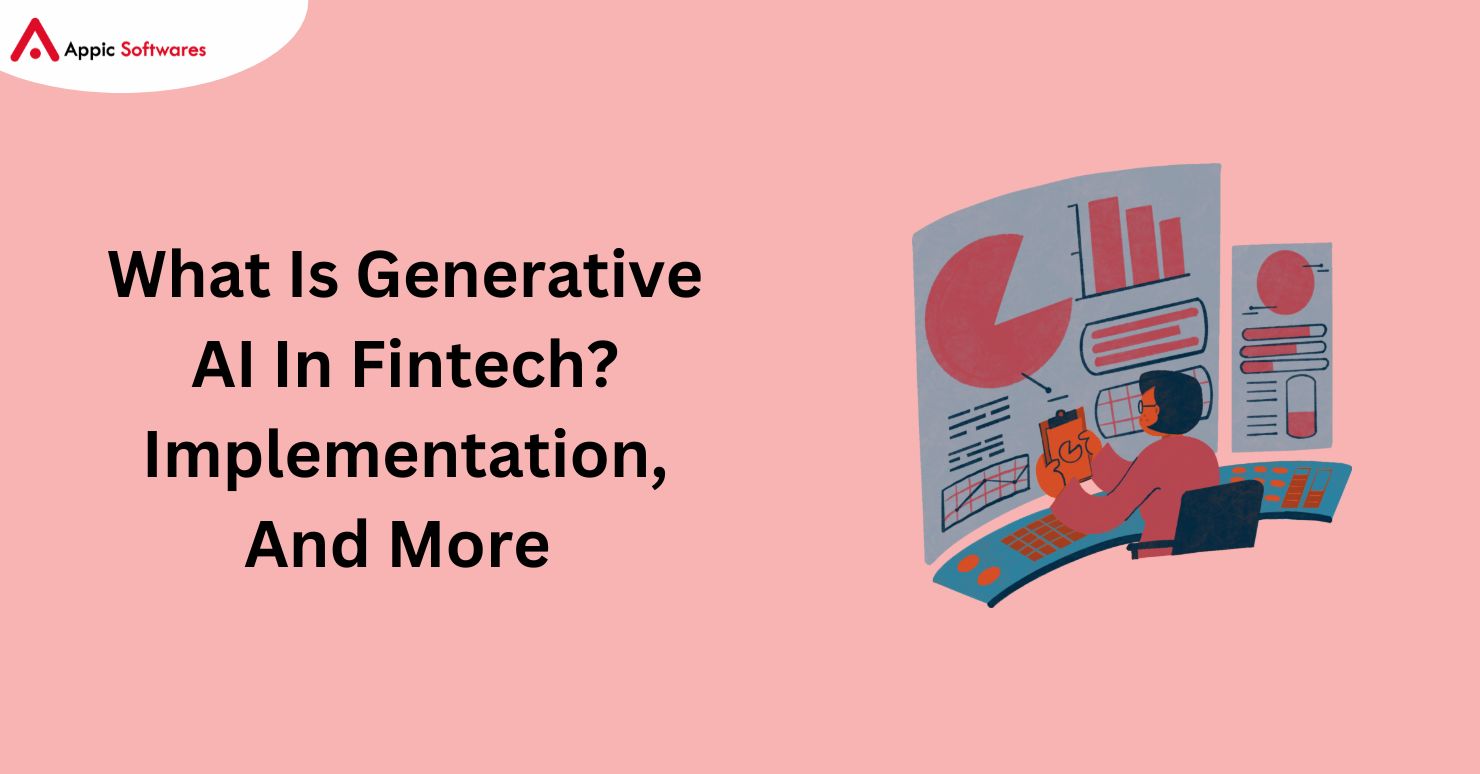
Among FinTech’s many applications, GenAI shines when it comes to improving customer service with sophisticated chatbots, making accurate predictions about the financial markets, and building synthetic datasets for risk assessment. A staggering 91% of fintech companies are either exploring AI or have begun implementing it into their operations, says NVIDIA. For FinTech companies, generative AI is a godsend due to its capacity to sort through massive data sets, automate procedures, and predict results. Banks and other financial companies can benefit from generative AI tools by making data-driven decisions, improving their risk management, and catering to customers’ unique demands. Read on to find out what’s new in the generative AI FinTech industry, how it’s influencing innovation, and some success stories from those who have used it recently.
Generative AI In FinTech: Implementation And Integration Process
Implementing and integrating generative AI into fintech operations is a complex yet rewarding endeavor. To successfully adopt this technology, financial institutions must follow a structured process that ensures alignment with business objectives, efficient deployment, and continuous optimization. Here’s a detailed breakdown of the implementation and integration process, complete with two relevant statistics to underscore the impact of generative AI in fintech.
1. Data Analysis And Planning
The foundation of any generative AI project in fintech lies in comprehensive data analysis and strategic planning. This stage involves several key steps:
- Assess Property Data Needs: Begin by evaluating the types of data required to support the generative AI models. This may include customer transaction data, market trends, regulatory information, and other relevant data sources. Understanding data requirements helps in selecting the right datasets and ensuring data quality.
- Define Objectives and Use Cases: Clearly define the objectives of the AI implementation, such as improving customer service, enhancing fraud detection, or automating compliance processes. Identifying specific use cases ensures that the AI deployment aligns with business goals and delivers tangible results.
- Identify Stakeholders and Data Sources: Engage with key stakeholders, including data scientists, compliance officers, and business leaders, to gather input and support for the AI initiative. Additionally, identify all relevant data sources, both internal and external, that will be used to train and optimize the AI models.
- Develop Implementation Strategy: Create a detailed implementation strategy that outlines the steps, timelines, and resources required for the AI project. This strategy should include a risk assessment, budget considerations, and a plan for addressing potential challenges.
2. LLM Selection & Acquisition
The global AI in fintech market is expected to grow at a CAGR of 15.9% from 2021 to 2028, reaching a market size of $58701.4 million by 2034, driven by the adoption of advanced AI technologies like LLMs. Selecting the appropriate Large Language Model (LLM) is crucial for the success of generative AI in fintech. The following steps are essential:
- Research LLM Providers: Conduct thorough research on available LLM providers, such as OpenAI, Google, or AWS, to identify models that align with your specific needs. Consider factors like model size, training data, and performance benchmarks.
- Assess Model Capabilities: Evaluate the capabilities of different LLMs, including their ability to understand and generate financial language, process large volumes of data, and integrate with existing systems. Consider the model’s adaptability to fintech-specific use cases, such as risk management or customer service automation.
- Evaluate Licensing and Pricing: Analyze the licensing options and pricing structures offered by LLM providers. Ensure that the selected model fits within the organization’s budget and offers a cost-effective solution for long-term use.
- Procure & Implement LLM Solutions: Once the ideal LLM is identified, proceed with procurement and implementation. This involves integrating the model into existing workflows, configuring it to meet organizational needs, and ensuring it complies with regulatory requirements.
3. Integration And Deployment
The integration and deployment phase is where the generative AI models are brought to life within the organization’s systems:
- Data Integration and Preprocessing: Begin by integrating the selected LLM with the organization’s existing data infrastructure. This may involve cleaning, transforming, and preprocessing data to ensure compatibility with the AI model. Proper data integration is critical for accurate and reliable AI outputs.
- Model Training and Fine-Tuning: Train the LLM on the organization’s specific datasets to tailor its performance to fintech-related tasks. Fine-tune the model to optimize its accuracy, relevance, and efficiency in generating insights and recommendations.
- Testing and Validation: Rigorously test the AI model to ensure it meets the defined objectives and performs well under real-world conditions. Validation involves comparing the model’s outputs with expected results to confirm its accuracy and reliability.
- Training and Knowledge Transfer: Provide comprehensive training to employees on how to use and interact with the generative AI system. Knowledge transfer is essential for ensuring that all stakeholders can leverage the AI model effectively, maximizing its impact on business operations.
4. Monitoring And Optimization
Once the generative AI model is deployed, ongoing monitoring and optimization are essential to maintain its effectiveness and adapt to changing conditions:
- Performance Monitoring & Feedback: Continuously monitor the AI model’s performance to identify any issues or areas for improvement. Collect feedback from users and stakeholders to understand how the model is being utilized and where it can be enhanced.
- Continuous Model Improvement: Based on the feedback and performance data, make iterative improvements to the AI model. This may involve retraining the model with new data, adjusting algorithms, or fine-tuning its parameters to enhance accuracy and relevance.
- Scale and Expand Usage: As the AI model proves successful, consider scaling its usage across different departments or business units. Explore new use cases where the AI can add value, such as expanding from customer service automation to fraud detection.
- Compliance and Security: Ensure that the AI model continues to comply with all relevant regulations and security standards. Regularly update the model’s security protocols to protect against potential threats and ensure the privacy of customer data.
7 Use Cases Of Generative AI In Fintech
The topic of generative AI is relatively new and expanding quickly. AI has the potential to contribute up to $15.7 trillion to the global economy by 2030, more than China and India’s combined production. Even if there is so much untapped potential, businesses still need to identify use cases that add value to their operations and yield a profit. Here are six applications of generative AI in fintech for firms.
1. Processing Of Documents Intelligently
Intelligent document processing (IDP) is the process of extracting and analyzing useful information from massive amounts of documents by utilizing machine learning techniques, data analytics, huge language models, and AWS services like Textract and Comprehend. Using this highly scalable method instead of hiring human writers or readers results in speedier and more accurate results.
IDP can help fintech companies expedite loan applications, mortgage underwriting, and compliance paperwork. In the past, loan applications have required manual document reviews of bank statements, tax returns, income statements, and other records. This method takes a long time, is prone to mistakes, and frequently causes delays in loan applications.
Important data, like debt-to-income ratios, credit histories, and income verification, can be automatically pulled from documents using IDP. This facilitates a quicker application process and allows loan officers to make choices more rapidly based on information.
2. Recapitulating Data
More data and information are available to businesses than ever before, which makes it more difficult for them to evaluate it fast and correctly. More quickly than a person, generative AI systems can scan and summarize the data while providing important insights clearly and succinctly. Executives, staff members, and other stakeholders can now easily catch up and utilize the insights to make well-informed decisions.
Users can ask additional questions about businesses, industries, investment opportunities, trends, and correlations thanks to generative AI’s use of natural language processing. Investment professionals’ demands and preferences can be accommodated via models. They gain experience and hone their summarizing skills over time, offering more specialized insights that complement business and investment strategies.
3. Assessing Investment Possibilities
Fintech companies can use generative AI to summarize a wide range of data, including investment prospects. When a large amount of the information is either poorly organized or not publicly available, this technology can be extremely useful.
Processing enormous volumes of disorganized and unstructured data from many information sources is an area in which generative AI shines. For instance, private equity firms frequently have to evaluate companies that have little public exposure while also taking the macroenvironment and other valuation-influencing aspects into consideration. Artificial intelligence (AI) systems have the ability to uncover themes and emotions that are hard or impossible for people or other technology to piece together.
Companies trying to track information that is visible to the public, such as earnings calls, press releases, or discussions on social media, might also benefit from generative AI in order to gauge public opinion and spot new trends. Additionally, GenAI is able to monitor indicators of possible prospects, such as a tiny research company’s advancement toward receiving regulatory approval for a new medication.
4. Building Financial Advisors With AI
Chatbots with generative AI capabilities can respond to consumer inquiries, offer financial advice, and offer individualized support. They can analyze consumer information, transaction history, and other data to make customized recommendations; they are not restricted to a basic set of scripts. Users can also get assistance from chatbots with financial planning, budgeting, and account administration.
Additionally, investors can communicate with robo-advisors through generative AI, which offers an affordable alternative for investment management. Robo-advisors evaluate an investor’s risk tolerance, financial goals, and market conditions. Subsequently, they provide automatic investment suggestions, encompassing asset allocation tactics, portfolio realignment, and investment diversity.
5. Setting Up AI-Driven Contact Centers
AI-powered contact centers let agents respond to consumer inquiries faster and more effectively. Additionally, self-service can be made more possible with AI tools, freeing up agents to handle the most complicated and high-priority consumer inquiries. Precision, consistency, and speedy customer service are provided by AI-powered contact centers when paired with AWS services like Connect and Lex.
Conversational AI interfaces offered by chatbots created with Lex can offer simple and natural self-service experiences. Interfaces for text and voice can be integrated into important channels. Interestingly, businesses don’t pay for speech or text requests until they happen.
6. Creating Tailored Marketing Content
Companies may improve marketing automation through tailored content by integrating AWS, generative AI tools, and consumer profiles. Companies no longer need to send out impersonal, perhaps mistimed messages that miss the mark; instead, they can respond instantly to shifts in consumer opinion.
Simple Email Service (SES) from Amazon offers organizations a scalable and affordable way to send customized emails. In a similar vein, Amazon Pinpoint facilitates consumer interaction for companies via email, SMS, push alerts, and voice. It provides analytics, campaign management, and audience segmentation features.
With real-time streaming data processing provided by Amazon Kinesis, companies can swiftly respond to shifts in the market and modify their content as necessary. Businesses can deliver personalized content based on client choices and behaviors and automate marketing processes with AWS Lambda, a serverless computing solution.
7. Recognizing And Avoiding Fraud
In the fintech sector, where there are significant risks and a dynamic threat landscape, fraud detection and prevention are essential. Generic AI can identify patterns and anomalies that traditional algorithms would miss, improving conventional fraud detection techniques. AI is able to identify anomalous activity in real-time and avert fraudulent transactions by evaluating large volumes of transaction data, user behavior, and past fraud cases.
For instance, to determine the possibility of fraudulent activity, generative AI can examine transaction sequences, consumer behavior, and even outside variables like geopolitical developments. To lower the chance of fraud, the system can automatically stop a transaction or flag it for additional inspection if it looks suspect.
Pros And Cons Of Generative AI
Generative AI Pros
Fintech is one of the many industries where generative AI has several benefits. The following are some main advantages and uses:
1. Data Generation And Interpretation
Generative AI is very good at creating new data, which is essential for tasks like organizing, processing, synthesizing, and augmenting existing data. It facilitates the creation of marketing data, medical imaging, maps from satellite imagery, and the interpretation of complicated systems. With this feature, decision-making procedures are streamlined and time and human error are greatly decreased.
2. Content development And Creativity
The innovative nature of generative AI makes it possible to create original texts, images, and music. For example, DALL-E and Canva can create graphics based on textual descriptions, whereas ChatGPT can create long-form text, code, and even lyrics. This makes it possible for people and businesses to produce content more effectively and efficiently.
3. Increased Productivity And Economical Remedies
Time savings is one of generative AI’s most notable benefits. Even with its considerable time investment, traditional content creation may not be able to satisfy all user’s needs. On the other hand, generative AI can produce targeted, valuable content more quickly, saving money and time.
4. Tailored Material
Creating individualized material is now easier thanks to AI models like ChatGPT and Gemini. Businesses and experts can customize content to the tastes of their target audience, producing more interesting and viral content. In terms of fostering closer ties with viewers and optimizing client relationships, this is revolutionary.
Generative AI Cons
Generative AI has certain disadvantages despite its benefits:
1. Quality And Accuracy Challenges
Large datasets that are only as current as the data used for training are utilized to train generative AI models. These models might therefore find it difficult to deliver precise, current information. For instance, ChatGPT occasionally generates ambiguous or repetitious responses, especially when addressing recent occurrences. Similarly, when it falsely stated that the James Webb Space Telescope had taken the first pictures of a planet outside of our solar system, Google’s Bard came under fire.
2. Legal And Ethical Issues
generative AI systems like ChatGPT have raised questions about intellectual property rights. Concerns over the originality and ownership of content produced by AI have surfaced since these models are trained on big datasets containing work from a variety of creators. Legal and ethical issues arise from this, especially when AI-generated content is strikingly similar to previously published works.
3. Adoption And Technological Barriers
Especially for small enterprises and those who are not familiar with the technology, generative AI adoption might be challenging. There are restrictions on what can be generated images and downtimes during periods of high demand for free AI services like ChatGPT and DALL-E. Furthermore, because of high computing costs and inefficiencies in the models, developing generative AI capabilities internally can provide substantial technological obstacles.
4. Problems With Data Labeling
Unlabeled data, which may not necessarily originate from reliable or trustworthy sources, is a common component of generative AI models. Consistency and dependability are questioned during the data mixing and matching process, which may have an impact on the final product’s quality. OpenAI, Facebook, and TikTok use contract labor for data classification, highlighting data quality issues.
Examples Of Cutting-Edge Generative AI Innovations
Generative AI has led to groundbreaking developments across various fields:
- GPT (Generative Pre-trained Transformer): OpenAI’s text generator is renowned for producing high-quality written content.
- Neural Machine Translation (NMT): These AI models translate text between languages with remarkable accuracy, enhancing global communication.
- Text-to-Speech (TTS) Systems: AI-driven TTS systems generate natural-sounding speech from text, revolutionizing voice-based interactions.
- DALL-E: Another OpenAI innovation, this model creates unique images from text descriptions.
- StyleGAN: Nvidia’s model generates hyper-realistic images of faces, animals, and objects.
- MuseNet: OpenAI’s music composition tool generates original music in various styles, demonstrating AI’s versatility in creative fields.
Conclusion
Generative AI is transforming the fintech landscape by enhancing data analysis, improving customer interactions, and optimizing risk management. Its ability to automate complex processes, generate insights, and prevent fraud makes it an invaluable tool for financial institutions. However, it is important to consider the challenges, such as maintaining data accuracy and addressing ethical concerns.
If you’re looking to leverage the power of generative AI in fintech, partner with Appic Softwares, the leading fintech app development company.
Contact us today to innovate your business and stay ahead in the financial revolution!