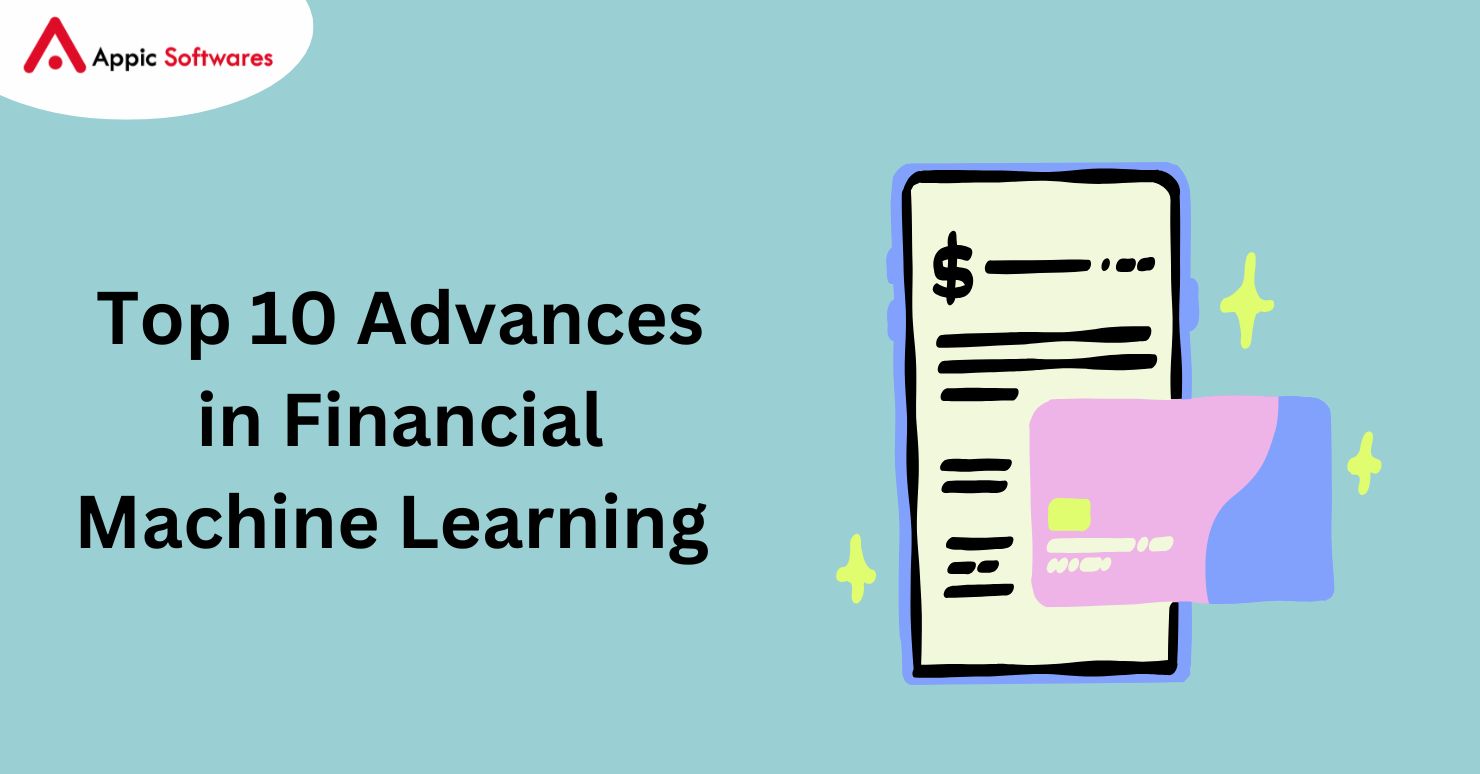
With its previously unheard-of improvements in security, efficiency, and decision-making, machine learning (ML) is transforming the banking industry. Financial institutions may be able to maximize investments, detect fraud in real time, and forecast market trends more precisely as they implement AI-driven solutions.
ML is changing contemporary finance, from deep learning models that improve financial forecasting to blockchain integration that increases transaction transparency. This article examines the top ten advances in financial machine learning for 2025, emphasizing the most recent breakthroughs that are revolutionizing the sector. Whether you work in fintech, invest, or are just interested in technology, knowing these trends can help you keep on top of
What Is Machine Learning?
Every person must have heard the term AI; however, very few know its branches. Machine learning is an extension of AI that permits computers to learn from data and enhance their forecasting outcomes, independent of programming. This technology underpins many advances in financial machine learning, significantly impacting finance. To improve their statistical and predictive skills, 70% of financial services firms employ machine learning. There is no longer a need to get a credit card signal; algorithmic trading takes care of that too. Modern finance needs foresight, accurate assessments, and fraud detection, and that is precisely what machine learning has to offer.
The transformation and advanced technology offered by machine learning in finance have boosted real-time data processing. As a result, accurate decisions can be made, which leads to greater efficiency. The competition in the market has made it mandatory for businesses to meet the expectations of their clients. Offering favorable experiences and optimizing investment strategies has become the norm, and efficiency since the dawn of machine learning is integral for financial institutions. The foundation of machine learning has enabled financial businesses, such as insurance and banks, to alleviate stress in areas. Offering favorable experiences and optimizing investment strategies has become the norm, and efficiency has become integral for financial institutions since the dawn of machine learning.
How does machine learning work?
Patterns in data are recognized by machines and models, and based on these wide datasets, predictions are rendered. The model types can be grouped into three branches:
– Supervised Learning: Contains labeled data where algorithms predict outputs based on pre-trained information. Credit scoring models and fraud detection systems are two common examples of this.
– Unsupervised Learning: Involves looking for hidden patterns without having set labels. It is employed in customer segmentation and anomaly detection.
– Reinforcement Learning: This learning method teaches models via trial-and-error. Commonly used in algorithmic trading and portfolio management, models are optimized over time.
These approaches fuel the advance in financial machine learning that drives innovation across the industry.
How Machine Learning Will Improve Services in Fintech?
Fintech is one of the industries that is changing at breakneck speed. Machine learning is at the center of the innovation. While companies are focused on building Fintech MVPs, lowering the cost, and upgrading the tech stack for FinTech, ML continues to optimize business services. These are some of the most important Directions of ML application in business process automation:
1. Fraud Detection and Prevention
Fraud detection in banks and other financial institutions is usually carried out using outdated static techniques with predetermined rules. Modern cyber threats compared to these static approaches render them utterly useless. The solutions powered by ML perform automated detection on transactions in real-time, tracking their movements, and marking unusual activities and attempts at fraud more accurately. Financial organizations are capable of preventing losses by using sophisticated algorithms designed for anomaly detection.
2. Improved Customer Experience
Personalized financial advice is now automated through AI chatbots and assistants. Customers are no longer switched from one customer service representative to another and response times have been greatly improved. Machine learning allows to customize of investment and banking services to intricate details of the particular user. AI assistants operate around the clock, which enhances the convenience and satisfaction of customers with digital banking services.
3. Risk Assessment and Credit Scoring
Many traditional credit scoring models fail to cover all aspects of the applicant’s financial behavior and may misinterpret information. Machine Learning (ML) algorithms construct a model of creditworthiness by analyzing a wider range of data including spending and social behavior as well as other forms of data. This step enables greater financial inclusivity by allowing lenders to consider borrowers who do not have traditional credit records.
4. Automated Trading and Portfolio Optimization
The use of machine learning has led to increased popularity of algorithmic trading. Successful investment requires accurate prediction, which integrates analysis of market signals, historical price movements, and macroeconomic indicators, all of which can be conducted using advanced ML models. Investors are now able to make decisions from automated trading algorithms that adjust according to real-time market changes, maximizing investment returns through optimized portfolios.
5. Regulatory Compliance and Reporting
The financial sector is subject to stringent regulations and oversight. Automated compliance ML tools assist in monitoring compliance risks by flagging non-compliant transactions and verifying compliance with legal and ethical boundaries set. AI-driven RegTech solutions enable financial institutions to satisfy compliance requirements to develop fintech MVP while still maintaining operational efficiency.
6. Predictive Analytics for Financial Planning
Predictive analytics help get valuable insights that can help construct and achieve desired long-term goals through investments. As technology progresses, finance companies will shift towards greater use of AI agents in finance and marvels of machine sophistication.
ML helps banks and financial service providers forecast customer requirements, and optimize loan offerings, and investment opportunities based on historical and behavioral data.
Top 10 Advances in Financial Machine Learning
Here are the ten most significant advances in financial machine learning set to redefine finance in 2025:
1. Deep Learning for Financial Forecasting
Deep learning, using recurrent neural networks (RNNs) and transformers, powers advanced financial machine learning for precise market predictions. This helps with time series data which allows bank and financial analysts to make more precise market predictions. Investment companies are more strategic with their funds and risk assessment thanks to deep learning. Not to mention, using lossy-less compression techniques in Generative Adversarial Networks (GANs) helps artificially construct realistic financial scenarios to stress test.
2. Quantum Machine Learning in Finance
It looks ready to shake up financial services by efficiently resolving computation challenges. Quantum ML can analyze vast datasets in record time, drastically improving portfolio optimization, risk management, and derivative pricing. Its advanced machine learning in finance capabilities require substantial cost due to the investment in cutting-edge research and infrastructure, promising enhanced security via quantum encryption.
3. Explainable AI (XAI) for Transparency
Financial institutions are widely adopting Explainable AI (XAI) to provide better transparency and insight to their ML frameworks. Financial institutions are now bound by robust legislative frameworks that require them to provide reasoning behind AI-derived decisions, making it a non-negotiable prerequisite. XAI allows ML algorithms a degree of autonomy while ensuring that the generated outputs are justifiable, instilling confidence among regulators, investors, and clients. This is very crucial for sensitive transactions like loan approvals, insurance underwriting, and credit risk evaluation. This promotes trust among stakeholders, a critical advance in financial machine learning.
4. Automated Risk Management Systems
Machine learning boosts risk management with the automation of detection and mitigation of financial risks. Real-time risk assessments are available using ML-equipped systems that analyze historical data alongside current market conditions. This enables institutions to proactively manage volatility, liquidity, and credit exposure risks. Advanced stress testing models put various financial crises through an institution to analyze their resilience.
5. Personal Finance Assistants with AI Capacity
Finance is one of the domains benefitting from the automation and AI boom through personalized finance apps. AI-powered assistants go a step further in dealing with a user’s unique behavior and shift trend patterns. Using voice commands and simplified language instructions, AI-powered assistants can help them identify spending habits and areas for savings, even providing investment recommendations tailored to their preferences. These innovations are further enhancing the financial literacy of people, helping them make informed choices regarding spending, savings, and investments.
6. Analyzing Market Sentiment for Predictive Analytics
Analysis of sentiment involves the use of emotions conveyed in articles, social media, and company announcements through network computing to evaluate the overarching text. Sentimental analysis has become an important tool for traders who want to know how the crowd feels about the share. Traders use sentiment analysis to gauge how positive or negative the important people feel about the given share and with the help of advanced tools, they are able to track economically significant social media posts, international relations, and news to make forecasts with greater certainty. These are also known as advanced sentiment analysis models. They are equipped with the ability to anticipate stock price movements more accurately.
7. Using Simulation Models to Train AI
The need to safeguard data is becoming of higher priority which brings synthetic data to train machine learning algorithms as a favorable alternative when looking at sensitive financial details. Financial institutions have started adopting the use of fictive data to train automatic fraud detection systems, test newly developed trading strategies, or enhance their predictive models without risking confidential information. Moreover, synthetic data provides a remedy for discriminatory assumptions in finance.
8. Reinforcement Learning for Algorithmic Trading
As a more sophisticated form of machine learning (ML), reinforcement learning (RL) enables trading algorithms to interact with the market. RL-based models modify their trading actions in real-time according to the outcomes achieved, strategically shifting portfolio allocation to increase returns and decrease losses. Such models are able to respond to different trading conditions and perform high-frequency trading more accurately than before.
9. Mashup of Blockchain and Machine Learning
In the context of finance, the combination of blockchain and machine learning (ML) technologies is enhancing security, transparency, and operational productivity. The decentralized, unchangeable record of transactions known as blockchain, along with ML’s data-driven capabilities, are changing many aspects of finance, considering everything from fraud detection to automated settlements.
One of the most remarkable advancements can be seen in smart contracts based on machine learning. These contracts execute agreements based on preset parameters without human intervention, cutting out the middlemen and enabling the safe and transparent execution of contracts. Also, fraud detection on the blockchain takes advantage of ML algorithms to study a large number of transactions in real-time, spotting deviations from the norm and flagging them before they lead to losses. Professionals can explore advances in financial machine learning books to stay updated on these transformative developments.
10. Advancements in Financial Machine Learning Books and Research
The area of financial machine learning is continuously changing with innovative research, new techniques, and newer publications. Breakthroughs in deep learning, reinforcement learning, and even natural language understanding are changing the standards of algorithmic trading and risk management.
Cutting-edge academic research is not limited to classrooms with many established institutions joining forces with fintech companies to improve ML applications for portfolio optimization, sentiment analysis, and even high-frequency trading systems. Staying up to date with all these changes allows finance professionals to fully appreciate what machine learning can do to redefine business value.
The Possibilities of Machine Learning Applications in the Financial Industry
The potential of advances in financial machine learning extends beyond current applications, fueling an industry-wide shift. It’s no surprise that as technology advances, financial institutions must also adapt to new technology for investment purposes. With the fintech app development companies the funding of it goes to fuel an entire industry, which directly aids in bringing optimized ML-driven solutions, and creates an environment of sustained success for these firms.
It’s safe to say that, ‘Financial machine learning’ is not some utopic vision, it stands as the foundation of every financial service today. The efficacy and profitability greatly increase with the adoption of artificial intelligence tools that improve multitasking, risk evaluation, and customer services, which maximize efficiency on any and every level. To put it simply, Businesses that are willing to proactively incorporate machine learning in their operations as the world around us continues turning will be the ones who thrive (if we get more specific, make sure you do this in an increasingly digitized economy).
To create a strong foundation along with other new possibilities for growth, organizations should also focus on integrating blockchain, motivating cutting-edge research, and focusing on ML-driven strategies. The potential integration and application of AI in finance will be significant in future development. Those who are prepared to embrace this transformation and lead the way in finance-infused machine-learning applications will undoubtedly lead the way.
Conclusion
As the world’s complexity and digital nature increase, financial organizations need to adopt machine learning to retain their market edge. The firms that will succeed are the ones that adopt ML tech and fintech early because it will boost operational efficiency and create trust and transparency in financial dealings.
Machine learning goes beyond technological evolution; it is an emerging revolution that will improve the finance world. Companies that adapt to new trends early on will have unparalleled growth opportunities while using the changing dynamics of finance to ensure effortless growth in the ever-changing finance world.
If you’re looking to develop an AI-powered fintech solution, Appic Softwares can help! With expertise in machine learning, blockchain integration, and AI-driven fintech development, we offer tailored solutions to elevate your financial services.
Contact us today to build the future of fintech with state-of-the-art AI technology!
FAQs
1. How is AI improving security in the banking sector?
AI enhances security through fraud detection, biometric authentication, and anomaly detection. Advances in Financial Machine Learning enable banks to identify suspicious activities in real time, reducing fraud risks.
2. What are the key benefits of AI-driven technologies in banking?
AI improves efficiency, decision-making, customer experience, and risk management. It automates processes, provides predictive insights, and offers personalized financial solutions.
3. How can Appic Softwares help in AI-powered fintech development?
Appic Softwares specializes in AI-driven fintech solutions, including machine learning algorithms, fraud prevention systems, and smart banking applications. Our expertise ensures secure and efficient financial software tailored to your needs.