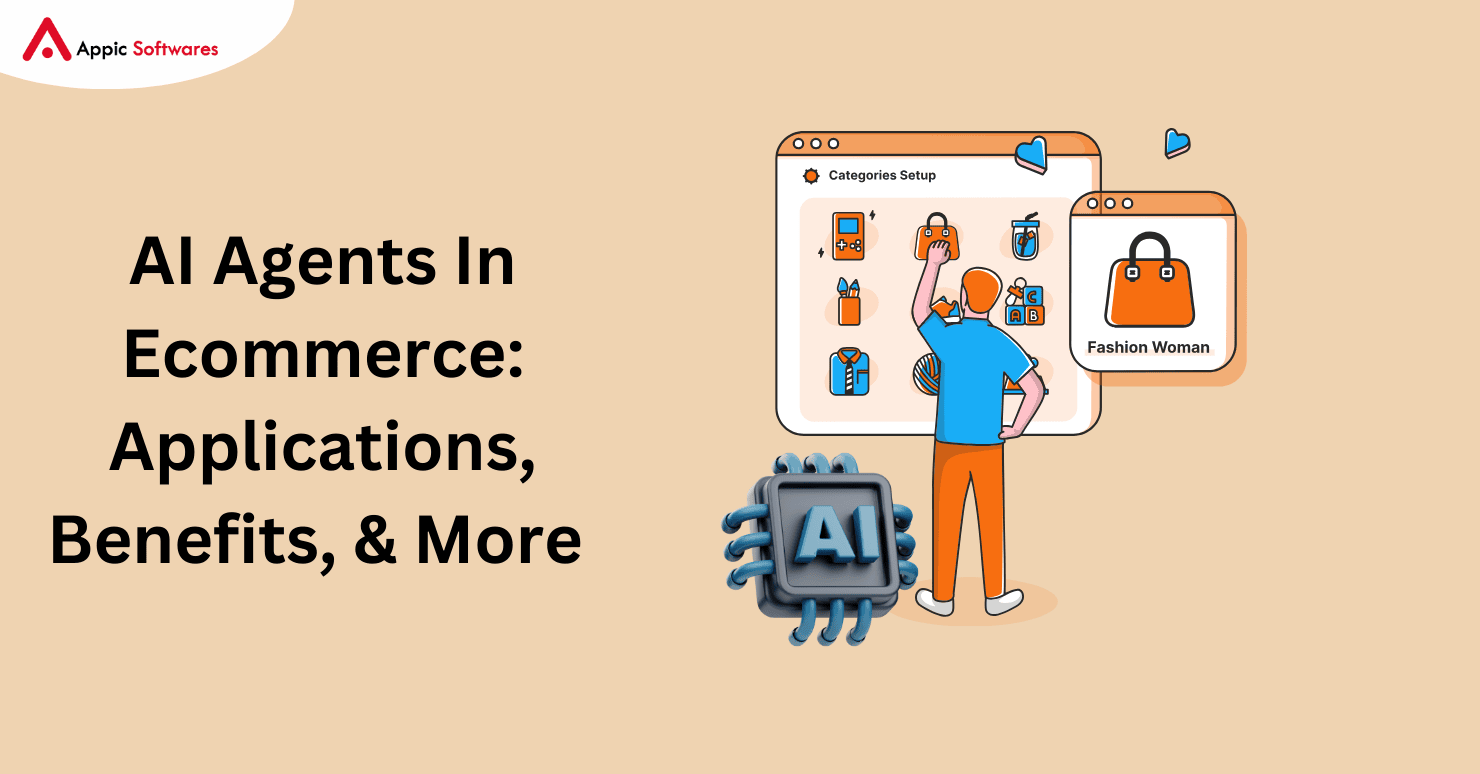
Do you know 90% of the large ecommerce companies have tried AI in their supply chain whereas 29% of them are planning to invest more to enhance it? That’s not it, AI is making considerable changes in the ecommerce industry, from customer support to inventory management, and more.
Through this blog, we will be informing you about the latest trends, use cases, and more about AI agents in ecommerce, from automated customer support to optimizing product pricing in such a way that it increases sales without affecting profit.
What Are AI Agents?
AI Agents are virtual assistants that are used to perform tasks by leveraging artificial intelligence. These are smart agents, developed to understand the occurrence of an event around an environment, interpret data, and make informed decisions accordingly.
The most advanced capability of AI Agents that differs from other AI models is that they learn and adapt to new changes on their own. Making it easier for AI agents to quickly upscale themselves.
Moreover, these AI agents can be combined to form a multi-agent system that performs various tasks simultaneously such as customer support, product price optimization, product recommendations, and more. As per research, 50% to 60% of shoppers have expressed their interest in using AI agents to purchase products like medicine, clothes, beauty items, and electronics.
AI Agents In Ecommerce Trends 2025
The AI agents in Ecommerce will not be limited to just customer support or operation management. These agents will play an important role in generating more sales, creating better visuals, and more in an ecommerce business. Here is how AI agents in Ecommerce will be shaping the eCommerce industry:
Better Product Visuals
AI Agents in Ecommerce will be responsible for developing product graphics that attract more users. They penetrate deep into the demographics, interests of people, and more to develop graphics that increase the chances of generating more sales.
AI will be playing different roles in creating graphics for ecommerce brands, such as deciding what product should be highlighted at a particular time, what should be its tagline, the background color, and more which helps the marketing team quickly get high-quality graphics in time.
AI Agents Will Enhance Brand Value
If trained well, the AI agents will understand how your brand communicates with customers. Be it with certain words, offers, or more, the AI agents will learn how you respond to your customers and will do the same on every platform where your customers are interacting with you.
This helps you deliver an omnichannel unified experience and scale up customer satisfaction.
Increase Loyalty And Foot Traffic
The AI agents will be acting as local store managers assisting and offering discounts/offers to customers so that they keep on purchasing from them. Moreover, AI agents will also be bringing back the dead profiles that no longer purchase from your store by providing them with personalized offers which increases the chances of more sales and scale up foot traffic.
Keep Humans In The Loop
With the help of Sentiment analysis, the AI agents will understand how serious the customer issue is and what steps should be taken to solve it. These AI agents are not chatbots that just copy-paste the content from the Knowledge base with no emotions.
Usecases Of AI Agents In Ecommerce
By leveraging Machine Learning, Natural learning processing, predictive analytics, and more the AI agents in Ecommerce will help you automate operations, scale up customer loyalty, increase revenue, and more. Let’s dive in know some of the most appropriate use cases of AI agents in Ecommerce:
Personalized Product Recommendation
By analyzing the customer browser history, previous purchases, and products in the cart, the AI agent will create a personalized list of products for every user making it easier for them to make a purchase.
With the help of ML Algorithms such as Collaborative Filtering, Content-Based Filtering, Hybrid Models, and more the AI agents create a list of complete personalized list. Amazon recently incorporated an AI agent that provides personalized product recommendations which helped them gain over 35% sales, just from AI product recommendations.
Virtual Shopping Assistant
AI Agents when integrated into your Ecommerce store help your customers to get info on the latest fashion trends. With the help of the previous purchase data, the virtual agent will suggest to users the clothes that they are looking for a particular function or event.
With the help of Dialogflow, Rasa, AWS Lex, and more you get a virtual agent that helps users decide on the perfect combination to wear. An example of a virtual agent that helps in customer support and fashion advice is Kik, installed on H&M’s site it offers fashion advice by analyzing user preferences and feedback.
AI-Powered Dynamic Pricing And Demand Forecasting
AI models analyze competitor pricing, demand trends, purchase behavior, and market conditions to dynamically adjust prices. These AI agents optimize profitability while maintaining competitiveness. By using price-optimizing algorithms such as Elasticity-based pricing, Competitive Price Tracking, and more your AI agent will change the price of the products based on the surge on your website.
Uber uses an AI-driven surge price algorithm which adapts rates based on demand spikes, maximizing revenue. This approach is a key aspect of dynamic pricing eCommerce machine learning, enabling businesses to adjust prices in real time to enhance sales and profitability.
AI-Driven Inventory And Supply Chain Optimization
With the help of OCR-based barcode scanning and defect detection in inventory, AI agents can be used to predict stock levels, forecast demand, automate reordering processes, and optimize warehouse logistics by analyzing large datasets.
Zara uses AI agents to manage inventory that predicts demand fluctuations and restocks accordingly, reducing stockouts and overstock issues.
AI Sentiment Analysis
AI agents analyze customer reviews, social media interactions, and feedback to extract sentiment insights. This helps brands understand customer perception and improve product offerings. Amazon and Netflix employ AI-based sentiment analysis to assess customer reviews and optimize content recommendations.
Types Of AI Agents In Ecommerce
Different types of AI agents serve various purposes, from simple rule-based systems to fully autonomous, self-learning models. Below is an in-depth look at the key types of AI agents used in e-commerce and their applications.
Reactive AI Agents
Reactive AI agents operate based on predefined rules and patterns set by AI developers. These AI agents do not have memory or learning capabilities, meaning they cannot improve over time. Instead, they provide instant responses based on specific inputs.
In e-commerce, reactive AI agents are commonly used in chatbots that handle frequently asked questions (FAQs) and solve minimalist problems. They also power rule-based recommendation engines that suggest bestsellers or trending products. Additionally, some basic fraud detection systems use reactive AI to identify anomalies based on predefined criteria.
For example, a chatbot that answers customer inquiries about return policies or available discounts operates as a reactive AI agent. These systems are useful for handling simple, repetitive tasks but lack adaptability.
Model-Based AI Agents (Limited Memory AI)
Unlike reactive agents, model-based AI agents have memory and learning capabilities. They use machine learning algorithms to analyze past interactions and refine their decision-making processes. These agents are widely used in e-commerce to enhance personalization and improve operational efficiency.
A major application of model-based AI agents is personalized product recommendations. Platforms like Amazon and Netflix utilize collaborative filtering models to refine suggestions based on user behavior. Another critical use case is dynamic pricing, where AI adjusts product prices based on demand, competitor prices, and inventory levels.
AI-powered chatbots with learning capabilities also fall under this category. These chatbots improve over time by analyzing past conversations and predicting better responses for future customer interactions.
Goal-Oriented AI Agents (Utility-Based Agents)
Goal-oriented AI agents optimize for specific objectives, such as increasing conversions, reducing cart abandonment rates, or improving customer engagement. These agents use reinforcement learning to evaluate different strategies and refine their approach based on performance.
One of the most impactful use cases of goal-oriented AI in e-commerce is marketing automation. AI-driven systems analyze customer data to optimize email campaigns, social media ads, and retargeting strategies. Additionally, these agents improve inventory management by predicting demand fluctuations and ensuring stock levels are balanced.
Another application is AI-powered retargeting, where the system identifies potential customers who abandoned their carts and delivers personalized ads to encourage them to complete their purchases. By continuously learning from user interactions, goal-oriented AI agents help businesses achieve better outcomes.
Learning AI Agents (Cognitive Agents)
Learning AI agents leverage deep learning and neural networks to make autonomous decisions. These agents continuously evolve by analyzing vast amounts of data, identifying patterns, and adapting their strategies accordingly.
One of the key applications of cognitive AI agents in e-commerce is sentiment analysis. These agents scan customer reviews, social media posts, and feedback to gauge customer satisfaction and identify areas of improvement. AI-powered virtual shopping assistants also fall under this category, providing fashion recommendations based on user history and preferences.
Another important use case is churn prediction. By analyzing customer behavior, AI can identify users likely to stop using a service and trigger personalized retention campaigns to keep them engaged. These advanced AI agents significantly enhance customer experience and business intelligence.
Autonomous AI Agents (Self-Learning & Self-Healing AI)
Autonomous AI agents function without human intervention, making decisions based on continuously evolving data. These agents utilize advanced reinforcement learning algorithms to optimize various e-commerce operations.
A prime example of autonomous AI in e-commerce is warehouse automation. AI-powered robots handle inventory, manage stock levels, and fulfill orders with minimal human oversight. Logistics optimization is another key application, where AI dynamically adjusts delivery routes based on traffic, weather conditions, and demand.
Customer service automation also benefits from autonomous AI, as these agents can handle complex queries, process returns, and even initiate refunds without requiring human intervention. Businesses implementing such AI agents achieve higher operational efficiency and scalability.
Multi-Agent AI Systems (Collaborative AI Agents)
Multi-agent AI systems involve multiple AI agents working together to perform complex tasks. These systems rely on multi-agent systems (MAS) and federated learning to enhance collaboration across different business functions.
In e-commerce, multi-agent AI is widely used in supply chain management. AI agents coordinate with manufacturers, suppliers, and logistics providers to streamline operations and ensure efficient inventory distribution. Fraud detection is another critical use case, where multiple AI agents analyze transactional data to detect suspicious activities.
Customer support automation also benefits from multi-agent AI. While one AI agent handles general inquiries, another may escalate complex issues to a human representative or suggest alternative solutions. These systems improve efficiency, reduce operational costs, and enhance the overall shopping experience.
Key Components Of AI Agents In Ecommerce
The table below outlines the essential components that enable AI agents to function efficiently in e-commerce environments.
Component | Description | Use Case in E-Commerce |
Machine Learning (ML) Models | Algorithms that allow AI agents to learn from data and improve decision-making over time. | Personalized product recommendations, and demand forecasting. |
Natural Language Processing (NLP) | Enables AI agents to understand, interpret, and respond to human language. | Chatbots, voice search, AI-powered customer support. |
Computer Vision | AI’s ability to analyze and interpret images or videos. | Virtual try-ons, image-based product search, fraud detection. |
Reinforcement Learning (RL) | A self-learning approach where AI improves actions based on rewards and penalties. | Dynamic pricing, inventory optimization, and marketing automation. |
Big Data Analytics | Processing large datasets to extract meaningful insights for better decision-making. | Customer behavior analysis, and market trend predictions. |
Predictive Analytics | Uses historical data to forecast future trends. | Churn prediction, personalized promotions, demand estimation. |
Knowledge Graphs | AI structures and connects data relationships for contextual understanding. | Semantic search, intelligent product categorization. |
Multi-Agent Systems (MAS) | Multiple AI agents work collaboratively to solve complex tasks. | Supply chain automation, fraud detection, and prevention. |
Edge Computing | AI processes data closer to the source rather than relying on cloud computing. | Personalization, and faster chatbot responses. |
Autonomous Decision-Making | AI independently makes decisions based on available data. | Automated customer service, AI-driven logistics, and self-learning recommendation engines. |
Challenges And Consideration Of AI Agents In Ecommerce And Retail
With advantages such as automation and enhanced customer support, AI poses risks to your business, such as data privacy, bias in AI algorithms, AI transparency, and more. Here are some challenges and considerations to be aware of while developing an AI agent:
-
Data Privacy and Security Concerns
AI agents rely on vast amounts of customer data to deliver personalized experiences. This raises concerns about data privacy, as businesses must comply with regulations like GDPR (General Data Protection Regulation) and CCPA (California Consumer Privacy Act). Unauthorized access, data breaches, and improper handling of sensitive information can lead to legal and reputational risks.
Considerations:
- Implement end-to-end encryption and secure authentication mechanisms.
- Ensure compliance with global data protection regulations.
- Use anonymization techniques to protect customer identities.
-
Bias in AI Algorithms
AI models are trained on historical data, which may contain biases. This can lead to unfair recommendations, discriminatory pricing, or biased hiring decisions in e-commerce platforms. If not addressed, biased AI can harm customer trust and brand reputation.
Considerations:
- Regularly audit AI models to detect and mitigate biases.
- Use diverse datasets to improve fairness in decision-making.
- Implement explainable AI (XAI) to make AI decisions more transparent.
-
High Implementation Costs
Deploying AI agents requires substantial investment in infrastructure, skilled personnel, and data processing capabilities. Smaller businesses may find it difficult to afford AI-driven automation and analytics tools.
Considerations:
- Start with low-cost AI solutions such as cloud-based AI services.
- Adopt a phased AI implementation strategy to manage costs.
- Evaluate ROI (Return on Investment) before large-scale deployment.
-
Customer Trust and AI Transparency
Customers are often skeptical about AI-driven recommendations and automated decisions, especially in cases of dynamic pricing or chatbot interactions. Lack of transparency in AI operations can lead to distrust and reluctance to engage with AI-powered services.
Considerations:
- Provide clear explanations of AI-generated decisions.
- Allow users to opt out of AI personalization if desired.
- Implement hybrid AI-human customer support for sensitive queries.
-
Integration with Existing Systems
Many e-commerce platforms use legacy systems that may not be compatible with modern AI-driven solutions. Integrating AI agents with such systems can be technically complex and resource-intensive.
Considerations:
- Use APIs and middleware to facilitate smooth integration.
- Opt for modular AI solutions that work with existing systems.
- Conduct thorough compatibility testing before full deployment.
-
Ethical and Legal Challenges
AI-powered automation in retail can lead to job displacement, raising ethical concerns about workforce reduction. Additionally, AI-generated content and decisions must comply with legal guidelines to avoid deceptive advertising or false product claims. Moreover, ecommerce app personalization must be implemented responsibly to ensure fair recommendations and transparency in AI-driven interactions.
Considerations:
- Implement reskilling programs for employees affected by AI automation.
- Ensure AI-generated marketing content adheres to consumer protection laws.
- Follow ethical AI guidelines to maintain fair business practices.
-
Scalability and Performance Issues
AI agents must handle high traffic volumes during peak sales seasons (e.g., Black Friday, and holiday sales). Poorly optimized AI models can lead to slow response times, affecting customer experience and sales conversions.
Considerations:
- Use cloud-based AI solutions for scalable performance.
- Optimize AI algorithms for decision-making.
- Conduct load testing to ensure AI agents can handle peak demand.
-
Fraud Detection and Security Risks
AI systems are vulnerable to adversarial attacks, where cybercriminals manipulate AI models to bypass fraud detection systems. AI agents must constantly evolve to detect new fraud tactics, such as fake reviews, phishing attempts, and transaction fraud.
Considerations:
- Implement adaptive fraud detection using deep learning models.
- Regularly update AI models to detect new fraudulent patterns.
- Use multi-layered security measures to prevent AI exploitation.
How Much Does It Cost To Develop Ecommerce AI Agent?
The cost of developing an AI agent depends on several factors, including its complexity, features, technology stack, and development approach. Below is a detailed breakdown of the estimated costs involved.
Type Of AI Agent
The type of AI agent and the project size play a major role in deciding the cost of developing an Ecommerce AI Agent. Here is a tabular representation of the cost of developing an AI agent:
Project Complexity | Estimated Cost | Development Timeline |
Basic AI Chatbot | $5,000 – $15,000 | 1 – 2 months |
Standard AI Agent | $20,000 – $80,000 | 3 – 6 months |
Advanced AI System | $80,000 – $200,000+ | 6 – 12 months |
Development Approach
The development approach of the AI agent also plays a major role in deciding the actual cost of development. For example, if you are going with custom AI agent development services then the cost would be high compared to using third-party APIs for the development.
Features And Functionalities
The cost also depends on the list of features that you want to include in your Ecommerce AI agent. Features like a personalized recommendation engine, voice search, predictive analysis, and more.
All these features add to the cost of developing an ecommerce AI agent.
AI Model Training and Data Processing
Training an AI model requires significant investment, depending on data volume and processing needs. Using pre-trained AI models is more affordable, with costs ranging from $10,000 – $30,000. In contrast, custom AI training, which involves building models from scratch using proprietary datasets, can cost between $50,000 and $200,000. The cost also varies depending on whether the AI model requires learning and adaptation to new data.
What Is The Process To Develop An Ecommerce AI Agent?
After knowing the cost to develop ecommerce AI agents let’s check its development process. Developing an AI-powered e-commerce agent requires a structured approach, from defining requirements to deployment and maintenance. The process involves multiple stages, including data collection, AI model training, and system integration, ensuring the AI agent can effectively enhance customer experience and business operations.
Requirement Analysis and Planning
The first step in developing an e-commerce AI agent is identifying business needs and defining objectives. This involves understanding customer pain points, market trends, and specific AI functionalities required. Businesses must determine whether they need an AI chatbot, recommendation system, voice assistant, or fraud detection AI. Defining key performance indicators (KPIs) at this stage helps measure the AI agent’s success.
Data Collection and Preprocessing
AI agents rely on vast amounts of data to function effectively. Businesses must gather data from multiple sources, including customer interactions, purchase history, browsing behavior, and support queries. This data is then cleaned, labeled, and structured to remove inconsistencies and improve training accuracy. The quality of data significantly impacts the AI model’s performance, making preprocessing a critical step.
Choosing an AI Model and Development Approach
The next step is selecting the right AI model based on the e-commerce agent’s functionalities. Businesses can either use pre-trained AI models from providers like OpenAI, Google AI, or IBM Watson or develop custom AI models for specialized tasks. If personalization and advanced decision-making are required, machine learning (ML) and deep learning (DL) algorithms are implemented. The choice of development approach—custom-built AI, third-party AI APIs, or hybrid AI solutions—affects both cost and flexibility.
AI Model Training and Optimization
Once the AI model is selected, it undergoes training using datasets. Machine learning algorithms analyze customer behavior patterns to improve accuracy in product recommendations, fraud detection, and personalized interactions. The model is continuously fine-tuned through supervised and unsupervised learning techniques, adjusting parameters to enhance decision-making capabilities. Natural Language Processing (NLP) is integrated into AI chatbots and voice assistants to improve conversational interactions.
Backend Development and System Integration
The AI agent must be seamlessly integrated with the e-commerce platform. Backend development involves connecting the AI model with the e-commerce website, mobile app, CRM, and payment gateways. APIs and cloud-based AI services are used to enable smooth interactions between the AI system and existing infrastructure. Integration ensures that AI-driven recommendations, chatbots, and automation work with minimal latency.
User Interface (UI) and Experience (UX) Design
For AI-powered chatbots and virtual assistants, an intuitive UI/UX is crucial for customer engagement. The AI agent should be easy to interact with, whether through text, voice, or a graphical interface. Features such as multi-language support, personalized recommendations, and interactive UI elements improve user experience and adoption.
Testing and Quality Assurance
Before deployment, rigorous testing is conducted to ensure the AI agent functions correctly across different scenarios. Testing involves:
- Functional Testing – Verifying AI-driven actions such as chatbot responses, recommendation accuracy, and fraud detection alerts.
- Performance Testing – Ensuring the AI system operates efficiently under high traffic conditions.
- Security Testing – Detecting vulnerabilities that could expose customer data or payment details.
- A/B Testing – Comparing different AI configurations to determine the most effective version.
Testing helps eliminate biases in AI models and improves response accuracy, ensuring a seamless user experience.
Deployment and Monitoring
Once testing is complete, the AI agent is deployed into a live e-commerce environment. It is continuously monitored to track performance, user interactions, and AI-driven outcomes. Analytics dashboards help businesses assess customer engagement and optimize AI functionalities. Any identified issues are resolved through frequent updates and patches.
Continuous Learning and Maintenance
AI agents require ongoing updates to improve accuracy and adapt to changing customer behavior. Regular model retraining using new datasets ensures the AI system remains relevant and effective. Businesses must also update AI functionalities to comply with new regulations, security requirements, and technological advancements. Periodic performance reviews help refine AI capabilities, enhancing automation and personalization in e-commerce operations.
How Does Appic Softwares Help You Develop An Engaging AI Agent?
Appic Softwares specializes in developing AI-driven solutions to the needs of e-commerce businesses. With expertise in artificial intelligence, machine learning, and automation, the company ensures that your AI agent enhances customer engagement, improves operational efficiency, and drives sales growth.
Custom AI Agent Development
Appic Softwares builds AI agents customized to match your business requirements. Whether you need a conversational chatbot, product recommendation engine, AI-powered customer support, or fraud detection system, the team develops solutions that align with your goals. The AI agents are trained on e-commerce data to ensure accurate decision-making and seamless integration into your platform.
Advanced Machine Learning and NLP Capabilities
The company incorporates machine learning (ML) and natural language processing (NLP) technologies to enhance AI performance. These capabilities allow AI agents to analyze customer behavior, predict user preferences, and generate personalized recommendations. NLP-powered chatbots improve customer interactions by understanding queries, providing instant responses, and adapting to different languages and dialects.
Seamless E-Commerce Integration
Appic Softwares ensures that AI agents integrate seamlessly with your existing e-commerce platforms, mobile apps, CRM systems, and payment gateways. The team works with leading technologies such as Shopify, Magento, WooCommerce, and custom-built solutions to provide a unified AI-powered shopping experience. The integration process is optimized for minimal downtime and maximum efficiency.
Scalable and Secure AI Solutions
AI agents developed by Appic Softwares are built with scalability in mind. The company leverages cloud-based AI services and microservices architecture to ensure that your AI agent can handle high traffic volumes and grow with your business. Security is a top priority, with end-to-end encryption, fraud detection algorithms, and compliance with industry regulations ensuring customer data remains protected.
Analytics and Continuous Improvement
Appic Softwares provides analytics dashboards to help businesses monitor AI agent performance. By analyzing key metrics such as customer interactions, conversion rates, and AI-driven recommendations, businesses can fine-tune their AI solutions for optimal results. The AI models are continuously updated using new data and feedback loops, ensuring that the system evolves with market trends and user behaviors.
Conclusion
Appic Softwares empowers e-commerce businesses with AI-driven solutions that enhance customer engagement and streamline operations. From custom AI development and seamless integration to analytics and continuous improvement, the company delivers innovative AI agents designed for long-term success. By leveraging advanced technologies and industry expertise, Appic Softwares a leading AI development company stay ahead in the competitive e-commerce and AI landscape.
FAQs
1. What Is an E-Commerce AI Agent?
An e-commerce AI agent is an artificial intelligence-powered system designed to automate various tasks in an online store. These tasks include customer support, personalized recommendations, fraud detection, inventory management, and chatbot assistance. AI agents improve user experience and operational efficiency by analyzing data and making intelligent decisions.
2. How Does an AI Agent Enhance Customer Experience in E-Commerce?
AI agents personalize the shopping experience by analyzing customer behavior, preferences, and purchase history to provide recommendations. They also offer 24/7 customer support through chatbots, automate responses, and assist in handling product inquiries and complaints, ensuring a seamless shopping journey.
3. What Technologies Are Used to Develop E-Commerce AI Agents?
Developing an AI agent for e-commerce involves various technologies, including:
- Machine Learning (ML) & Deep Learning – For predictive analytics and decision-making.
- Natural Language Processing (NLP) – For chatbots and voice assistants.
- Computer Vision – For visual search and automated product tagging.
- Big Data & Analytics – To process large datasets for customer insights.
- Cloud Computing – For scalability and processing.
4. Can AI Agents Be Integrated with Existing E-Commerce Platforms?
Yes, AI agents can be seamlessly integrated with Shopify, Magento, WooCommerce, Adobe Commerce, and custom e-commerce solutions. They can work alongside existing CRM systems, payment gateways, and marketing tools to optimize business operations.
5. How Much Does It Cost to Develop an E-Commerce AI Agent?
The cost of developing an AI agent varies based on complexity, features, AI model customization, integrations, and cloud infrastructure. Basic AI chatbots may cost $5,000 to $15,000, while advanced AI agents with machine learning and predictive analytics can range from $30,000 to $100,000 or more. Ongoing maintenance and updates also influence long-term costs.
6. What Are the Key Challenges in Developing an E-Commerce AI Agent?
Some major challenges include:
- Data Quality Issues – AI models require large, well-structured datasets for accurate predictions.
- Integration Complexities – Ensuring smooth compatibility with existing platforms.
- Security & Privacy Concerns – Protecting customer data from breaches.
- High Development Costs – Custom AI models can be expensive to develop and maintain.
- Bias in AI Models – AI decisions need to be fair and unbiased to provide accurate recommendations.
7. How Long Does It Take to Develop an AI Agent for E-Commerce?
The development time depends on the complexity of the AI agent. A basic chatbot can be built in 4 to 6 weeks, while an advanced AI system with personalized recommendations and predictive analytics may take 4 to 6 months or more. Continuous improvements and updates extend the AI’s lifecycle.
8. Can AI Agents Help Reduce Cart Abandonment Rates?
Yes, AI agents can send automated reminders, offer personalized discounts, and provide assistance to customers who leave items in their carts. AI-powered predictive analytics can also help businesses understand why users abandon carts and implement strategies to recover lost sales.
9. How Secure Are AI-Powered E-Commerce Solutions?
AI agents use encryption, multi-factor authentication, fraud detection algorithms, and compliance protocols to ensure security. Businesses must adhere to GDPR, CCPA, and PCI DSS regulations to protect customer data. Regular security audits help maintain a safe environment.
10. How Can Appic Softwares Help in Developing an AI Agent for My E-Commerce Business?
Appic Softwares provides custom AI solutions, seamless integrations, machine learning-driven personalization, fraud detection, and analytics to enhance e-commerce platforms. With expertise in AI-powered chatbots, voice assistants, and recommendation engines, Appic Softwares ensures an engaging and efficient shopping experience for customers.