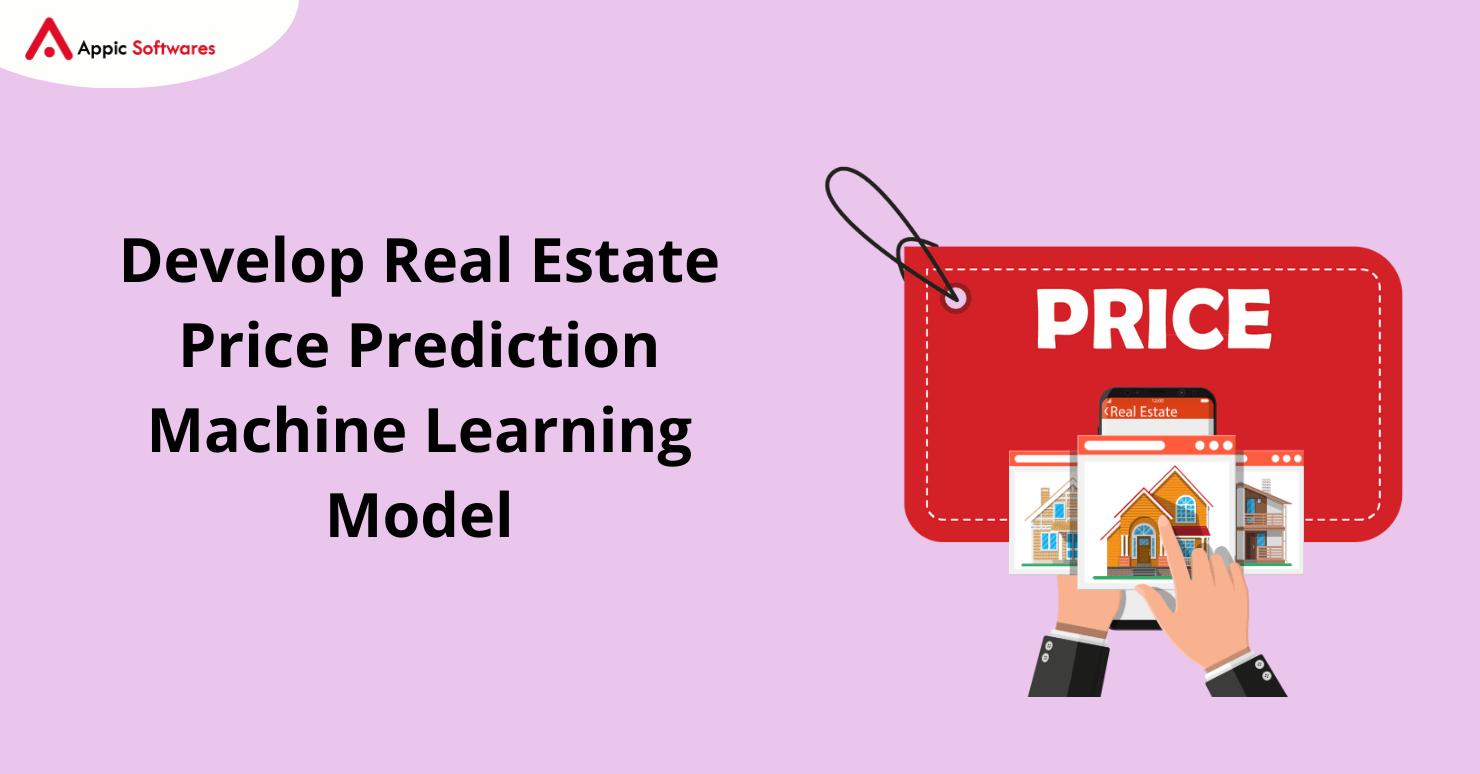
Do you know, the real estate market project is expected to reach $731.59 billion by 2028? Buying or selling a house is a big decision. You want to know what a fair price is, but figuring that out can take a lot of time and effort. What if there was a tool to help you guess a house’s price quickly and accurately? That’s where a real estate price prediction machine learning model comes in. This tool uses data and smart math to predict property prices, making life easier for buyers, sellers, and real estate agents. In this article, we’ll talk about the benefits of this model, how to use it, the steps to build one, and what it might cost. Let’s get started!
What Are The Benefits Of Real Estate Price Prediction Machine Learning Model?
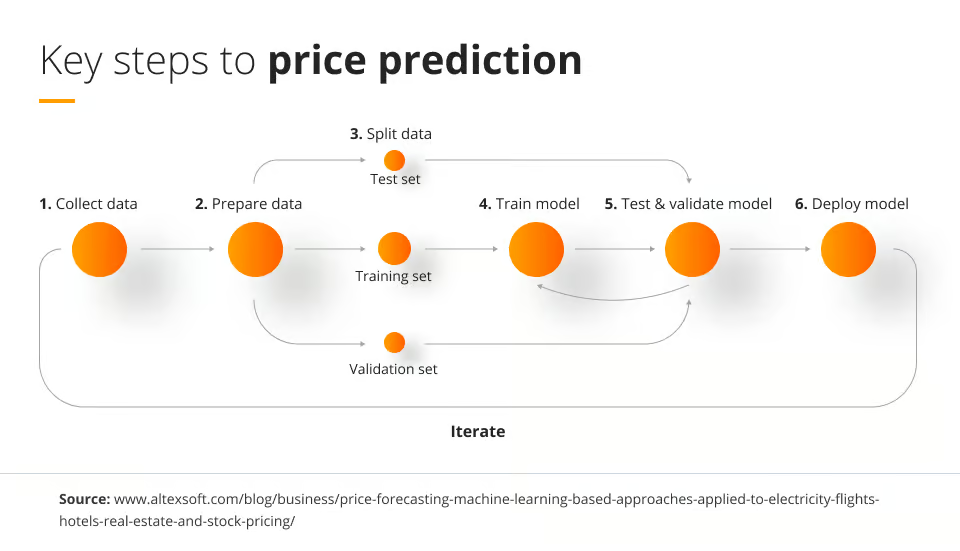
Source: https://www.future-processing.com/blog/the-importance-of-price-prediction/
The benefits of a real estate price prediction machine learning model stretch from improved investment choices to a better understanding of market shifts. When you work with machine learning and real estate, you use a data-driven method to find patterns in market data. The method supports decision-making and reduces the chances of mistakes. Many investors and property experts now rely on these models to help them plan for the future.
-
Improved Market Trends Analysis
A key reward is a better view of market trends. The model gathers many details about property sales, pricing changes, and location data. With these numbers, the model shows shifts in the market over time. Real estate price prediction machine learning users see patterns that help them decide when to buy or sell a property.
-
Enhanced Investment Planning
This model gives investors a stronger basis for planning. It works by reviewing a large range of data to point out patterns. The outcome is a forecast that many investors trust. Investors who use real estate price prediction machine learning models often plan their budgets with more confidence. They also consider advice from AI and machine learning in real estate investment to shape their long-term strategies.
-
Better Risk Management
Using a price prediction model helps to cut down on unexpected risks. Investors benefit when the model shows how past data relates to current prices. Many use real estate price prediction machine learning to spot signs of trouble ahead of time. With the help of machine learning real estate investing, buyers and sellers make choices that reduce losses. The model aids in finding cost changes that may affect overall investment returns. Plus, AI Agents In Real Estate can take this further by automating risk checks for you.
-
Faster Data Processing
A significant benefit of the model is that it handles large amounts of data quickly. It sorts through thousands of records about sales, prices, and locations. This speed gives investors the chance to respond to market changes in time. When using real estate price prediction machine learning, users experience a boost in the speed of analysis. Fast processing pairs well with insights from machine learning in commercial real estate to provide a rapid look at market conditions.
How To Use Real Estate Price Prediction Machine Learning Model?
The next step is to see how you can use a real estate price prediction machine learning model. Users follow a series of steps to get the most from the model. This part of the article covers data gathering, model training, and testing. We include methods and steps that use ideas from deep-learning real estate and machine-learning commercial real estate.
-
Input Data Preparation
Before you start, gather all the data you need. Information about past property sales, market trends, and economic factors helps shape the model. The process starts with collecting records from trusted sources. A good practice is to use both public data and private databases. When you prepare the data, include factors that many real estate price prediction machine learning models need. This step also uses techniques from machine learning for real estate to ensure the data fits the model.
-
Feature Selection Process
Next, choose the important details in your data. Not every piece of information adds value. Pick the features that have a direct impact on property prices. These features may include location, square footage, and the number of rooms. Experts in real estate price prediction machine learning stress the importance of picking the right attributes. Users also take advice from machine learning and real estate to decide which features to use in their model.
-
Algorithm Choice and Training
With the data ready, select the right algorithm to work on it. Many options exist, such as regression analysis and decision trees. Each method works differently based on the kind of data you have. The algorithm will train on the data and start to see the patterns. With real estate price prediction machine learning, users rely on algorithms that many say work best for property markets. This method aligns with insights from machine learning in real estate to build a reliable prediction model. And thanks to AI In real estate, this process is becoming a must-know skill for pros.
-
Model Testing and Refinement
After training the model, it is important to test its predictions. Compare the model’s forecast with actual market trends to check its accuracy. You may need to adjust some settings to get a better result. Testing helps ensure that the real estate price prediction machine learning model gives results that match the market. Users who follow this advice use tips from deep-learning real estate to make the model work well over time.
Easy Steps To Develop A Real Estate Price Prediction Machine Learning Model
Building your own real estate price prediction machine learning model might seem hard at first, but it can be done with a series of well-defined steps. Each step adds a building block to your model. The process uses ideas from machine learning in real estate investing and machine learning in commercial real estate. Let us look at the process in five easy steps.
Step 1: Data Collection
Start with gathering all the numbers you need. Look for past sales, price history, and factors like location and size. Data comes from many sources, including online records and public reports. The task is to amass enough information so that your real estate price prediction machine-learning model can see trends over time. Use advice from machine learning for real estate to know what data matters most. Collecting a rich set of numbers is the first part of a working model.
Step 2: Data Cleaning
Next, fix the data so it works well for your model. Remove any mistakes or duplicate numbers from the dataset. A clean set of numbers helps the model understand the true trends in the market. This step makes your real estate price prediction machine-learning model more reliable. Use tips from machine learning and real estate to spot errors and fix them. The better the data, the better the model will work.
Step 3: Model Building
Now, build the model with the data you prepared. Choose an algorithm that fits the type of data you have. Many opt for regression or decision tree methods in real estate price prediction machine learning. The algorithm trains by finding connections between the numbers in your data. Experts use techniques from machine learning commercial real estate to build models that suit the property market. You work on the design until the model starts to predict prices well.
Step 4: Model Evaluation
After you build the model, you must check how it performs. Test the model with new data that was not used in training. See if it makes good guesses about property prices. This check shows if the model has learned the trends in the market. Users who work on real estate price prediction machine learning models follow this step to spot mistakes. Tips from ai and machine learning in real estate investment can guide you on how to adjust the model if needed.
Step 5: Deployment and Monitoring
Finally, put your model to work. Set it up so it can predict prices for real homes. The real estate software development process comes in here; it’s about making your model easy to use through apps or websites. The method of monitoring ensures the model keeps pace with market changes.
Users who value machine learning real estate investing techniques find that ongoing checks make the tool a good helper in the property market. If you’re not a tech pro, a real estate app development company like Appic Softwares might help get it live.
How Much Does It Cost To Develop a Real Estate Price Prediction Machine Learning Model?
Here is a tabular representation of developing a real estate price prediction machine learning model:
Project Phase | Basic Model ($10K – $25K) | Intermediate Model ($25K – $50K) | Advanced Model ($50K – $80K) |
Project Planning & Strategy | $2,000 – $4,000 | $4,000 – $7,000 | $7,000 – $10,000 |
Data Acquisition & Processing | $3,000 – $6,000 | $6,000 – $12,000 | $12,000 – $18,000 |
Feature Selection & Engineering | $2,000 – $5,000 | $5,000 – $10,000 | $10,000 – $15,000 |
Model Design & Training | $3,000 – $7,000 | $7,000 – $15,000 | $15,000 – $25,000 |
System Deployment & Integration | $2,000 – $4,000 | $4,000 – $8,000 | $8,000 – $12,000 |
Performance Testing & Optimization | $2,000 – $3,000 | $3,000 – $6,000 | $6,000 – $10,000 |
Ongoing Support & Maintenance (Annual) | $1,000 – $2,000 | $2,000 – $5,000 | $5,000 – $10,000 |
Total Estimated Cost | $10K – $25K | $25K – $50K | $50K – $80K |
When you build a real estate price prediction machine learning model, you might wonder about the expenses involved. Costs depend on many factors, such as data size, the complexity of the model, and the resources needed for training. Some projects use open-source tools and free datasets, while others may require investment in advanced software. Investors and developers who use machine learning for real estate often plan their budgets based on the scope of the work.
· Factors Influencing Cost
Many parts affect the cost of a prediction model. One main factor is data. If you need vast amounts of updated numbers, you might pay more. The work on real estate price prediction machine learning also needs computers that can handle large jobs. Software expenses, storage, and cloud services add to the total cost. Experts in machine learning in commercial real estate say that planning ahead can help reduce extra costs. Every part of the project counts when you work on your model.
· Data Acquisition Costs
Data isn’t always free. You can snag some from public records, but the good stuff, like up-to-date sales or machine learning in commercial real estate details, might mean paying. Small sets could cost a few hundred bucks, while big, juicy ones might hit thousands. It’s like buying ingredients: the better they are, the more you pay.
· Development Time Costs
Making this model takes time. If you’re doing it, it’s your hours on the line. Hiring a pro? They might charge $50 to $200 an hour, depending on their skills. A quick model could take weeks, but a big one might need months. And if you want it on your phone, custom mobile software development adds more to the bill.
· Computing Resource Costs
Training needs power. A home computer works for tiny jobs, but big data means renting cloud space from places like Amazon. That can run from a few dollars to hundreds, based on how much you use. Real estate app development cost works the same way, power isn’t cheap when you scale up.
· Maintenance Costs
Once it’s live, you’ve got to keep it running. New data and updates cost money, think more data fees and time to refresh it. It’s not as much as building it, but it’s ongoing. Like a car, it needs regular care to stay sharp.
Budget Planning Tips
When you plan the budget, think about both current and future needs. Estimate the work hours needed for data collection, model building, and testing. Look at the costs for cloud services if you need them for training. Many who work with real estate price prediction machine learning suggest starting small and growing the model as you see success.
Advice from AI and machine learning in real estate investment often includes checking various vendors and options to keep the cost low. A planned budget helps in keeping the project on track.
Final Thoughts
A real estate price prediction machine learning model helps many people in the property market. It gives a strong view of market trends and supports investors in making smart decisions. From collecting data to testing the model, every step adds value to your work. This guide shows that using machine learning and real estate ideas makes the process more organized. When you study how deep learning real estate works, you see that accurate forecasts can boost property investments.
Ready to bring your software ideas to life? Contact Appic Softwares today for innovative, tailored solutions that drive success
FAQs
1. What is a Real Estate Price Prediction Machine Learning Model?
A Real Estate Price Prediction Machine Learning Model is an AI-driven system that analyzes various factors such as location, property size, market trends, and historical data to predict real estate prices. By leveraging machine learning algorithms, it helps investors, realtors, and buyers make informed decisions based on accurate pricing forecasts.
2. How does a Real Estate Price Prediction Machine Learning Model work?
The model collects data from multiple sources, including real estate listings, economic indicators, and demographic trends. It then processes this data using machine learning techniques like regression models, neural networks, or decision trees to identify patterns. Based on these patterns, the model predicts property prices by assessing how different features influence pricing over time.
3. What are the key benefits of using a Real Estate Price Prediction Machine Learning Model?
This model offers several advantages, such as improved pricing accuracy, reduced human bias, and enhanced decision-making. It enables real estate professionals to forecast market trends, helps buyers understand fair property valuations, and supports financial institutions in risk assessment. Additionally, it minimizes the time and effort required for manual price evaluations.
4. What types of data are required to develop this model?
To develop a Real Estate Price Prediction Machine Learning Model, diverse datasets are necessary. These include property attributes (size, age, type), location-based factors (proximity to schools, hospitals, transportation), economic indicators (interest rates, inflation), and historical real estate transactions. High-quality and up-to-date data significantly improve the model’s accuracy.
5. Which machine learning algorithms are best for predicting real estate prices?
Several algorithms can be used for real estate price prediction, depending on the complexity of the dataset. Common approaches include Linear Regression, Random Forest, Gradient Boosting (XGBoost), Neural Networks, and Support Vector Machines (SVM). Each algorithm has its strengths, and often, a combination of models (ensemble learning) is used to enhance predictive performance.
6. How can I improve the accuracy of a Real Estate Price Prediction Machine Learning Model?
Model accuracy depends on factors like data quality, feature engineering, and algorithm selection. To enhance performance, use high-quality datasets, clean and preprocess the data, select relevant features, and apply hyperparameter tuning. Incorporating external factors like economic trends and local development projects can further refine predictions.
7. How much time does it take to develop a real estate price prediction model?
The development timeline varies based on data availability, complexity, and model requirements. A basic model may take a few weeks, while a more advanced, fine-tuned version with real-time data integration can take several months. Continuous model training and updates are essential for maintaining accuracy over time.
8. Can this model predict future real estate prices with complete accuracy?
No model can predict real estate prices with 100% accuracy due to market fluctuations, unexpected events, and economic changes. However, a well-trained Real Estate Price Prediction Machine Learning Model can provide highly reliable estimates by identifying trends and analyzing historical data. Continuous updates and improvements help maintain precision.
9. What are the challenges in developing a real estate price prediction model?
Some of the primary challenges include data collection issues, feature selection, model overfitting, and handling market volatility. Ensuring data privacy and security is also a concern, as real estate pricing involves sensitive financial information. Addressing these challenges requires robust data preprocessing, feature engineering, and regular model retraining.
10. How can businesses and realtors benefit from this model?
Real estate agencies, investors, and homebuyers can use a Real Estate Price Prediction Machine Learning Model to make data-driven decisions. It helps in property valuation, risk assessment, and identifying investment opportunities. Businesses can integrate these models into real estate platforms to provide users with automated price insights, enhancing customer engagement and sales efficiency.