How AI Fraud Detection Can Safegaurd Digital Transactions 2024?
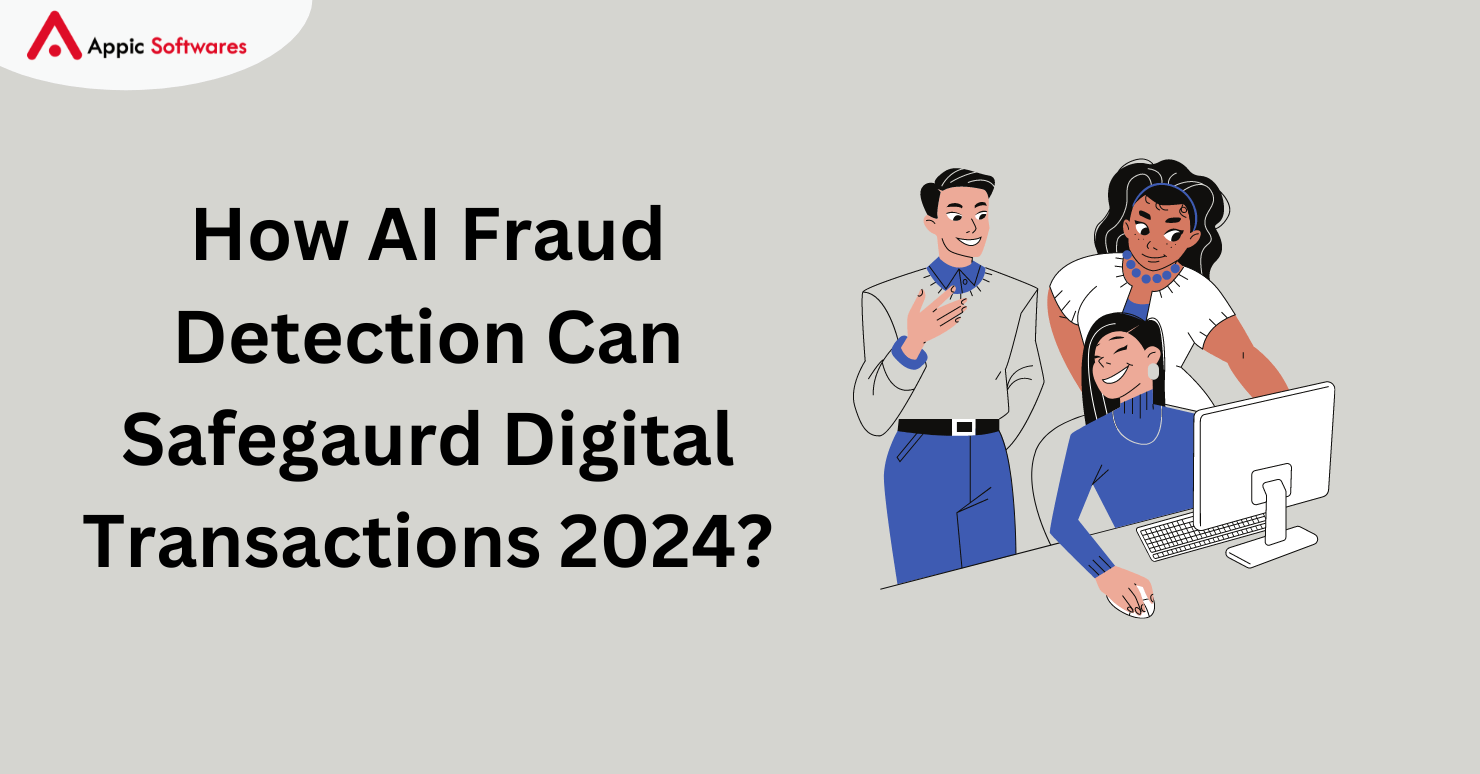
The advent of the digital age has brought about a new era of potential and ease. But it has also made room for bad actors, which has resulted in an increase in fraud in a number of industries. The potential of fraud is constant in financial transactions and online accounts, presenting serious financial and reputational hazards to both individuals and businesses.
The conventional approaches to fraud detection are frequently insufficient in this constantly changing environment. Although they are good at spotting established patterns, rule-based systems find it difficult to adjust to the ever-evolving strategies used by con artists. This has increased the demand for more complex and dynamic solutions, which has led to the development of anomaly detection systems driven by artificial intelligence.
According to industry estimations, the global anomaly detection market is expected to grow at a significant rate and reach a value of USD 26.51 billion by 2027. This increase, which is being propelled by a strong Compound Annual Growth Rate (CAGR) of 18.5% starting in 2022, highlights how anomaly detection is becoming more and more recognized for its revolutionary potential in fraud prevention tactics. These technologies provide an impressive toolkit to detect and prevent fraudulent activity with unmatched precision and effectiveness by utilizing artificial intelligence.
The process of creating a fraud-fighting AI-based anomaly detection system is explored in greater detail in this article. We will examine the most important factors, covering everything from model design and data preparation to training and continuous monitoring, giving you the tools you need to use AI to combat fraud.
- Anomaly detection in fraud prevention – what does it entail?
- Types of anomalies in fraud detection
- Exploring common methods of anomaly detection
- How does anomaly detection contribute to fortifying fraud prevention?
- Traditional anomaly detection techniques face difficulties in preventing fraud.
- AI and its advantages in anomaly detection
- Essential specifications needed to construct an anomaly detection system
- How to create an anomaly detection system based on AI
- Important things to think about when developing an AI-based anomaly detection system
- Future trends in AI-based anomaly detection
Anomaly detection in fraud prevention – what does it entail?
Overview of abnormalities
Anomalies are defined as observations or patterns found in data that substantially differ from typical or expected behavior. These abnormalities can take on a variety of shapes, from minute deviations to conspicuous distortions. Finding anomalies in a dataset is essential because they frequently point to possible problems or dangers.
Comprehending anomaly detection
Detecting entries in a dataset that seem inconsistent or out of place is a process known as anomaly detection, sometimes referred to as outlier detection. Finding anomalies, odd patterns, occurrences, or observations in the data that might point to mistakes, fraudulent activity, or other unexpected behaviors and possible risks is the main goal.
part in preventing fraud
One essential element of systems for preventing fraud is anomaly detection. Organizations can proactively detect and stop fraudulent actions before they cause major harm by recognizing abnormal behavior. This could include any suspicious activity that could jeopardize the integrity and security of the system, such as fraudulent transactions, unauthorized access attempts, or unusual user behaviors.
Applications in many industries
Many industries use anomaly detection: finance, where it helps identify fraudulent transactions and activities; cybersecurity, where it helps identify anomalous network traffic or intrusions; healthcare, where it helps identify anomalies in patient data or medical records; and industrial monitoring, where it helps detect equipment failures or irregularities in manufacturing processes. No two industries use anomaly detection more than the others.
Essentially, anomaly detection’s ability to reveal anomalies and possible dangers in datasets highlights its critical function in guaranteeing the security and dependability of data in a variety of businesses. Organizations can strengthen the robustness of their systems against malicious intent by proactively protecting against fraudulent actions by utilizing anomaly detection techniques.
Types of anomalies in fraud detection
Data may exhibit a variety of anomalies, each of which can point to a distinct kind of irregularity or unanticipated event. The primary categories are as follows:
- Point anomalies: These anomalies arise when a single data point is seen as abnormal in relation to the entire set of data. A single instance that deviates noticeably from the bulk of the data points is called a point anomaly. A point anomaly could be, for instance, an abnormally high transaction value in a string of smaller transactions.
- Contextual anomalies: These happen when a data point’s abnormality is contingent upon the circumstances surrounding it. These anomalies are deemed aberrant in a particular context, but they are not necessarily outliers in the dataset as a whole. For example, an abrupt increase in prescriptions for a certain drug during an unanticipated season or in violation of standard treatment standards suggests prescription fraud or abuse.
- Collective anomalies: Although individual data points may not be anomalous on their own, collective anomalies occur when a set of data points collectively exhibit aberrant behavior. By examining the connections or exchanges between data points, these anomalies are found. A coordinated, simultaneous set of minor transactions across multiple accounts that collectively deviate from conventional patterns and could be indicative of money laundering or account takeovers are examples of such coordinated, simultaneous transactions.
- Temporal anomalies: When the anticipated temporal behavior of the data is not followed, there is a temporal anomaly. By examining time-series data and spotting patterns that deviate from the usual temporal sequence, these anomalies can be found. For instance, during regular business hours, an employee might normally access confidential corporate information. However, an unexpected increase in late-night access requests could be a sign of insider danger or unlawful access.
Anomalies in geographical locations or the spatial relationships between data points are referred to as spatial anomalies, and they can arise in spatial datasets. By examining spatial patterns and looking for outliers in spatial distributions, these anomalies can be found. A strange login attempt that deviates from normal login patterns and comes from a location where there hasn’t been any previous user activity could serve as an example. This could indicate a possible security breach or account takeover.
Designing efficient anomaly detection systems that can precisely locate irregularities and unexpected patterns in data across numerous domains and applications requires an understanding of the various forms of anomalies.
Exploring common methods of anomaly detection
Anomaly detection is the umbrella term for a number of methods used to find anomalies in datasets. Typical techniques for identifying anomalies include:
Analytical statistics:
Using statistical analysis and measures like Z-scores, anomaly detection locates irregularities in the data. By taking this step, potential anomalies can be identified by identifying data points that deviate significantly from the mean.
Algorithms for machine learning:
Anomaly detection adapts to various datasets by using supervised and unsupervised machine learning methods. While unsupervised models identify patterns without labeled examples, increasing the flexibility of the anomaly detection process, supervised models learn from labeled datasets. Clustering-based techniques, isolation forests, and autoencoders are a few examples.
Analysis of time series:
Analyzing patterns, seasonality, and unforeseen changes across time are all important considerations when examining anomalies in time series data. This approach offers a dynamic viewpoint, spotting irregularities that could change across various time periods. One can use methods like sophisticated time series models, exponential smoothing, or moving averages.
Methods based on density:
The density of data points is another way to find anomalies. Outliers are instances with a much lower density, which helps identify abnormalities that may not be visible using conventional techniques. The Local Outlier Factor (LOF) represents one instance of a density-based methodology.
group techniques:
Multiple models or methodologies are combined in ensemble methods to improve anomaly detection capabilities. This cooperative strategy makes use of the advantages of several anomaly detection techniques to improve the system’s overall performance.
The features of the data and the particular needs of the application determine which method is best.
How does anomaly detection contribute to fortifying fraud prevention?
Anomaly detection’s diverse approach to early identification, adaptability, precision, real-time monitoring, and compliance makes it crucial for bolstering fraud prevention efforts. Let’s get into further detail about each:
- Early detection: By quickly identifying any fraudulent activity, anomaly detection makes it possible to take appropriate action before serious harm is done. Early discovery can reduce monetary losses and stop additional harm from happening to people or organizations.
- Adaptability: Since fraudulent operations are always changing, con artists come up with new strategies to elude detection techniques. Adaptability is demonstrated by anomaly detection systems’ ability to keep ahead of changing fraud trends without requiring frequent user intervention or rule modifications. This guarantees that efforts to combat fraud will continue to identify new dangers.
- Finding unknown patterns: Conventional rule-based systems could miss new fraud patterns that don’t follow established guidelines. Through the process of learning from typical user behavior, anomaly detection systems are able to discover previously unidentified or unusual fraud tendencies. To stay one step ahead of sophisticated fraud schemes, this expertise is essential.
- Reducing false positives: By precisely recognizing patterns of typical user behavior and causing the least amount of disturbance to real users, anomaly detection helps reduce false positives. Anomaly detection systems contribute to preventing legitimate transactions from being wrongly reported as fraudulent by maintaining a high degree of precision in fraud detection.
- Real-time monitoring and response: When suspicious activity is discovered, anomaly detection permits prompt responses through real-time monitoring and response. This quick action is necessary to stop fraudulent transactions from being completed and to lessen possible damages.
- Preventing account takeovers: Account takeovers can lead to financial losses and identity theft and are a serious danger to user security. By identifying suspect login attempts, odd account activity, or unauthorized access, anomaly detection plays a crucial role in stopping account takeovers and protecting user accounts and sensitive data.
- Reducing financial losses from fraud: Anomaly detection is essential in reducing financial losses from fraud because it stops fraudulent actions before they get out of hand. By being proactive, businesses may safeguard their resources and keep the confidence of their clients.
- Observance and confidence: Anomaly detection technologies help businesses maintain client confidence and brand recognition by helping them adhere to fraud protection rules. Establishing a commitment to upholding the security and integrity of their systems can help firms gain the trust of stakeholders and customers.
A proactive and essential approach, anomaly detection in fraud prevention basically uses cutting-edge methods to spot anomalies, spot possible dangers, and strengthen defenses against fraudulent activity in a constantly changing digital environment.
Traditional anomaly detection techniques face difficulties in preventing fraud.
- Unbalanced data: When compared to valid transactions, fraudulent operations are usually uncommon, which results in skewed datasets. The prevalence of normal data may make it more difficult for conventional anomaly detection techniques to spot fake patterns.
- Dynamic aspect of fraud: Since fraudsters are always changing and refining their methods, fraud trends are unpredictable and challenging to identify. Conventional approaches might not be able to quickly adjust to new kinds of fraudulent activity.
- Feature representation: An important aspect of anomaly detection is creating features that accurately depict both legitimate and fraudulent activity. Conventional techniques could depend on human feature engineering, and in a setting that is changing quickly, choosing pertinent features can be difficult.
- Limitations of unsupervised learning: A lot of conventional anomaly detection methods are unsupervised, which means they don’t require labeled data to be trained. This can lead to higher false positive rates by making it difficult to discern between genuine fraud and normal changes.
- Model sensitivity: When faced with small variations in typical behavior, anomaly detection models may be overly sensitive to shifts in the data distribution, which could result in false positives. This sensitivity could be a factor in the high false alarm rate.
- Scalability problems: Conventional approaches may not be able to scale effectively as data volumes rise. Processing huge databases in real time can be difficult, which affects how quickly fraud can be identified.
- Adversarial attacks: By purposefully changing their behavior or adding noise, fraudsters may actively try to trick detection systems. Conventional techniques might not be strong enough to fend off such hostile attempts.
- Inability to explain: Preventing fraud requires knowing why a certain incident is marked as abnormal. However, interpretability may be lacking in traditional approaches, especially in sophisticated ones, which makes it difficult for investigators to comprehend the logic behind a finding.
- Evolution of technology: Sophisticated strategies are also used by fraudsters due to their embrace of advanced technologies like machine learning. In the face of quickly changing technology environments, traditional practices could become antiquated and less effective.
- Integration difficulties It can be difficult to integrate anomaly detection technologies with current fraud prevention workflows and systems. The seamless integration of traditional methods with contemporary technology and platforms may present obstacles throughout the implementation phase.
- Limited context awareness: Contextual data, such as transaction history or user behavior trends, is frequently ignored by traditional approaches. Accurately identifying anomalies becomes harder in the absence of context.
In conclusion, problems with scalability, dynamic fraud patterns, and imbalanced data are some of the difficulties that classic anomaly detection techniques encounter. It will take the use of cutting-edge technology, improved interpretability of the model, and integration of contextual data to overcome these obstacles. Organizations can effectively strengthen their fraud prevention efforts against rising risks by adapting their tactics to suit these objectives.
AI and its advantages in anomaly detection
The many benefits of AI-based anomaly detection outweigh the drawbacks of conventional techniques. Here is a summary of some of the main advantages:
- Scalability: The capacity of AI-based anomaly detection to grow is one of its main benefits. Large data quantities are typically difficult for traditional approaches to handle effectively. Artificial intelligence (AI) methods, in particular machine learning and deep learning models, are perfect for real-time anomaly identification in large datasets because they can handle enormous volumes of data quickly.
- Complex pattern recognition: By examining intricate patterns in data, AI is excellent at spotting anomalies. From past data, machine learning algorithms are able to discern regular patterns and spot departures from them. Neural networks and other deep learning approaches are particularly good at detecting minute irregularities buried in complex datasets, even ones with high-dimensional features.
- Decreased false positives: When compared to conventional rule-based methods, AI-based anomaly detection systems can drastically lower false positives. Artificial intelligence (AI) systems may differentiate between truly anomalous events and innocuous variations by learning the system’s regular behavior. This allows for more precise detection and fewer false alarms. By reducing pointless alarms, this feature frees up human operators to concentrate on actual dangers.
- Unknown anomaly detection: AI-powered anomaly detection is capable of detecting unique or unknown abnormalities, in contrast to rule-based systems that depend on predetermined thresholds or rules. Artificial intelligence (AI) algorithms can identify anomalies without prior knowledge of what typical behavior is by utilizing strategies such as unsupervised learning. This talent is very helpful in situations where new kinds of abnormalities appear out of the blue.
- Early detection: Anomalies can be found by AI-based anomaly detection systems early on, frequently before they become serious problems. These systems can quickly identify departures from expected behavior by continually monitoring data streams in real-time, allowing for preemptive intervention to reduce potential dangers. In many applications, early detection can avert expensive downtime, security lapses, or unfavorable outcomes.
- Multimodal data analysis: Artificial intelligence (AI) anomaly detection is not confined to the examination of structured data; it can also encompass unstructured data kinds including text, photos, and audio. This feature improves the detection of intricate anomalies spanning several modalities by enabling thorough anomaly detection across a variety of data sources. AI is used in cybersecurity, for instance, to identify sophisticated cyberthreats by analyzing system logs, network traffic records, and user behavior patterns.
- Constant enhancement: Through feedback loops, AI-driven anomaly detection systems can enhance their performance over time. These algorithms can be improved and made more accurate by adding input from human specialists or from the results of earlier detections. Through experience, the anomaly detection system will become more dependable and efficient thanks to this iterative learning process.
- Efficiency and automation: Artificial intelligence minimizes the need for human supervision and intervention by automating the anomaly detection process. By swiftly detecting abnormalities without requiring human interaction, this automation increases operational efficiency and enables businesses to spend resources more wisely. AI can also rank warnings according to how serious they are, which allows for quicker reaction times to important abnormalities.
- Predictive analytics: Using historical data trends and predictive models, AI-powered anomaly detection is able to anticipate future anomalies in addition to detecting existing ones. Organizations may proactively mitigate developing risks and vulnerabilities before they become real anomalies by utilizing predictive analytics. By being proactive, possible disruptions are reduced and resilience is strengthened.
- Adaptability to data variability: The inherent complexity and variety of real-world data frequently provide a challenge for traditional anomaly detection techniques. By adapting and learning from a variety of data sources, AI models, on the other hand, are able to capture the complex linkages and dependencies that may distinguish between normal and aberrant behavior. Furthermore, sophisticated AI methods like deep learning can automatically extract pertinent features from unprocessed data, eliminating the need for human feature engineering and boosting the resilience of the model.
- Anomaly interpretability: Although AI models are well known for their accuracy in predicting outcomes, at times their internal workings might seem like “black boxes,” making it difficult to understand the reasoning behind them. This is particularly problematic in important applications where explainability is crucial. But new developments in interpretable AI, such explainable neural networks and attention processes, hope to clarify how complex models make decisions so that interested parties can comprehend and believe the anomalies that are found.
Essential specifications needed to construct an anomaly detection system
To ensure the effectiveness and feasibility of an anomaly detection system, it is necessary to meet a number of critical conditions. Here, we list the five essential components that are necessary for the system to function well.
-
Thorough data processing
Any anomaly detection system’s core competency is its capacity to manage the complexity of real-world data. Effective preprocessing is essential, dissecting the data into its basic elements, including trend, seasonality, and residual noise. This phase increases anomaly detection accuracy and builds user trust by offering clear insights into the detection process. Furthermore, the system needs to be built to work well with unlabeled data using reliable algorithms that can adjust to different data distributions, as labeled anomaly data is frequently not accessible in commercial datasets.
Suggestions:
- Use strong preprocessing methods to efficiently validate and decompose signal components.
- Set reasonable cutoff points for non-parametric identification techniques.
- Recognize that there might not be pre-labeled anomalies in datasets and make plans appropriately.
-
Accessibility and scalability
As anomaly detection becomes more widespread, the system needs to show that it can scale to support a large number of metrics and time-series data. Even with growing volumes of data, anomalies should be easy for users to discover and understand. Information overload can be avoided and actionable and easily digested insights can be made sure of by putting in place systems to filter and expose relevant anomalies to specific users. Furthermore, the system must to facilitate smooth integration with current tools and infrastructure, enabling broad adoption among various user groups.
- Suggestions:
- To avoid information overload, create systems for sorting among anomalies and providing pertinent ones to certain users.
- When designing the user interface, give usability and accessibility top priority so that anomalies can be easily accessed and interpreted as data volume grows.
-
Mitigation through false positives
False positives have the potential to damage an anomaly detection system’s efficacy and erode user confidence. The system needs to have strong procedures in place to reduce false positives while keeping the false-negative rate low in order to reduce this danger. To optimize performance, this involves carefully validating detection results against historical data and human understanding. This enables algorithmic modifications. Furthermore, granting users the capacity to adjust detection parameters and participate in the decision-making process can improve the system’s dependability and flexibility in changing circumstances.
Suggestions:
- Put waiting methods in place for data that is incomplete or missing.
- Review and adjust the algorithm frequently in response to user input.
-
Taking recognized occurrences into account
Systems for detecting anomalies must take into account recognized occurrences or trends that can affect the behavior of the data. Contextual information about the events is incorporated into the system so that it can distinguish between true anomalies and predicted deviations. To improve the system’s accuracy and relevance, this may entail modifying expected values based on past observations or silencing notifications during known events.
Suggestions:
- Update event-based models and algorithms often to take into account shifting environmental conditions and business dynamics.
-
Contextualization and insight sharing
The anomaly detection system should have an intuitive user interface that lets users investigate discovered abnormalities, communicate insights with stakeholders, and work together on problem-solving activities in order to promote knowledge sharing and cooperation. A well-designed interface should put accessibility and usability first, giving users short explanations and easy-to-understand visualizations of abnormalities that have been found. Furthermore, the business may promote a culture of data-driven decision-making and expedite information exchange by integrating with communication and collaboration platforms.
Organizations may build a cutting-edge anomaly detection system that satisfies expectations and offers useful insights for well-informed decision-making by attending to these essential requirements.
How to create an anomaly detection system based on AI
It takes a methodical strategy that covers several phases, from comprehending the problem domain to real-world deployment and continual development, to build an efficient AI-based anomaly detection system. We will go over each stage in-depth in this extensive tutorial, emphasizing the important factors and best practices related to creating a reliable anomaly detection system.
Recognizing the issue:
Any anomaly detection system starts with a thorough examination of the problem domain. This entails taking into account a variety of abnormalities seen in the data, from outliers and uncommon occurrences to malevolent activity. Determining the acceptable risk level and comprehending the financial ramifications also require assessing the effects of false positives and false negatives on the system or organization. This method is further enhanced by close collaboration with domain experts, who offer deep insights into the subtle differences between normal and aberrant behavior.
Gathering and preparing data:
It is necessary to gather a wide range of data from many sources in order to fully encompass the range of actions and behaviors. User profiles, transaction logs, and history records are just a few of the abundant data sources that offer insightful information. To preserve confidence and compliance, it is crucial to guarantee tight adherence to privacy and data integrity laws. The next step is a thorough data preparation procedure that includes the extraction of pertinent aspects including IP addresses, timestamps, buyer and seller information, and payment amounts. The highest standards of data quality are ensured by meticulous data cleaning and handling of missing values, outliers, and inconsistencies. Furthermore, the data is prepared for additional analysis by applying sophisticated encoding techniques to categorical variables and normalizing numerical features.
Engineering features:
In order to improve the performance of the model and derive valuable insights from unprocessed data, feature engineering is essential. It is crucial to carefully discover and extract attributes that offer insights on user behavior and transactions. To create informative features, this entails fusing subject matter expert input with domain knowledge. A thorough picture of the data is ensured, and the model’s comprehension of the underlying patterns is enhanced, by using both raw and derived features, such as transaction frequency and account balances.
Model choice:
A crucial choice that has a big impact on the system’s efficacy is choosing the right anomaly detection algorithms. It is crucial to assess various algorithms, such as supervised learning, clustering methods, and time-series analytics, in light of the needs of the problem and the properties of the data. Supervised learning methods train machine learning models for fraud prediction by using past data with known outcomes. While time-series analytics methods offer insights into behavioral trends across time, clustering algorithms enhance supervised learning by spotting anomalous patterns or outliers. It is imperative to evaluate the applicability of algorithms like Gaussian mixture models, autoencoders, one-class SVMs, and isolation forests, taking into account aspects like interpretability, scalability, and real-time processing capabilities.
Training and assessing models:
To guarantee strong performance, model training and evaluation must be done with great care. Preventing overfitting and promoting generalization is achieved by carefully dividing the dataset into training, validation, and testing sets. Performance is optimized by training the chosen model with the training set and adjusting hyperparameters with the validation set. Thorough evaluation and validation is ensured by applying metrics like area under the ROC curve, precision, recall, F1-score, and recall to measure the model’s performance on the testing set.
Methods of explanation:
Sophisticated explanation approaches must be used in order to give the model’s decisions transparency and interpretability. Techniques like as feature importance analysis, LIME, and SHAP values are used to confirm the model’s conclusions and clarify the sources causing anomalies. Collaborating with domain experts to analyze the model improves its explainability and makes actionable insights easier to obtain.
Adjusting and optimizing:
To improve overall performance and generalizability, the model’s parameters and hyperparameters must be carefully adjusted. In order to comply with certain business requirements and limitations, the threshold value for anomaly detection is optimized to achieve a balance between false positives and false negatives.
Combination:
A smooth deployment and operation of the model depend on its seamless integration with the current infrastructure. Important steps in the integration process include wrapping the model into a service with a strong API, rigorously evaluating performance, and deploying the model—possibly in the form of a Docker container. Model performance is further improved and risks are reduced by human validation and parallel testing with current fraud detection systems.
In the real world:
Thorough testing, validation, and performance monitoring are necessary before deploying the trained model in a real-world setting. Prompt detection of irregularities in incoming transactions can be achieved by using sophisticated monitoring and alerting systems, and notifying pertinent parties can aid further inquiry. Ensuring the deployed system is scalable, reliable, and secure is critical to managing massive data sets and protecting confidential information.
Constant enhancement:
A strong monitoring system makes it easier to evaluate the model’s performance on a regular basis in a real-world setting. Actively gathering user and domain expert feedback facilitates the identification of possible areas for improvement, and regular updates that incorporate new features, data, or algorithms guarantee that the model is flexible enough to respond to evolving risks and patterns. Periodic evaluations and audits of the anomaly detection system guarantee that it remains relevant and effective throughout time.
Sharing of knowledge and documentation:
Transparency and reproducibility require thorough documentation that covers the whole development process. The promotion of knowledge-sharing initiatives within the organization via targeted training sessions, well-organized documentation, and knowledge-sharing platforms fosters a collaborative culture that supports ongoing learning and gives teams the tools they need to create and manage efficient anomaly detection systems.
Organizations may create efficient anomaly detection systems that can identify and neutralize threats across a range of domains by adhering to the comprehensive instructions provided in this guide and utilizing cutting-edge methods and industry best practices. Organizations can maintain the security and integrity of their systems and data while staying ahead of evolving threats through proactive monitoring, ongoing feedback, and a collaborative and learning culture.
Important things to think about when developing an AI-based anomaly detection system
The development of an AI-based anomaly detection system is a challenging undertaking that necessitates careful consideration of a number of important aspects. To help you better understand, consider these important points:
Information
- Quantity and quality: Having pertinent, high-quality data is crucial. Make sure your dataset is well-maintained and complete, with enough anomaly cases to support efficient model training.
- Labeling: In order to support supervised learning, labeled data must be obtained, which can be expensive and time-consuming. As an alternative, investigate unsupervised or semi-supervised techniques to lessen the burden of labeling.
Drift: Over time, data distributions may change, making trained models useless. Keep an eye on your system and make necessary adjustments to allow for changing data trends.
Model selection and instruction:
Choose algorithms based on the properties of your data and the definitions of anomalies. Statistical techniques, machine learning, and deep learning methods like LSTM networks or isolation forests are among the options.
- Hyperparameter tuning: Adjust model parameters to minimize false negatives and false positives while maintaining a reasonable degree of precision.
- Explainability: Give top priority to models with comprehensible outputs that make it evident why anomalies are detected.
System architecture and implementation:
Define appropriate levels for false positives and false negatives by weighing the costs of looking into false warnings against the possible repercussions of missing abnormalities.
- Adapting to evolving anomalies: In order to stay up to date with shifting behavioral patterns and new threats, anomaly detection systems need to be updated on a regular basis. The AI models that drive the detection system need to be updated and retrained on a regular basis in order to remain successful when new abnormalities appear and old ones change. To guarantee that the anomaly detection system stays reliable and able to identify both known and unknown anomalies, constant observation and modification are necessary.
- Alerting and escalation: Create efficient workflows to manage system alerts, guaranteeing prompt action and suitable escalation protocols.
- Monitoring and feedback: To improve accuracy and effectiveness iteratively, continuously evaluate the performance of the system and ask users for their comments.
Security and privacy of data:
- Privacy compliance: Verify that your system complies with applicable privacy laws, protecting private information as it detects anomalies.
- Security precautions: Put strict security measures in place to guard against unwanted access or system manipulation, ensuring user confidentiality and data integrity.
Future trends in AI-based anomaly detection
AI-based anomaly detection systems have a bright future ahead of them, one that should increase their efficacy and expand their range of uses. The following major themes are expected to influence the future:
-
Improved flexibility and learning:
- Adaptive learning: As a result of the data that AI systems process, they will continuously learn from it and develop. This will allow them to quickly identify anomalies that depart from established norms and adjust to changing patterns. Remaining ahead of sophisticated threats and developing anomalies requires this adaptive learning.
- Unsupervised learning: There will be less dependence on labeled data, which can be expensive and rare. Unsupervised learning techniques will be used more often by AI systems to detect abnormalities, even in cases when the precise signs of these anomalies are not known in advance. This strategy will greatly improve the system’s capacity to be applied in a wide range of contexts and to be generalized.
-
More sophisticated methods and deeper integration:
- IoT integration: The Internet of Things (IoT) infrastructure will be easily integrated with AI-based anomaly detection. Through real-time monitoring and analysis of data streams from various sensors and devices made possible by this integration, anomalies will be found more quickly and thoroughly in a variety of applications, including industrial automation and smart cities.
- GANs, or Generative Adversarial Networks: By creating artificial “normal” data using these models, the system can be trained to recognize real-world data points that differ markedly from predicted patterns, thereby revealing latent anomalies.
-
Overcoming obstacles and broadening the scope:
- Interpretability: The goal is to create models that are easier for human specialists to comprehend so they can comprehend the reasoning behind the decisions made by the system. This openness promotes trust and makes it easier to respond to abnormalities that are found in an efficient manner.
- Explainable AI (XAI): By using Explainable AI approaches, the system’s actions will have explicit explanations, promoting transparency and increasing user confidence in its abilities.
- Privacy considerations: Robust privacy-preserving approaches will be crucial as AI systems handle ever-more-sensitive data. In order to protect data security and privacy and facilitate efficient anomaly detection, it will be imperative to investigate differential privacy and federated learning techniques.
With the help of the developments described in emerging trends, AI-based anomaly detection systems are going to play a major part in strengthening a variety of industries. These systems are positioned to play a key role in improving security, efficiency, and dependability across a range of disciplines, from bolstering cybersecurity and fraud detection to advancing healthcare and industrial process monitoring.
Conclusion
We hope that this guide has helped you understand How AI Fraud Detection Can Safegaurd Digital Transactions 2024?. Additionally, Appic Softwares is the top Finance app development company that you should check out.
What Are You Waiting For, Then?