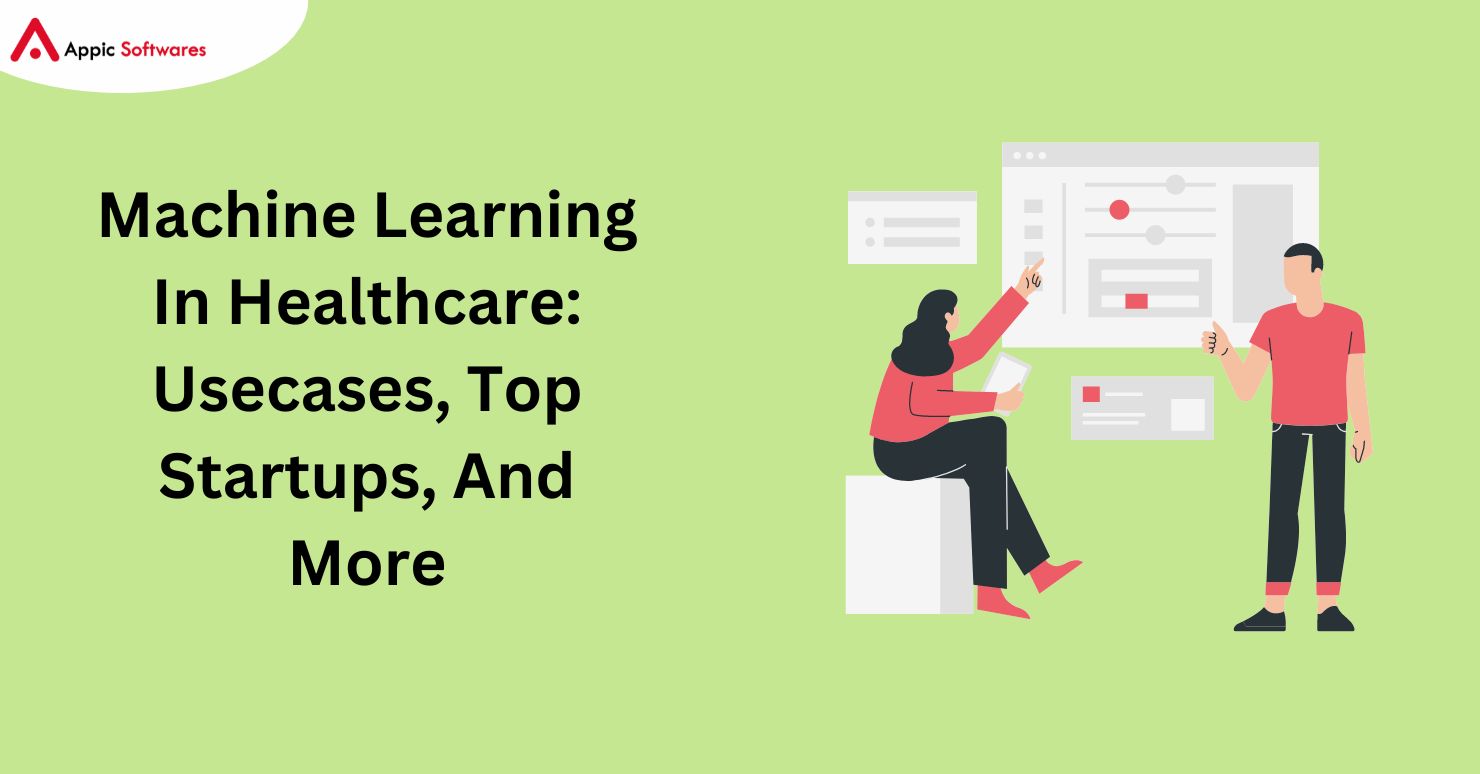
Artificial intelligence and machine learning are advancing clinical operations, drug discovery and distribution, and data administration in healthcare. The impact of deep learning in healthcare is also becoming increasingly evident as more machine learning applications in healthcare emerge.
According to Statista, the global healthcare AI market was valued at over $11 billion in 2021 and is projected to skyrocket to $187 billion by 2030, growing at a compound annual growth rate (CAGR) of 37% between 2022 and 2030.
But what factors are contributing to this rapid expansion of AI and ML in healthcare? What are the practical applications and benefits of machine learning in the medical field? And what implications does adopting these technologies have for healthcare organizations?
Let’s explore the answers to these questions in the blog below, including insights into companies using AI in healthcare and examples of machine learning in healthcare.
What Is Machine Learning In Healthcare?
Machine Learning in Healthcare refers to the application of machine learning algorithms and models to solve complex problems within the healthcare domain. It involves using data-driven approaches to enhance decision-making, improve patient outcomes, and optimize various aspects of healthcare delivery. Machine learning in healthcare leverages vast amounts of data—from electronic health records (EHRs) and medical imaging to genomics and wearable devices—to provide insights, predictions, and automation that aid healthcare professionals in various tasks.
Key Aspects Of Machine Learning In Healthcare
1. Data-Driven Decision Making
Machine learning models analyze large datasets to identify patterns and trends that might not be immediately apparent to human clinicians. These insights can help in making informed decisions about patient care, treatment plans, and resource allocation.
2. Predictive Analytics
Machine learning can predict patient outcomes, disease progression, and potential complications by analyzing historical data. This allows for early intervention and personalized treatment plans, improving overall patient care.
3. Automation Of Routine Tasks
Machine learning algorithms can automate repetitive and time-consuming tasks, such as medical image analysis, administrative paperwork, and even some aspects of patient monitoring. This frees up healthcare professionals to focus on more complex and critical tasks.
4. Personalized Medicine
By analyzing individual patient data, such as genetic information, lifestyle factors, and medical history, machine learning can help tailor treatments specifically to each patient’s unique needs. This approach, known as personalized medicine, aims to optimize the effectiveness of treatments and reduce side effects.
5. Enhancing Diagnostics
The use of machine learning to enhance the precision of diagnoses is on the rise. For example, it can analyze medical images to detect conditions such as tumors, fractures, or infections with high precision. This assists doctors in making more accurate diagnoses and planning appropriate treatments.
6. Operational Efficiency
Machine learning can optimize hospital operations, including resource management, patient flow, and scheduling. By predicting patient admissions and discharges, healthcare facilities can better manage their resources, leading to cost savings and improved patient care.
Machine Learning In Healthcare Use Cases
Personalized Treatment
One of the most prominent uses of machine learning in healthcare is the development of personalized treatment plans. By analyzing a patient’s medical history, symptoms, and test results, machine learning algorithms enable healthcare providers to tailor treatments that are specifically designed for each patient. This approach ensures that patients receive the most effective therapies from the outset, potentially improving outcomes and reducing the need for trial-and-error in treatment.
Moreover, machine learning can assist doctors in determining whether a patient is ready for changes in their medication regimen. This helps in optimizing the treatment process, ensuring that patients receive the correct dosage and medication adjustments at the right time.
Fraud Detection And Prevention
Healthcare fraud is a significant issue, resulting in billions of dollars in financial losses annually. According to the National Health Care Anti-Fraud Association (NHCAA), healthcare fraud accounts for approximately 3% to 10% of total healthcare expenditures, translating to around $300 billion in losses each year.
Machine learning plays a critical role in combating healthcare fraud by identifying invalid insurance claims before they are processed and paid. This technology speeds up the approval and payment process for legitimate claims while preventing the theft of sensitive patient data. For instance, Harvard Pilgrim Health has implemented AI and machine learning systems to detect and prevent fraudulent claims, enhancing the security and efficiency of its operations.
Early Disease Detection
It is crucial for determining the most effective treatment plans and improving patient quality of life. Machine learning enhances the early detection of various conditions by comparing new patient data with historical data on similar ailments. This comparison allows doctors to make informed decisions and design appropriate treatment strategies.
Machine learning algorithms can analyze vast amounts of data to identify patterns and correlations that may not be immediately apparent to human clinicians. This capability enables healthcare providers to detect diseases in their early stages, often before symptoms become severe, thereby improving patient prognosis and outcomes.
Robot-Assisted Surgery
Machine learning has also made significant strides in the field of robotic surgery. ML-powered surgical robots have transformed surgical procedures by increasing precision and reducing the risk of complications such as blood loss and infection. These systems can perform complex surgeries with greater accuracy and efficiency, leading to faster recovery times and reduced post-operative pain for patients.
A notable example is the surgical robot used at Maastricht University Medical Center, which employs machine learning to assist in suturing tiny blood vessels, some of which are as thin as 0.03 millimeters. This level of precision is unmatched by human surgeons and represents a significant advancement in surgical care.
Error Analysis In Prescriptions
Prescription errors are a major concern in healthcare, with thousands of patients dying each year due to incorrect medications or dosages. In the United States alone, it is estimated that between 7,000 and 9,000 patients die annually due to prescription errors.
Machine learning can help reduce these errors by analyzing historical electronic health record (EHR) data and flagging prescriptions that deviate from established patterns. This allows doctors to review and adjust prescriptions before they are administered, reducing the risk of adverse drug interactions and overdoses.
For instance, Brigham and Women’s Hospital has implemented a machine learning system that identified over 10,000 potential prescription errors in a single year, of which 79% were clinically significant. This system not only improved patient safety but also saved the hospital $1.3 million in healthcare costs.
Assistance In Clinical Research And Trials
Clinical trials are essential for testing the safety and efficacy of new medical treatments, but they are often time-consuming and expensive. Machine learning can streamline this process by selecting optimal trial participants, analyzing ongoing trial data, and reducing data-related errors.
By leveraging machine learning, researchers can expedite the development of new treatments, bringing them to market more quickly. This is particularly important in situations where rapid responses are needed, such as during the COVID-19 pandemic.
Drug Discovery And Development
Machine learning is also revolutionizing the drug discovery and development process. By analyzing molecular structures and vast databases of chemical compounds, machine learning algorithms can identify potential new drugs more quickly and cost-effectively than traditional methods.
A prime example is Atomwise, a pharmaceutical company that uses deep learning technology to repurpose existing drugs for new therapeutic applications. In 2015, Atomwise successfully identified two drugs that could potentially treat the Ebola virus in just one day—a task that would have taken years using conventional methods.
Automating Image Diagnosis
Machine learning is transforming the field of medical imaging by enabling the automated analysis of MRI and radiology scans. These algorithms can identify abnormalities such as tumors, infections, and other medical conditions with high accuracy, assisting doctors in making more accurate diagnoses.
One example is the tool used at UVA University Hospital, which employs machine learning to analyze biopsy images in pediatric patients. This tool can differentiate between celiac disease and environmental enteropathy with the same reliability as human doctors, improving diagnostic accuracy and patient outcomes.
Top 4 Machine Learning In Healthcare Startups
Rad AI
It is at the forefront of integrating AI and machine learning in healthcare, specifically within the radiology sector. Founded in 2018 by Dr. Jeff Chang and Doktor Gurson, this USA-based company has raised $33 million in funding. Rad AI Omni is an innovative tool that leverages machine learning to automatically generate radiology impressions from dictated findings, customized to each radiologist’s style. This medical machine learning application significantly reduces the time radiologists spend on report generation, helping to alleviate burnout and improve patient care quality. By streamlining radiology workflows, Rad AI exemplifies how machine learning in healthcare can enhance efficiency and outcomes.
BrainSight AI
BrainSight AI is a pioneering startup from Bangalore, India, focusing on AI and machine learning applications in healthcare, particularly in neurological and psychiatric diagnostics. Dr. Rimjhim Agrawal and Laina Emmanuel founded the startup in 2019, which uses fMRI, sMRI, and digital phenotypes to enhance diagnosis and prognosis. This deep learning in healthcare technology helps neurosurgeons, psychiatrists, and neurologists make more accurate, data-driven judgments and gives patients a personalized digital treatment experience. BrainSight AI’s work demonstrates the critical role of machine learning in the medical field for advancing patient care.
Octopus.Health
Octopus.Health, a startup from Tel Aviv, Israel, is focused on AI and machine learning to enhance patient engagement and population health management. Yoav Ariav founded the business in 2018, and it has raised $5.9 million to create a platform that uses machine learning algorithms to develop individualized healthcare plans. This system helps improve patient adherence to medication regimens and diagnostic testing, ensuring better clinical management and care quality. Octopus.Health is a prime example of how AI and ML in healthcare can be applied to improve patient outcomes through tailored, data-driven interventions.
VIEBEG
VIEBEG Technologies, headquartered in Nairobi, Kenya, utilizes machine learning to optimize the healthcare supply chain in underserved markets. Founded in 2018 by Tobias Reiter and Alex Musyoka, VIEBEG has raised $1.9 million to develop a platform that ensures hospitals have the necessary medical supplies when they need them. This machine learning in healthcare solution de-risks and streamlines procurement processes, making it easier for healthcare providers to maintain inventory in challenging environments. VIEBEG’s platform underscores the potential of AI and machine learning in improving healthcare infrastructure and access to essential supplies.
Challenges Of Adopting Machine Learning In Healthcare
Patient Safety Concerns
One of the primary challenges of implementing ML in healthcare is ensuring patient safety. Machine learning algorithms are dependent on the quality of data they are trained on. Unfortunately, there are frequently gaps, inaccuracies, and inconsistencies in medical data, which can cause ML models to make incorrect predictions or decisions. Inaccurate data can result in misdiagnoses, inappropriate treatments, or even endanger patients’ lives. Therefore, healthcare organizations must ensure the reliability and accuracy of the data fed into ML models to avoid adverse outcomes.
Bias In Datasets
Biases in the datasets used to train ML models pose another significant challenge. If training data is biased, ML algorithms might reinforce these biases, skewing results. Biased healthcare models can misdiagnose or undertreat specific patient populations. Robust data governance and ML model training on diverse, representative datasets are needed to eliminate prejudice and promote fair healthcare.
Privacy And Security Issues
The implementation of ML in healthcare also raises concerns about data privacy and security. Healthcare data often contains sensitive and confidential information, making it a prime target for cyber threats. Healthcare firms must secure patient data because ML models demand enormous data for training. To prevent data breaches, this includes using modern encryption, secure data storage, and rigorous access controls.
The Future Of Machine Learning In Healthcare
Despite these challenges, the future of ML in healthcare is bright. ML can solve problems and identify trends to improve patient outcomes and healthcare operations, revolutionizing healthcare. ML can analyze vast amounts of data, personalize treatment plans, predict risks, and enhance the accuracy of diagnoses. For instance, ML can provide early warnings for conditions like seizures or sepsis, which require intensive analysis of complex datasets.
Looking ahead, the integration of ML with emerging technologies like nanotechnology could further enhance healthcare delivery. ML could enable “virtual biopsies” and accelerate radiomics, which uses images to assess disease features. Additionally, ML-powered technologies could assist doctors during surgeries, minimizing risks and improving patient outcomes.
Conclusion
Machine learning is transforming healthcare by improving patient care, operational efficiency, and medical sector innovation. Machine learning is transforming healthcare with individualized treatment plans, early disease identification, diagnostic automation, and medication development. The continuing acceptance and refining of these technologies will help solve modern healthcare problems and improve patient outcomes worldwide.
If you’re looking to integrate machine learning into your healthcare solutions, partnering with a trusted development company is essential. Appic Softwares stands out as the best for machine learning development services, offering cutting-edge solutions tailored to your specific needs. With expertise in the latest technologies and a commitment to delivering high-quality, innovative solutions, Appic Softwares can help you harness the full potential of machine learning to transform your healthcare operations.
Contact us today to learn how we can assist you in your journey toward a smarter, more efficient healthcare system.