patterns and dependencies in a sequential manner.
- Neural networks with convolutions (CNNs)
- efficient at removing characteristics from financial records that use images.
- very helpful for handling scanned invoices or receipts.
- Transformer-oriented models, such as BERT
- Textual content can be understood contextually thanks to pretrained language models.
- Ideal for assignments that call for understanding intricate document structures.
Other important factors to take into account throughout the model choosing process are:
- Use transfer learning to your advantage by refining a pre-trained model—like BERT—using your unique financial data. This method makes use of the knowledge that is already present in the trained model.
- If available, take advantage of domain-specific pre-trained models for transfer learning. Model performance is improved and alignment of financial text data with your use case is facilitated by fine-tuning models.
- Iterative model refinement: The process of choosing a model is iterative. Review this step frequently, particularly after assessing the model’s performance. Make continuous improvements by iteratively honing your architectural selection and incorporating industry innovations.
Model creation and assessment
You can adjust hyperparameters particular to the selected architecture after you choose your model architecture based on needs. For best model performance on financial documents, tweak variables like as regularization terms, batch sizes, and learning rates.
- Develop and polish the model: Labeled data can be used to train and improve your model. To maximize performance, tweak the settings and hyperparameters.
- Analyze the performance of the model: Use important metrics to evaluate the success of your model, such as precision, recall, and F1-score. Coherence, fluency, and relevance of generated content are evaluation measures for generative models. As you see fit, refine your training methodology and model to ensure ongoing progress.
- Iterative creation: Take part in an iterative process of development. If necessary, go back and modify the model’s architecture, training methods, or data preprocessing in light of the evaluation’s findings. This iterative procedure makes sure that your financial document processing model is continuously improved and refined. Iterative improvements are made to generative AI models for document processing through qualitative evaluations and user input.
- group methods Investigate ensemble approaches that integrate predictions from several architectures. Ensemble models have the potential to improve generalization and resilience, particularly when handling a variety of document kinds.
- Taking future scalability into account: Take your solution’s scalability into consideration while choosing an architecture. Select models that maintain efficiency even in the face of future changes in document formats or an increase in the volume of documents.
Implementation and upkeep
When deploying the AI model in a production setting, keep the following things in mind:
- Scalable infrastructure: To enable the model’s deployment in a production setting, select an infrastructure that is scalable.
- Integration: Make sure there is a smooth transition between the production environment’s current procedures and systems.
- Instruction for users: Provide end users or the operational teams using the deployed model with training.
performance tracking for required updates
- Real-time tracking: Set up real-time tracking systems to keep tabs on the accuracy and reaction times of the model.
- Automated alerts: Set up automated alert systems to inform the appropriate staff members of abnormalities or performance declines.
- Constant improvement: Make plans for ongoing model updates based on fresh information and understandings.
- The way that generative models produce content changes with time, thus they are modified accordingly.
- Transparency is necessary while making financial decisions. When feasible, use interpretable models (such as decision trees and linear regression).
- Apply strategies (such LIME and SHAP values) to clarify model predictions.
Make plans for future iterations and scalability.
- Scalability measures: Take into account steps to guarantee scalability when the amount of papers handled or the intricacy of the financial data rises.
- Feedback loops: Put feedback loops in place for ongoing learning and model improvement in response to customer input and changing business requirements.
- Version control: Keep the deployed model’s version under control to enable the smooth introduction of new iterations while guaranteeing traceability.
Best practices for building AI models in financial document processing
A deliberate strategy is necessary to create machine learning models that work in financial document processing. The following crucial best practices will direct the creation of AI models for processing financial documents:
- Cooperation with financial specialists: To benefit from their domain expertise, collaborate closely with financial specialists. Their understanding improves model accuracy and relevance by bridging the gap between data patterns and complex financial situations in the actual world.
- Make use of distinct document formats: Create models with an acute awareness of the many formats used in financial documents. Whether working with bank notes, letters of credit, or 10-K reports, accurately extracting and analyzing information is ensured by adapting to these distinct forms.
- Composable, modular pipelines: Choose composable, modular pipelines over monolithic models. Make representations of Directed Acyclic Graphs (DAGs) that coordinate individual processing blocks. This modular strategy promotes effective dependency management, hierarchical data flow, and teamwork.
- Reusing current models as flimsy indicators Add knowledge from current models to the pipeline. Models may become antiquated, yet for some subsets of data, their insightful conclusions hold true. Reusing pre-existing models improves the overall performance of the model as a starting point.
- Combine technical know-how with intuition: Developing successful AI models for financial document processing necessitates combining technological expertise with a thorough understanding of financial difficulties. This all-encompassing strategy guarantees solutions that satisfy technical requirements and correspond with the particular needs of the financial sector.
Developers may provide technically sound models that are precisely tailored to the complexities of the financial landscape by following these best practices, which help them traverse the complexities of financial document processing.
AI models’ advantages in processing financial documents
A number of benefits come from integrating AI models with financial document processing, which transforms how businesses handle data. The following important advantages highlight the revolutionary influence of AI in this field:
- Scaled consistency and accuracy: AI models are excellent at classifying and extracting data accurately, removing human error and guaranteeing a uniform methodology across financial records. Generative models facilitate accurate data extraction and contextual understanding within financial documents by understanding and producing text that is similar to that of a human.
- Rapid and efficient document processing: Automated AI algorithms greatly increase speed by accelerating document processing. Generative models improve the overall efficiency of financial document workflows by enabling quick document comprehension and processing.
- Scalability to handle increasing volumes: Artificial intelligence (AI) systems are scalable, effectively handling big datasets without sacrificing speed. This scalability is essential for businesses handling growing amounts of financial documentation.
- Effectiveness in information retrieval: Artificial Intelligence (AI) reduces human labor while simultaneously improving data accuracy by automating the extraction of pertinent information from financial documents.
- Customization and flexibility: AI models exhibit adaptability to a variety of document kinds and structures, and they can be tailored to certain financial domains or organizational requirements. It is possible to fine-tune generative models for certain financial domains, adjusting their language production to organizational requirements and accommodating different document structures.
- Automation that saves money: AI-powered automated document processing results in lower operating expenses. Organizations also gain from the insightful data that AI produces, which facilitates well-informed decision-making. Generative models save operational costs and offer nuanced insights for better decision-making by automating document summarizing and content development.
Conclusion
We hope that this guide has helped you understand AI For Financial Document Processing In 2024. Additionally, Appic Softwares is the top Finance app development company that you should check out.
What Are You Waiting For, Then?