Machine Learning In Fintech: Top 7 Uses Cases
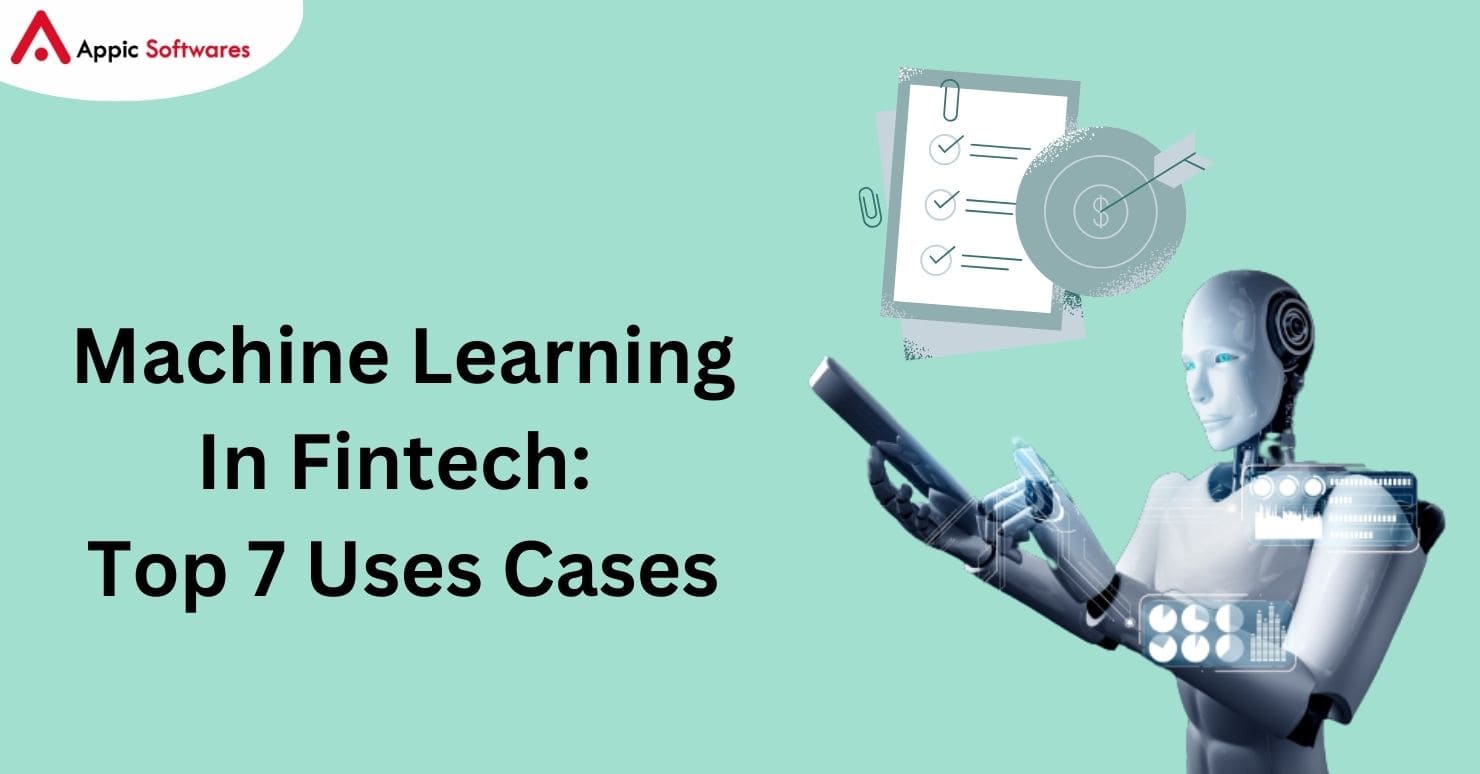
ML is becoming more and more popular in FinTech for everything from making business decisions to making things run more smoothly. And since many banks have put a lot of money into using machine learning technology, is this a match made in financial heaven?
The banking sector is always looking for new ways to use machine learning in their daily work so that they can make more money and give better customer service. The financial business is a great fit for machine learning because of its strong historical trends, large amounts of structured and unstructured data, and focus on numbers.
A new survey found that about 70% of all financial services companies use machine learning to find fraud, predict events that will affect cash flow, and improve credit scores.
Find out if your FinTech company needs to use machine learning by reading about the top 7 ways it is used in FinTech.
Table of Content
- How do machine learning and FinTech work together?
- Top 7 ways that machine learning is used in fintech
- Does the bank you work for need to use machine learning?
- Conclusion
How do machine learning and FinTech work together?
FinTech businesses are constantly getting new data because of changes in the market, the actions of millions of customers, and multiple attempts to do something illegal. In this kind of setting, it’s not possible to keep track of all processes by hand and give accurate reports on these huge operating systems.
Finding patterns is one of the best uses of machine learning in FinTech. They find connections between a huge number of events and patterns, pulling out useful data that is hidden in huge amounts of data that people normally miss. Machine learning (ML) can learn and make predictions, which helps FinTech companies find new business chances and come up with good strategies.
So it makes sense that machine learning and FinTech have joined forces so quickly. The FinTech market for AI is expected to grow at a compound annual growth rate (CAGR) of 16.5% from 2022 to 2030, hitting $41.16 billion by 2030.
Top 7 ways that machine learning is used in fintech
A picture showing the seven best ways that machine learning is used in FinTech
As market trends change, FinTech businesses can use AI and ML to offer services that are focused on the customer. In FinTech, the best examples of how machine learning is used are:
- Trading through machine learning and algorithms
- Machine learning and finding and stopping scams
- Using machine learning to follow the rules
- IoT to help customer service get better
- Using AI to learn how to trade stocks
- How machine learning can help automate loans
- Use of machine learning for decision making and data analysis
Let’s talk about the specifics.
#1: Trading based on machine learning and algorithms
Companies use algorithmic trading to make their financial choices automatically and make more trades. It is a very effective strategy. It includes carrying out trading orders in line with trading instructions that have already been programmed and made using machine learning algorithms. Every big financial company puts money into algorithmic trading because it’s hard to make as many trades by hand as ML technology can.
AI and machine learning are used by 8topuz to help buyers make more money, for example. An automated trading system driven by artificial intelligence is offered by the company. ML algorithms are used by 8topuz to look at huge amounts of past data from different stock markets. This helps investors come up with better algorithmic trading strategies.
#2: Machine learning and stopping and finding fraud
According to PwC’s Global Economic Crime and Fraud Survey, 46% of companies polled had experienced fraud, corruption, or other economic crimes in the past 24 months.
But advanced deep learning models that have been trained on huge amounts of data make it possible for organizations to find secret connections between different data points and quickly spot outliers.
One of the biggest banks in Denmark, Danske Bank, which used to use an old rule-based system to find fraud, cut down on false positives by 60% and raised the number of frauds found by 50%.
A smart tool that uses machine learning for analysis and helps NFT fans avoid fraud is one of our most recent success stories.
#3: Using machine learning to follow the rules
A lot of big businesses say that keeping up with regulations can cost up to $10,000 per worker on average.
Regulatory Technology (RegTech) uses are some of the best ways to use machine learning in banking. ML algorithms can find links between rules because they can read and learn from a lot of regulatory papers. So, cloud solutions for finance that include machine learning systems can automatically keep up with and report on changes to regulations as they happen.
Additionally, banks can keep an eye on transaction data to spot any oddities. ML can then make sure that all customer deals meet the rules set by regulators.
#4: Using machine learning to give better customer service
Personalization is the best way to get people to trust you and stick with you. People respect honesty and accuracy, especially when it comes to money matters. In this case, FinTech machine learning can look at data about users and guess what services they might like or give them good advice.
Capital One, for example, has released Eno, a tool that can, among other things, track how much money people spend. The program can check carefully to see if a customer has been charged twice for the same item or service and let them know.
Because natural language processing has come such a long way, FinTech businesses are also using chatbots to help customers. Modern robots, on the other hand, can do more than just answer basic customer questions. They can also give more personalized and useful financial advice.
#5: The stock market and machine learning
The huge number of trades creates a huge amount of past data that can be used to learn anything. On the other hand, historical data is only used to make forecasts.
ML algorithms look at real-time data sources, like news and trade results, to find trends that explain how the stock market works. Then it’s up to the traders to choose which machine learning algorithms to use in their trading methods and which behavioral pattern to use.
Do you know what MLOps are? Check out our article to learn what it is and how it can help software developers.
#6: Using machine learning to automate loans
Machine learning systems can work with more types of data than just FICO scores and income information. These kinds of uses of machine learning in finance have given lenders access to new types of data. For instance, a correct risk score is now based on a lot of different factors, such as social accounts, phone companies, utilities, rent payments, and even health checkup records.
To get a good risk score, algorithms match a lot of data points with information from thousands of customers. If the risk score drops below the lender’s cutoff, the loan will be given immediately.
Unlike human credit assessors, machine learning algorithms can look at borrowers without any bias or feeling. The Harvard Business Review says that when financial companies make models, they can get rid of racial, gender, and other biases that might make loans less fair.
#7: Using machine learning to look at facts and make decisions
No matter how much data FinTech has, machine learning gives them well-thought-out information they can use to make decisions in real time, which saves them time and money. But it also makes it easier to identify market trends more quickly and more accurately. With the help of these predictive analytics, FinTech businesses can stay ahead of the curve by coming up with new, future-proof solutions that can adapt to changing customer needs and market trends.
Does the bank you work for need to use machine learning?
In the next few years, as businesses change and new models come out, there will be a race to better computers. This will happen at the same time as machine learning technology.
With easier-to-use machine learning tools, a wider range of algorithms, and more powerful computers, the number of connections between machine learning and FinTech will only grow. It’s time to keep up with this trend.
And since FinTech machine learning is still a new technology, there are a lot of ways that finance and technology can work together to make the future much better for customers.
Are you planning to work on your next FinTech project? Check out our services for developing and advising on financial software.
Conclusion
The use of machine learning in FinTech is a game-changer that is promoting new ideas and better performance in many financial tasks. If you look at these seven use cases—from credit scores and robo-advisors to predictive analytics and fraud detection—you can see just how useful machine learning can be in the financial world. Machine learning is changing the way we use financial services because it can help us make better decisions, give customers better experiences, and handle risks more effectively. As FinTech keeps changing, adding machine learning technologies should make financial solutions smarter, more personalized, and safer, which will help both companies and consumers in the long run. The power of machine learning is becoming more and more connected to the future of FinTech. This combination is going to change the way we think about money.
Appic Softwares machine learning business is the best place to start as traditional finance goes digital. When the first FinTech tools came out, we saw history being made and used their power. We’ve become experts in the field and learned how to solve any financial problem, come up with only legal strategies, and create solutions that serve a purpose.
So, what are you waiting for?