What Is Predective Analysis In Fintech? Use Cases & More
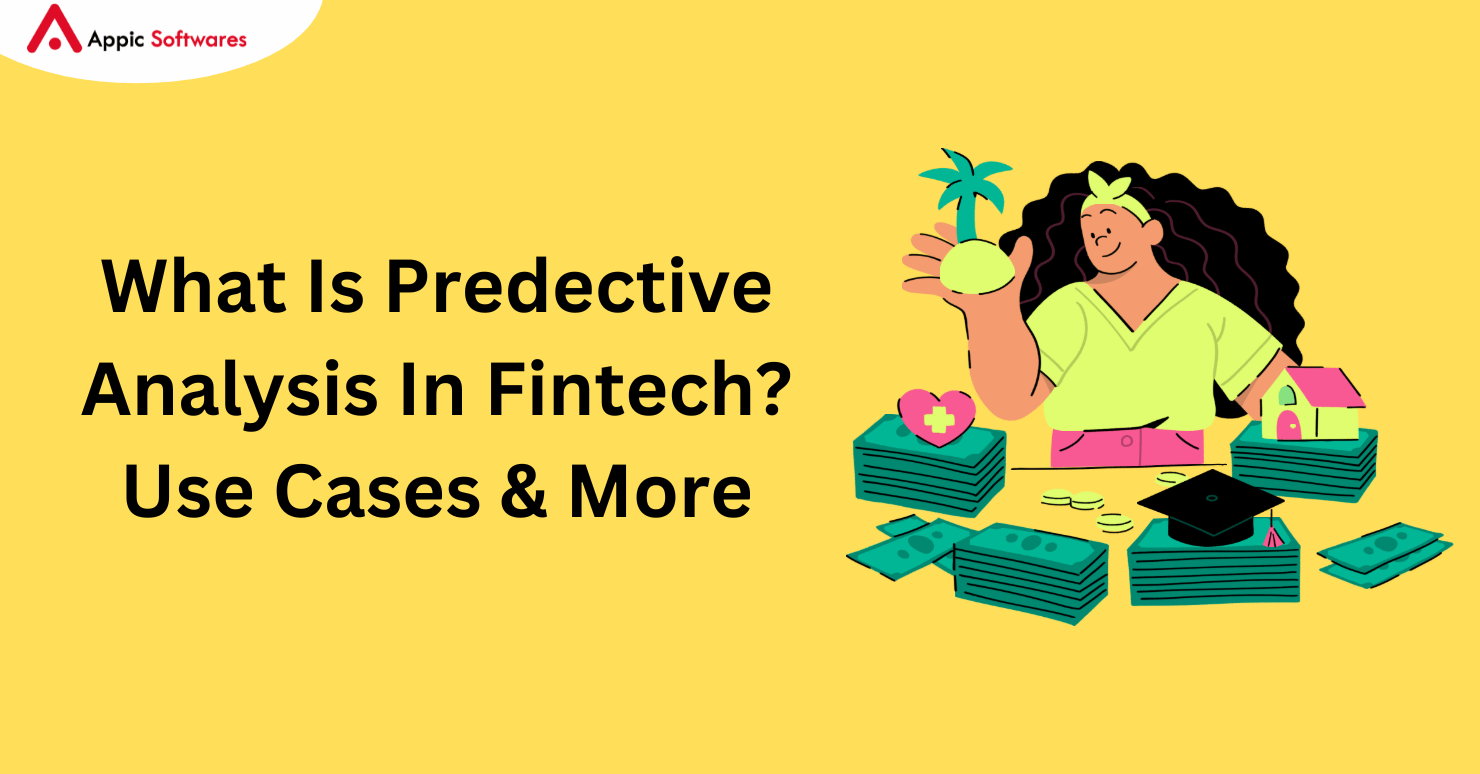
There are 2.5 million ants for every human on Earth. That’s analytics. You can find out how many ants there will be for every human in 100 years using predictive analytics. What is its fintech capability?
Learn about the advantages and difficulties of predictive analysis in the Fintech sector, as well as where to use it and how to integrate it most effectively into your offering.
P.S. Do you believe that you will be outnumbered by the 2.5 million ants that nature has in store for you? Discover more at the article’s conclusion.
- Predictive analysis and its methods explained
- Common misconceptions about predictive analytics
- Why FinTech needs predictive analytics
- Use cases for predictive analysis in FinTech
- Implementing predictive analytics in financial products and services
- Potential dangers and obstacles, and strategies for overcoming them
Predictive analysis and its methods explained
In order to identify trends and generate forecasts for companies and their clients, predictive analytics employs machine learning algorithms, statistics, and other techniques.
It is necessary for your teams to gather and prepare the data before performing this kind of study. After that, you train the models, evaluate their accuracy, and choose appropriate modeling strategies. Selecting from a range of software tools and platforms allows you to concentrate on the unique requirements and objectives of your company. You can also employ many approaches from the list below, either separately or in combination, to leverage analytics to accomplish your objectives.
Artificial intelligence (AI) is the study of past, present, and future events and trends via the use of techniques and technologies. To identify patterns, connections, and trends in the data, it makes use of machine learning models and statistical techniques. Through this method, organizations can forecast results and use insights from pertinent data to guide their decisions.
Using methods from statistics, machine learning, database administration, and artificial intelligence, data mining is the methodical extraction of significant patterns and insights from large datasets for predictive analysis and well-informed decision-making.
A type of artificial intelligence called machine learning (ML) enables systems to learn from data and improve performance over time. It is used in finance to detect fraud, make investment decisions, and forecast market trends.
- Modeling: The development and application of mathematical or computational models for the purpose of classifying, predicting, or deriving insights from data is known as modeling in predictive analytics. By using modeling, you may develop algorithms that can learn from past data, allowing them to identify patterns and draw conclusions about previously unseen data.
- Statistics: By offering a framework for drawing conclusions and making decisions based on the gathering, analyzing, interpreting, presenting, and organizing of data, statistics plays a critical role in deriving useful insights from data.
- Mixed approach: Predictive analytic software can combine machine learning, cutting-edge data mining techniques, and traditional statistical methodologies. For example, these sophisticated models go beyond user-specified predictors in the prediction of portfolio risk. Rather, they independently identify predictors through the analysis of complex interactions among several nonlinear risk variables. With this method, particular combinations of risk factors are identified that can aid in the prediction of future events.
Common misconceptions about predictive analytics
Machine learning versus predictive analytics
Predictive analytics makes educated assumptions about future events based on historical data. It entails analyzing past data using machine learning and statistical methods to estimate or forecast potential future events based on past experiences. It’s a method of using data patterns to make well-informed forecasts. Thus, one tool that predictive analytics uses is machine learning.
Contrasting Prescriptive and Predictive Analytics
While predictive analytics makes predictions about the future, prescriptive analytics takes a step further and makes recommendations for actions based on those forecasts. Prescriptive analytics aids in selecting the optimal growth plan, for instance, if predictive analytics indicates that a company’s revenue may plateau in the future. It simulates possible results for various strategies, directing choices toward the most promising course, such as choosing a product that will probably increase sales.
Why FinTech needs predictive analytics
Predictive analytics has several advantages, ranging from better fraud detection and risk management to more effective operations, enhanced investment decision-making, and a general competitive edge.
- FinTech organizations may effectively assess and manage risk with the use of predictive analytics.
- Security protections are improved using machine learning algorithms, which allow for constant adaption to novel fraud patterns.
- Predictive analytics is essential to the credit scoring and underwriting procedures in lending. It examines income, spending habits, and credit history to provide more precise evaluations of creditworthiness.
- Fintechs can increase client pleasure and loyalty by providing customized financial products, investment recommendations, and personalized user experiences through the analysis of consumer data.
- Predictive analytics is used by fintechs to improve pricing models, customer retention, and operations. This include estimating demand, allocating resources effectively, and automating repetitive tasks to boost productivity.
Use cases for predictive analysis in FinTech
This kind of data analysis is essential to the financial industry for controlling risks, predicting market trends, and making the best possible investment choices.
Give credit
Predictive analytics plays a major role in credit scoring. Data from the applicant’s credit history and the credit records of borrowers with comparable characteristics are used in the credit application process when an individual or business requests credit. This data is used to predict the possibility that the applicant will have trouble meeting obligations associated with any given credit.
Evaluation and control of risks
Financial professionals may assess and manage risks associated with loans, investments, and other financial products with the help of predictive analytics. Through the examination of past data and the identification of patterns and connections, these algorithms assist in identifying possible hazards and advantages as well as facilitate risk management decision-making inside financial organizations.
Portfolio management and investment analysis
By examining past market data and seeing patterns and trends, businesses can evaluate investment performance and forecast future returns. This helps financial experts to decide on portfolio management strategies with knowledge.
Undertaking
Data and predictive analytics can significantly help insurance businesses with their underwriting procedure. Insurers can forecast the possibility of future payouts by rating insurance applicants, examining the risk pool of comparable policyholders, and reviewing historical claim occurrences. In order to assess risk more accurately, actuaries frequently use predictive models that consider a variety of parameters and compare them to information on previous policyholders and claims.
Targeting and segmenting customers
By taking into account variables like income, credit history, and spending patterns, financial organizations use these procedures to divide up their clientele. This segmentation is made feasible by predictive analytics, which also provides a deeper understanding of the requirements and preferences of the consumer. Institutions can then develop targeted digital marketing campaigns and tailor their offerings to meet the unique needs of certain consumer segments.
Allocating resources and creating a budget
Financial firms can examine future financial performance by analyzing past data and looking for trends and patterns by utilizing predictive analytics. These algorithms help forecast future earnings, costs, and total profitability. Financial institutions are empowered by this foresight to decide strategically how to allocate resources and plan for the future by using well-informed views.
Identification and prevention of fraud
In financial services, predictive analytics makes it possible to look at transactions, trends, and patterns. Every unusual activity is carefully examined for signs of fraud. Examining activity between bank accounts or seeing odd trends in transaction timing may be part of this investigation.
Implementing predictive analytics in financial products and services
Selecting the appropriate model
Two sets of models that reflect various viewpoints on predictive analytics are shown below. Although they are not precisely subgroups of one another, their applications may lead to a relationship between them. Let’s dissect every group.
Models for predictive analytics are made to evaluate past data, find trends, identify patterns, and utilize that knowledge to forecast future trends.
In the financial industry, three well-known predictive models are frequently used:
- Classification model: This model’s binary output generates predictions by giving the topic a general evaluation. For instance, it can predict whether the shares of a specific company will rise or fall in the banking industry.
- Outliers model: Designed to identify notable deviations within a dataset, the outliers model is particularly useful in the identification of fraudulent activity. In the event that a customer’s credit card is used in an unexpected way in a location they don’t often visit, the outlier model would flag the transaction as possibly fraudulent.
- Time series model: This model forecasts how a given variable will be affected at a later time by tracking a certain variable over a predetermined amount of time. This model is frequently used in finance to predict long-term changes in things like economic circumstances or securities prices.
These models have different uses, but they can also be used in related fields. For example, in trading, time series models can be used for forecasting or regression to anticipate stock prices, while an outliers model can be used to identify potentially fraudulent trading activity.
Models from data science that are utilized in predictive analytics follow next.
- Models of statistics. These handle numerical data and use trends to compute metrics for the future. They are most useful for forecasting steady quantitative KPIs in finance and are less expensive than the other two.
- Machine learning models that do not use neural networks (NNs). Non-NNs anticipate a wide variety of variables (e.g., risk, revenue, expenses, etc.) by examining multiple factors for each variable. They interpret multi-dimensional data, which requires structuring. Because of this, they are highly effective for batch predictive analytics but also fairly costly.
- Models of deep neural networks (DNNs). These are the most expensive of the three and make use of AI. DNN models work well in real-time predictive analytics because they handle enormous volumes of structured and unstructured financial data. DNN models recognize and analyze complex non-linear connections to automatically discover changing factors for the required variables and deliver accurate forecasts.
Traditional statistical procedures fall under the larger area of statistical models. On the other hand, within the larger subject of machine learning, deep neural network models and non-neural network models reflect distinct paradigms.
Although there may be some crossover and integration between these categories—particularly in light of recent developments such as neural network-based time series models—their fundamental ideas and working methods usually set them apart. In actuality, the type of data and the precise objectives of the research determine which model is best.
Selecting appropriate architecture
A FinTech predictive analytics solution’s design should take into account a number of elements, such as the type of data being used, the intricacy of the analytics jobs involved, the need for scalability, and regulatory issues. Now let’s examine which FinTech architectures work well with predictive analytics.
Small-scale services
A microservices architecture splits the program up into discrete, stand-alone services. They can be created, implemented, and scaled independently of one another. Every microservice is in charge of a certain business function.
FinTech applications that handle a variety of functions, like risk management, fraud detection, and client segmentation, are well suited for this kind of software architecture, which enables each service to focus on a particular area of expertise. Your solution will become more scalable and agile with the help of microservices, making fault isolation, upgrades, and maintenance simpler.
Architecture motivated by events
An event-driven architecture reacts to events and sets off the proper reactions by processing events in an asynchronous manner. This is especially helpful for real-time analytics, processing optimization, adaptability to changing circumstances, and scalability.
Event-driven architecture can be used for customized consumer interactions, market monitoring, and real-time fraud detection.
Architecture of data lakes
A data lake is a centralized location where your company may store data at any size, both structured and unstructured. With centralized data storage and flexibility in processing various data kinds, it lets you execute machine learning algorithms and analytics tools on a variety of datasets. As with the other two architectures, this one can be expanded.
A data lake can hold past transaction data, customer information, and external market data for FinTech predictive analytics, offering a thorough source for analysis.
Architecture without servers
Developers can write programs using serverless computing without having to worry about maintaining the underlying infrastructure. Functions in this kind of architecture are carried out in reaction to triggers or events.
Because of its low cost, this architecture guarantees automatic scalability and minimal operating overhead. It can be used for certain tasks like model inference or data pretreatment in a FinTech analytics pipeline.
An illustration of a mixed architecture is shown below, which combines aspects of event-driven design with microservices architecture.
Real-time and batch insurance data processing and analysis take place in separate flows in this scenario. An already trained DNN produces accurate predictions on its own for the key variables. Teams can use these forecasts to aid in operational and strategic planning by storing and visualizing them. Instantaneous real-time insights are transmitted to pertinent systems, prompting actions such as dynamic premium adjustments, fraud alerts, and price notifications.
Potential dangers and obstacles, and strategies for overcoming them
Although predictive analytics presents numerous advantages, there is a challenging aspect as well. A number of the most typical predictive analytics mistakes are listed here, along with solutions.
- data caliber. Predictive modeling can be compromised by incomplete, out-of-date, or erroneous data, which can lead to forecasts that are not accurate. Thus, when gathering information from various sources, be cautious to verify the quality of the data. Establish strong data governance procedures to keep data management organized and consistent while reducing the possibility of inaccurate or deceptive forecasts and insights.
- Price. As previously stated, maintaining excellent data quality is essential. However, it might be expensive as it calls for expenditures in methods and instruments for data cleaning. A significant portion of your budget will go toward developing, testing, and maintaining the requisite technological infrastructure; hiring qualified data scientists, analysts, and specialists with predictive analytics experience; and developing and testing models. It’s important to evaluate your resources if you plan to locate a vendor and use their predictive analytics solution into your systems.
- security and privacy. Your analytics will be using a ton of personal data, therefore it needs to be handled cautiously and in compliance with all applicable laws and guidelines. Concerns about data privacy and procedure fairness rise annually with the volume of data used. Ensure that your teams create robust data protection rules and procedures to prevent fraudulent or identity theft by protecting client information from careless and unsecured use.
- excessive model fitting. When a model learns noise or random oscillations in addition to the underlying patterns found in the training data, this is known as overfitting. The data is very particular to the training set because of this sensitivity. Overfitting in the context of FinTech can result in a number of issues, including erroneous risk assessment, poor generalization to novel market conditions, and misleading outcomes. All of that will probably have a detrimental effect on the client’s portfolio and, in certain situations, result in significant losses.
- Regularization and strong model validation strategies must be used to reduce overfitting problems. Make sure that a variety of datasets are used, representing the complexity of real-world situations. Recall that constant observation and updating of models is necessary to preserve their relevance and accuracy in the field where context is changing instantaneously.
- Inability to be understood. Different systems, apps, or components can communicate and use information in a coordinated manner more efficiently when they are interoperable. Chaos-ridden systems that fail to exchange vital information result in biased models, difficulties establishing model governance, opaque stakeholder communications, and regulatory noncompliance. The trust that you have gained from partners and clients is eroded by everything mentioned above.
All of the aforementioned issues have the potential to develop into larger, more severe micro-challenges. To give more thorough advice, it’s essential to comprehend the specifics of your product or service and the business environment in which it operates.