AI In Credit Scoring: A Complete Financial Risk Overview
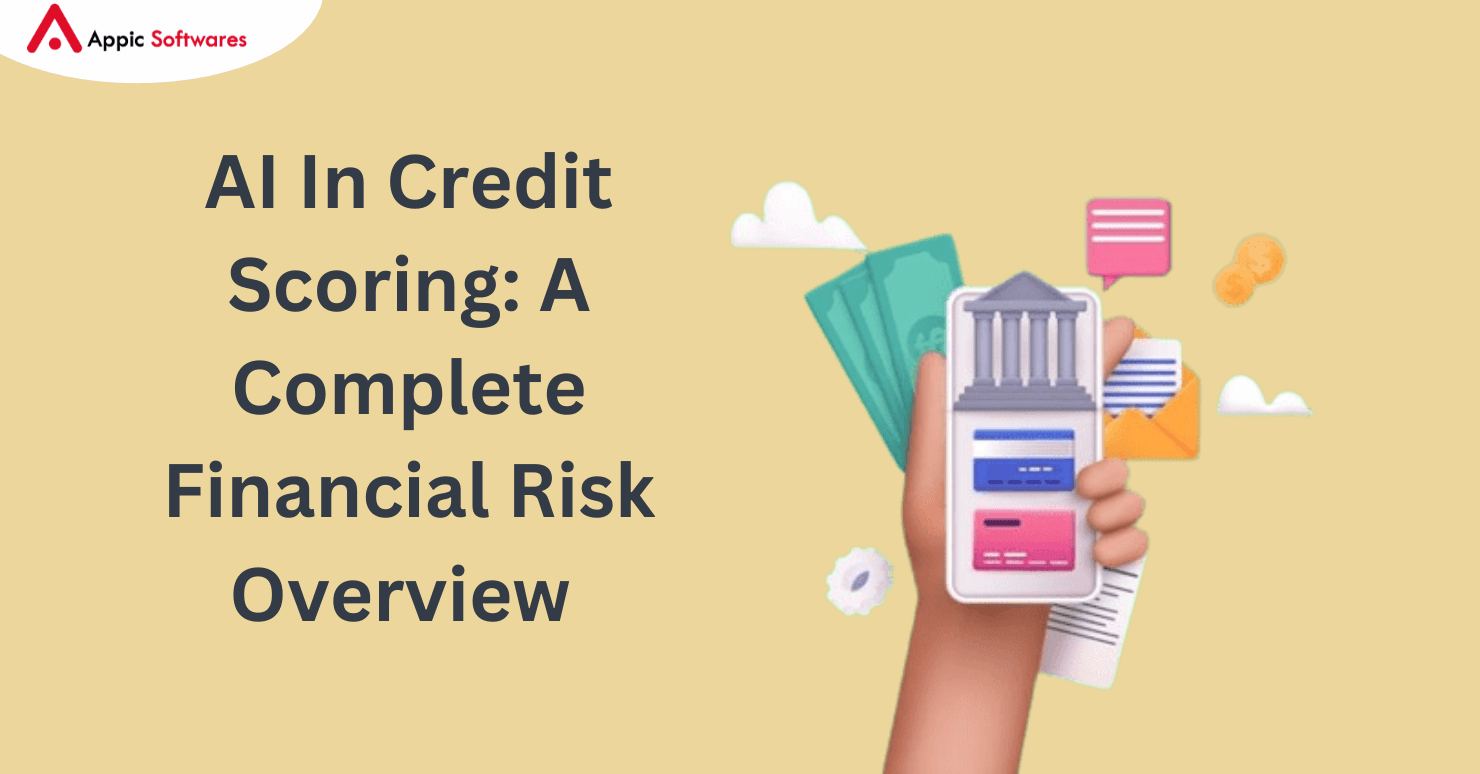
In the intricate world of finance, having access to liquidity is crucial. It’s important for individual life milestones like buying a house, a car, and an education. It’s also important for entrepreneurship, as it influences the start-up, development, and growth of businesses. Lending and borrowing transactions are rife with uncertainty, which has a substantial impact on prospective debtors as well as creditors. In order to determine if a borrower would be able to repay a loan, creditors often need a lot of information. In contrast, prospective debtors find it challenging to raise their creditworthiness since they must traverse a rather opaque procedure with unclear approval requirements.
In this context, AI-based credit rating shows itself as a game-changing approach. It assesses a borrower’s creditworthiness holistically, allowing creditors to make better loan choices. Artificial Intelligence (AI) integrates several data sources, including internet transactions and behavioral patterns, in contrast to conventional approaches. With this method, AI algorithms are able to uncover correlations and patterns that would otherwise go unnoticed, providing a more accurate and detailed picture of an applicant’s creditworthiness. The worldwide market for credit scoring services is anticipated to increase at a compound yearly growth rate of 24.06% to reach USD 18.97 billion in 2022, according to Polaris Market Research.
The purpose of this essay is to examine the complexities of artificial intelligence (AI)-based credit scoring. It will cover its mechanisms, advantages, uses, and practical applications.
What is credit scoring and its key metrics?
The intricate process of credit scoring entails evaluating a person’s or company’s creditworthiness to ascertain their capacity to repay a loan or credit. Numerous quantitative and qualitative elements are considered in this evaluation. Let’s examine these variables and their effects on credit rating in more detail. Important elements consist of:
Factors that are quantitative
- Income: One important consideration is the borrower’s income. Lenders want to make sure that borrowers make enough money, consistently, to cover their debts. Lower credit risk is often associated with higher income levels.
- Monthly expenditures: It is possible to calculate how much discretionary income the borrower has for loan repayments by looking at their monthly expenses, which include rent or mortgage payments, utilities, insurance, and other regular charges.
- Cash flow analysis: This is an essential process for firms. The assessment of a business’s capacity to produce steady income and fulfill financial commitments is based on an analysis of its cash flows.
Qualitative elements
- Marital status: Given its potential to affect a person’s financial stability, it might be seen as a significant aspect. For example, compared to single-income homes, dual-income households could have more stability and financial resources.
- Education: A borrower’s educational background may be taken into account by some lenders. Though this criterion is less often utilized, better education may be correlated with increased earning capacity and financial responsibility.
- Type of work: Lenders evaluate a borrower’s job steadiness. Long-term or permanent work is often seen more favorably than part-time or erratic employment.
- Legal form (for enterprises): A borrower’s creditworthiness may be impacted by their choice of legal structure (such as corporation, LLC, or sole proprietorship). Every legal structure has effects on financial stability and liabilities.
- Industry: The credit risk of business borrowers may be affected by their industry. Lenders may be less reluctant to provide loans to some sectors than others because of their relative stability and resilience to recession.
- Accounting procedures: Lenders may take into account a company’s record-keeping procedures. Lender trust may be bolstered by using proper accounting procedures and clear financial reporting.
It is important to remember that different lenders and credit scoring algorithms may use different particular variables and weight them differently. Depending on their lending policy and risk tolerance, various lenders may give differing weight to certain considerations.
Negative customer actions that affect credit score are as important. Among them are:
History of payments
One of the most important aspects of credit score is payment history. It displays a history of paying loans, bills, and credit accounts on schedule. The credit score will be favorably impacted by consistently making on-time payments and maintaining a spotless record free of public records of litigation, liens, or bankruptcies. On the other side, late payments are penalized, and the more past due a payment is, the worse it will be for your credit rating.
Use of credit
Credit utilization, which is calculated by comparing your credit card balances and limitations, indicates the percentage of your available credit that is presently being used. It’s widely accepted that keeping your credit usage ratio lower—ideally around 30%—will improve your credit score.
Credit history
The duration of your credit account history is referred to as your credit history. Longer credit histories are seen positively by credit scoring algorithms since they provide a more comprehensive background of your credit management practices.
Utilizing credit
The terms “credit use” and “credit management” relate to the several forms of credit. A variety of credit types, such as credit cards, mortgages, rent, vehicle loans, and utility bills, indicate a varied credit history. When you utilize credit responsibly and consistently, along with timely payments and good account management, you may build a good credit history.
fresh credit
In credit scoring models, the “new credit” component takes into consideration the creation of new credit accounts and recent credit inquiries. The process of applying for credit is what matters most in this situation. It’s usually okay to apply for credit cards, mortgages, and vehicle loans. However, applying for more than one credit card at once or for an auto loan and credit card at the same time may cause people to question if you plan to utilize one kind of credit to pay off other obligations.
What is AI-based credit scoring?
A modern technique to assess a borrower’s creditworthiness is AI-based credit scoring. Unlike the traditional method, which is dependent on fixed characteristics and past data, AI-based credit scoring makes use of machine learning algorithms to examine a wide range of data from several sources. The goal of this sophisticated method is to predict the likelihood that a borrower would return a loan. Consequently, AI-powered credit scoring gives lenders an accurate and complete picture of a borrower’s financial behavior by offering a thorough evaluation of credit risk.
Credit scoring models are used by credit bureaus and lenders to evaluate an individual’s or business’s creditworthiness and predict the probability of default on loan commitments. These models take into consideration a number of variables, including credit usage, credit history, payment history, account types, and recent queries. A formula based on this assessment is used to construct a credit score, to which each component is allocated a weight.
Higher scores indicate a reduced default risk. Credit scores often range from 300 to 850. These ratings are used by lenders to set lending conditions, such as interest rates, loan amounts, and repayment schedules. Higher credit scores may lead to more beneficial loan conditions; conversely, lower credit scores might result in less favorable terms, such as tighter repayment obligations and higher interest rates.
How does AI-based credit scoring work?
Machine learning algorithms are used by AI-based credit scoring models to determine a person’s creditworthiness. Extensive historical datasets that provide details on the financial habits and loan repayment outcomes of borrowers are used to train these algorithms. Machine learning algorithms find patterns and correlations that indicate a borrower’s capacity or propensity to repay a loan by examining this previous data.
Here’s a more thorough explanation of how credit scoring using AI functions:
- Information gathering: AI-powered credit rating algorithms collect information from a range of sources. A fundamental data source includes traditional credit information, such as payment history, current obligations, and credit history length. But the capacity of AI-based models to include other data sources—which provide a more complete picture of a borrower’s financial behavior—sets them apart. These substitute resources may consist of:
- Transaction data: Details on a person’s financial dealings, such as earnings, outlays, and spending patterns.
- Internet surfing behavior: Information on internet searches, website visits, and purchasing patterns.
- Social media activity: Examining postings, relationships, and exchanges on social media to get insight into the habits and social circle of an individual.
- Data processing and feature engineering: Following collection, the data is processed and features are created. Choosing pertinent features (variables) for the machine learning model to employ in its prediction process is known as feature engineering. This is an essential stage in developing prediction models that work.
- Model training: Preprocessed and designed datasets are used by ML algorithms to train them. The model gains the ability to identify intricate links and patterns in the data during training. It determines which characteristics and their interactions have the most influence on creditworthiness prediction.
- Prediction: The model can make predictions on fresh, untrained data after training. The model evaluates the borrower’s credit risk and forecasts the chance of repayment based on the information provided in the loan application. The patterns and connections it has discovered from the past data serve as the foundation for this forecast.
- Comprehensive evaluation: By examining a wide variety of data sources, AI-based credit scoring algorithms provide a more thorough evaluation of credit risk. Conventional credit scoring techniques depend on past credit information, which could not accurately reflect an individual’s financial habits. On the other hand, AI-based models take into account both conventional and unconventional data sources, providing a comprehensive picture of a person’s creditworthiness and financial behavior.
But in order to guarantee ethical and correct conclusions when using AI-based credit scoring, fairness, openness, and data privacy issues must be addressed.
How can AI in credit scoring enhance risk assessment?
The standard scorecard method created at the process’s commencement is still used in many financial organizations for credit scoring. A potential borrower has to have a significant history of prior borrowing activity in order to be deemed “scorable.” Even if they are creditworthy, new clients in the banking industry sometimes struggle to get credit because they lack past information.
In comparison, evaluating a prospective borrower’s creditworthiness using AI-powered credit scoring is more dynamic and real-time. It takes into account their prospective earning ability, job prospects, and existing income level. This implies that although borrowers who may pass standard credit scoring tests but engage in hazardous conduct (such as frequent credit card churning) may not be accepted into credit programs, those with high potential are more likely to be accepted.
Financial organizations may make better lending choices thanks to AI-based credit scoring, which uses intelligent AI models that take into account a wider variety of real-time indications to provide forecasts that are more accurate.
Types of credit scoring models
The two main categories of credit scoring models are statistical and judgmental, and each has a unique method for determining a person’s creditworthiness.
- Statistical scoring models: By examining a variety of variables obtained from credit reporting agencies, statistical scoring models use a data-driven methodology. Payment patterns, credit use, length of credit history, kinds of credit accounts, and recent credit inquiries are a few examples of these variables. After correlating and analyzing these variables, the model determines weights depending on how each affects creditworthiness. The credit official’s experiences or subjective opinions have no bearing on the scoring procedure, which is entirely objective. Based on a statistical study of the person’s financial activity, the resultant credit score is a numerical representation of their credit risk.
- Models of judgmental scoring: Models of judgmental scoring use a more subjective methodology, taking into account both subjective evaluations and objective financial facts. These models include a financial statement for the person or company, payment history, bank references, and the subjective assessment of human underwriters throughout the decision-making process. A more individualized assessment is made possible by this scoring approach, which takes into account both financial information and the specifics of an applicant’s credit history.
While judgmental scoring models use individual evaluations and experiences to determine credit ratings, statistical scoring methods depend on a statistical examination of measurable data. Each model has advantages of its own and is used according to the particular requirements and preferences of the lending institution or organization.
Traditional vs. advanced credit scoring models
Most credit scoring models were built on the basis of historical payment data and were created using statistical analytic techniques such as logit modeling, decision trees, and linear regression. To evaluate credit risk, these conventional models used a little amount of structured data.
- Linear regression: The goal of regression-based credit scoring models is to forecast and elucidate default risk and credit risk. This is accomplished by the analysis of structured data with an emphasis on the desired result (default/non-default, for example). Numerous independent variables or aspects pertaining to a person’s credit history, financial situation, and other pertinent information are included in the structured data.
- The procedure entails determining the parameters that minimize the discrepancies between the observed credit risk and the expected credit risk (derived from these independent variables). Regression analysis, a statistical method that determines the connections between the independent variables and the desired result, is used to do this.
- The goal of the regression model is to express this connection mathematically as an equation. For example, the likelihood of a person defaulting rises with their level of debt. Based on the available data, the parameters are tuned to provide the most accurate credit risk estimate.
- Through the use of this technique, lenders and other financial organizations may learn important information about the variables affecting credit risk. This knowledge helps them analyze possible risks related to the loan and guarantees that they make well-informed judgments about granting credit to an application and under what conditions.
- Discriminant analysis: Discriminant models are unbiased techniques for identifying the distinctions between loyal and unfaithful clients. Lending companies may distinguish between clients with strong credit and those with poor credit by using discriminant analysis. Lenders often search for ways to use information from the client’s financial accounts to detect problematic borrowers. Thus, using basic discriminant analysis helps a lot in giving the lenders a trustworthy answer.
- A composite score is given to every consumer. After that, lenders might establish a minimal score to separate their excellent clients from their bad ones. The advanced discriminant analysis technique takes into account a wide range of characteristics that might affect credit ratings, in contrast to the simpler model that only takes into account two. These elements interact, and the model gives each element the proper weight to provide a more thorough credit rating system.
These days, two widely used credit scoring models are:
- Deep neural networks: Rather than depending on pre-established equations, these models learn to identify patterns in data by repeatedly processing information over many layers. By integrating outputs from earlier layers, they may adjust and improve their comprehension, which makes it possible to identify intricate, nonlinear patterns in unstructured data.
- Clustering: The credit scoring method divides data into discrete groups that show significant variations. Under these circumstances, a clustering algorithm may produce a distinct cluster for borrowers whose creditworthiness is difficult to determine with accuracy. Once this cluster has been located, the average assessment or default rate within it may be used as a benchmark to determine the likelihood of default for specific borrowers in the same cluster. It is a useful tool for lenders evaluating creditworthiness since it uses similarities within clusters to produce more accurate credit risk evaluations for borrowers with comparable features.
While newer AI-based models employ a variety of data sources and sophisticated algorithms to give more accurate and inclusive credit evaluations, traditional credit scoring methods largely use structured data and statistical methodologies. By processing unstructured data and spotting intricate patterns, these AI models—in particular, deep neural networks and clustering—have transformed credit scoring systems and, in the process, increased credit accessibility for a wider spectrum of people and enterprises.
Use cases of AI-based credit scoring
Credit scoring models are effective instruments that lenders use to assess a borrower’s or business’s financial standing. These models forecast the probability that borrowers will make timely loan repayments by using a variety of variables and data. AI-based credit scoring models have many and significant applications in a variety of industries, including:
Finding interest rates on loans
Artificial intelligence (AI)-based credit scoring models use machine learning algorithms and a wide range of data sources to improve the accuracy of loan interest rate calculations. In comparison to conventional techniques, these algorithms use a more dynamic and thorough evaluation by carefully examining a borrower’s payment patterns, credit history, and other relevant criteria.
The AI-driven method uses complex models to filter and examine large amounts of data in order to find patterns and insights that may not be obvious at first glance. The evaluation of a borrower’s creditworthiness thus gets more precise and detailed.
This implies that interest rates on loans are customized for borrowers based on a thorough credit risk analysis powered by AI. Due to a decreased perceived risk of default, those with solid credit histories and prudent financial habits—as determined by the AI—are probably going to profit from reduced interest rates. Conversely, in order to reduce the lender’s risk, borrowers who are deemed to have a greater credit risk by the AI model may face higher interest rates, which would incentivize them to continue practicing prudent financial management.
Determining credit limits and credit card approvals
AI-based credit scoring algorithms are being used by credit card issuers more often to assess the applicants’ financial stability. These models go beyond typical credit scores to include an applicant’s income, debt, and other pertinent financial habits, in addition to their credit history. Credit limits, interest rates, and credit card conditions are determined in large part by the results of these AI-driven models, which guarantee that the choices are in line with the risk profile of the applicant.
AI-based credit scoring uses machine learning algorithms to do an extensive and dynamic examination in order to determine credit limits. Examining a person’s payment history, transaction patterns, and other relevant financial data are all part of this procedure. Higher credit limits may be granted to applicants with better credit scores as a consequence of AI’s thorough analysis, which is supported by a larger dataset and reflects a sophisticated assessment of their lower-risk characteristics. Conversely, AI’s evaluation may recommend more limited credit limits for those with lower credit ratings, in keeping with a deemed greater default risk.
A more comprehensive and data-rich evaluation is made possible by this AI-enhanced method, enabling lending limitations that are more closely matched to a person’s actual creditworthiness and financial capability. This procedure helps issuers provide adequate credit card alternatives to a wide range of customers while successfully controlling risk.
Approvals of business loans
When assessing companies that apply for loans, artificial intelligence (AI) has a revolutionary effect on existing credit rating techniques. AI carries out complex analysis of large datasets using machine learning algorithms, gleaning insights from non-traditional and dynamic aspects to evaluate a company’s creditworthiness more comprehensively. It enables enhanced prediction accuracy, adaptive learning, and real-time evaluation, giving lenders more information with which to decide whether to approve loans and what conditions to set for them.
AI in figuring out insurance costs
AI-based credit scoring models are used in the insurance industry to evaluate an individual’s or entity’s financial responsibility beyond only their credit history. Artificial Intelligence (AI) may assist insurance businesses, such as house insurance providers, establish rates that are more in line with the individual’s real risk level by evaluating large amounts of data and providing a more nuanced forecast of payment dependability and overall risk.
AI-powered risk management and portfolio analysis
Advanced analytics are incorporated into risk management and portfolio analysis using AI-based credit rating algorithms. They let financial organizations to analyze risk exposures in their loan portfolios more quickly and accurately by processing massive volumes of data at rapid rates. More proactive and strategic decisions about capital allocation, risk reduction, and diversification are made possible by AI’s predictive powers.
Artificial Intelligence in Financial Counseling and Planning
Financial advisers may provide more proactive and individualized guidance by using AI-based credit rating algorithms. With the use of AI, customers’ financial patterns and habits may be better understood, allowing for more accurate advice on raising credit ratings and maintaining overall financial health. The effectiveness of financial planning and counseling may be increased by advisers using AI to provide methods that are more suited to each client’s particular financial condition and objectives.
In what ways can the generative AI technology from Appic Softwares improve credit scoring workflows?
ZBrain, a generative AI platform by Appic Softwares, revolutionizes credit scoring procedures by enabling companies to improve risk assessment, expedite credit approval workflows, and simplify lending procedures. ZBrain is an all-inclusive platform that is prepared for corporate use, enabling companies to create and deploy applications that are customized to meet their unique needs. The platform trains sophisticated LLMs like as GPT-4, Vicuna, Llama 2, or GPT-NeoX to create contextually aware applications that can do various activities by using the data of its clients, which may be text, photos, or documents.
Credit scoring is plagued by issues including imprecise risk assessment, drawn-out approval procedures, and difficult decision-making. Through its unique feature dubbed “Flow,” which offers an easy-to-use interface that enables users to develop complex business logic for their applications without the need for coding, ZBrain successfully tackles these issues. Large language models, prompt templates, and media models may be seamlessly integrated into the logic of your app using Flow’s user-friendly drag-and-drop interface, making it simple to conceptualize, create, and modify.
ZBrain’s powerful solutions streamline credit-scoring procedures by transforming complex data into useful insights. This leads to increased accuracy, faster processing times, and better credit risk management overall. ZBrain’s all-inclusive solutions successfully address important issues with credit scoring procedures. Examine the next section to see how ZBrain improves credit scoring for the banking and financial industries:
AI-powered credit scoring in banking and finance
ZBrain uses an automated method to change the way that banks and the financial industry score credit. It collects financial information with ease, including bank statements, credit reports, tax returns, job verification, and public records. ZBrain uses exploratory data analysis (EDA) to make sure that data is consistent and that complex data structures are understood. ZBrain converts textual input into numerical representations by using embedding techniques, and it is capable of capturing semantic subtleties for efficient retrieval. ZBrain receives a question, gathers relevant data, and sends it to an OpenAI LLM for comprehensive analysis. ZBrain uses cutting edge AI algorithms to provide a credit score that accurately measures creditworthiness. It does this by taking into account a variety of data points and current financial indicators while the LLM dynamically creates a cohesive report. ZBrain carefully parses and organizes the output after report production, removing any unnecessary material and following the required parts, format, and report criteria. Credit scoring is more accurate and efficient because to this painstaking procedure.
ZBrain’s LLM applications significantly reduce the time and effort often required for human credit score evaluations, enabling financial institutions to make data-driven lending decisions quickly. This preserves stringent data privacy rules while producing more effective credit approval procedures, less risk exposure, and an overall improvement in the lending process. Check out this in-depth Flow to see how ZBrain is redefining credit scoring in the banking industry by offering a more precise and efficient way to determine creditworthiness and speed the loan approval process.
Benefits of AI-based credit scoring
Numerous advantages of AI-powered credit scoring may greatly increase the effectiveness and precision of determining a person’s or company’s creditworthiness. Here’s a thorough analysis of the benefits of using AI to credit scoring:
- Increased accuracy: AI models are able to examine enormous volumes of data from several sources, which leads to a more thorough and precise assessment of credit risk. AI can detect subtle patterns and connections that people would overlook by taking a broader variety of characteristics into account, which lowers the possibility of false positives—rejecting qualified candidates—and false negatives—approving dangerous applications.
- Faster decision-making: Compared to manual methods, AI systems digest information much more quickly. Both loan applicants and lenders may save time and effort by having their applications examined and judgments made in a matter of seconds. This speed is particularly crucial in peer-to-peer and online lending, where making judgments quickly is necessary to stay competitive.
- Risk segmentation: By classifying customers into more accurate risk groups, AI enables lenders to provide customized loan terms and interest rates. This helps both borrowers and lenders by connecting people or companies with the best possible financial offers.
- Decreased human bias: Biases based on race, gender, or other characteristics may unintentionally be introduced by traditional credit scoring techniques. By concentrating on pertinent financial indicators rather than human traits, AI may be built to reduce these biases. This promotes consistency in credit rating methods and guarantees equitable lending practices.
- Scalability: Models for AI credit scoring are readily scalable to handle a large number of loan applications. AI can manage the workload without sacrificing quality, regardless of how many applications a lender gets each day—ten thousand or ten thousand.
- Cost savings: By automating the risk assessment process, reducing the need for human labor, and enhancing operational efficiency, AI credit scoring significantly reduces costs. By minimizing mistakes, cutting labor costs, and expediting the processing of loan applications, lenders may drastically cut operating costs.
- Fraud detection: By examining past data, AI systems are able to identify trends in fraudulent activity. This aids lenders in spotting and stopping fraud, loan stacking, and theft.
- Financial inclusion: Businesses and people who may not have conventional credit records, such as those in developing countries or with little banking experience, may find it easier to get loans thanks to AI credit scoring. AI can expand the pool of potential borrowers by using different data sources.
- Regulatory compliance: AI credit scoring systems may be made to abide by rules and can provide clear justifications for their judgments, which will help lenders fulfill their legal commitments.
- Decreased human error: Improper lending choices may result from manual credit scoring methods, which are prone to inaccuracies. AI provides consistency in decision-making and reduces the possibility of human mistake.
Conclusion
the comprehensive guide on AI in credit scoring for 2024 provides a thorough financial risk overview. The transformative applications and benefits showcased underscore the pivotal role of artificial intelligence in reshaping credit assessment and risk management within the financial industry.
Fintech is always changing, and making an app like an e-wallet requires a lot of careful planning and the newest technology. Using Appic Softwares as a guide gives you a strategy plan to get through the tough parts, which encourages innovation and makes it possible to create groundbreaking fintech apps in 2024 and beyond.
So, What Are You Waiting For?