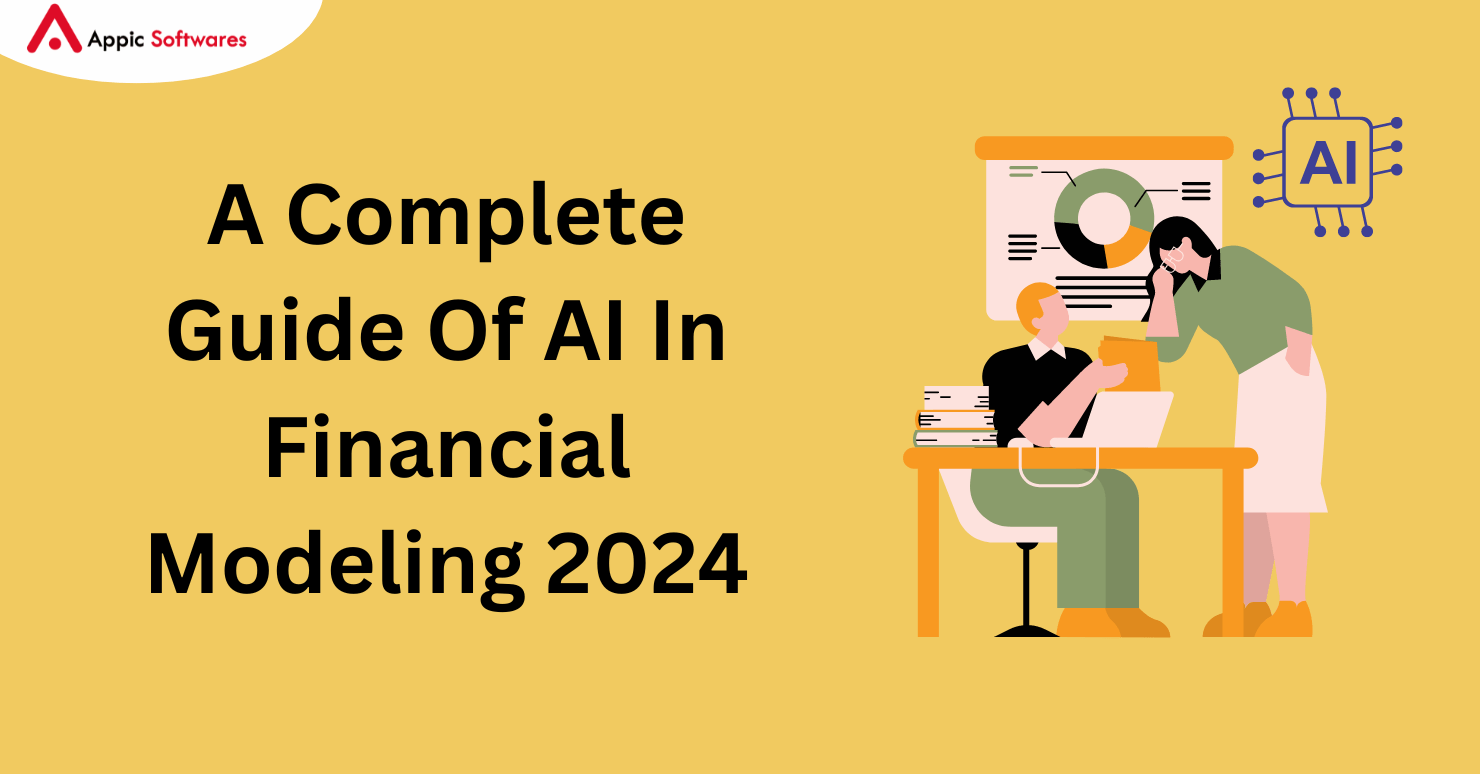
Every choice you make in the high-risk financial sector might be the difference between success and failure. It is impossible to overestimate the importance of precise and flexible financial modeling in such a volatile industry. Assume a multinational investment company is thinking about making a multimillion-dollar purchase. They must analyze vast amounts of financial data, evaluate risks, and forecast future performance precisely to make an educated choice. In a circumstance like this, a hasty choice might cost the company millions of dollars in projects. This is the point at which financial modeling becomes important.
The foundation of strategic financial decision-making is financial modeling. It functions as a guiding tool for cash flow forecasts, investment choices, and opportunity discovery in the fast-paced financial business environment. Traditional financial modeling, on the other hand, is labor-intensive and prone to human error when processing large datasets and responding to changing market circumstances. In a situation like this, artificial intelligence (AI) intervenes as a game-changer, revolutionizing financial modeling and improving financial decision-making.
This article examines the use of AI in financial modeling as well as its advantages in the investing and private equity industries.
What is financial modeling?
The practice of utilizing diverse financial facts, variables, and assumptions to create a mathematical representation or simulation of a financial condition or a firm is known as financial modeling. It is an essential instrument for financial and investment analysis that is used for planning, forecasting, and decision-making. In summary, financial models combine accounting, business analytics, and finance to assist in forecasting a company’s future financial performance. They may be used in a variety of situations, including risk assessment, investment opportunity evaluation, future financial performance projection, and firm valuation.
Financial models are often shown using a variety of techniques and tools, depending on the model’s complexity, intended use, and modeler preferences. Spreadsheet programs like Microsoft Excel are among the most used tools for creating financial models. Excel worksheets may include cells with the ability to store data, formulas, and functions. The connected spreadsheets that make up Excel’s built-in financial modeling capability often represent different parts of the model, such as the cash flow statement, balance sheet, and income statement.
What is a financial model used for?
Financial models are used in the business, investment, and financial domains for a variety of reasons. These are a few uses for financial models:
- Valuation: To determine the worth of a business, an asset, or an investment, financial models are used. Discounted cash flow (DCF), comparable company (CCA), and previous transactions analysis are common methods of valuation.
- Financial models are used by investors in investment research to evaluate the possible risks and returns of various investment options, including stocks, bonds, real estate, and startups.
- Financial models are used by businesses to plan, establish financial targets, and make well-informed choices on resource allocation, capital allocation, and budgetary constraints.
- Financial models are essential to mergers and acquisitions (M&A) negotiations because they assist buyers and sellers in analyzing the financial effects of a deal, figuring out a reasonable purchase price, and identifying possible synergies.
- Project finance: By projecting future cash flows, debt payment costs, and return on investment, financial models assess the viability and profitability of large-scale projects, such as infrastructure construction.
- Risk management: To evaluate and control financial risks including interest rate, currency, and credit risk, financial models are used. For risk assessments, Monte Carlo simulations are often used.
- Financial models are used by businesses to generate projections and budgets that help them prepare for cash flow, costs, and income in the next months and years.
- Startup valuation: To draw in investors, get capital, and showcase their development prospects, startups, and early-stage businesses use financial models.
- Real estate analysis: To assess the financial sustainability of real estate investments, including determining property prices, rental revenue, and return on investment, real estate experts use financial models.
- Loan and credit analysis: To evaluate borrowers’ creditworthiness and set conditions for loans and credit lines, financial organizations use models.
- Portfolio management: To optimize asset allocation and risk-adjusted returns, investment managers build and oversee investment portfolios using financial models.
- Financial reporting: To create financial statements—such as income statements, balance sheets, and cash flow statements—for shareholder reporting and regulatory compliance, publicly listed corporations utilize financial models.
- Sensitivity analysis: To evaluate risk and make wise choices, sensitivity analysis is a process that includes analyzing the effects of various factors and assumptions on financial outcomes using financial models.
- Capital structuring: By examining different debt, equity, and other financing source combinations, financial models assist in maximizing a company’s capital structure and determining the best affordable and long-term capital mix.
- Tax planning: To help with effective tax planning and compliance, businesses use financial models to assess the tax ramifications of various company choices and tactics.
- Dividend policy analysis: Using the company’s profits, cash flow, and investment requirements as a guide, financial models help determine the best dividend policy.
- Operational optimization: Financial models may be used to estimate the financial effects of operational decisions such as facility expansions, product line extensions, or other strategic initiatives.
- Financial models are used for long-term strategic planning, which aids businesses in assessing possible avenues for development and expansion.
- Economic and market analysis: Financial models are often used to examine market conditions and economic trends, which helps with macroeconomic forecasting and market analysis.
- Financial models are used in the insurance sector for underwriting, which involves determining the possible risks and expenses related to insurance policies.
- Analysis of the energy and commodity markets: Financial models are used in these industries to forecast price and market changes, which is important information for trading and investment choices.
- Supply chain management: By examining costs, revenues, and logistical efficiency, financial models may be utilized to improve supply chain operations.
Financial models help with pension fund management, assisting in making sure there are enough assets on hand to cover future obligations.
Challenges in traditional financial modeling and how AI addresses them
AI may provide solutions that enhance decision-making, efficiency, and accuracy by addressing several issues with conventional financial modeling. The following are some major obstacles and how AI may assist with them:
Managing big and intricate data sets
- Challenge: Large volumes of data are difficult for traditional financial models to handle and evaluate quickly and properly.
- AI solution: From structured and unstructured data sources, AI algorithms can effectively handle massive and complicated data sets and extract insightful information. Machine learning models can recognize correlations and underlying patterns that people would find difficult to notice.
Error-prone procedures and manual data entering
- Problem: Inaccurate findings and judgments might arise from manually entering data into financial models.
- AI solution: By automating data collection, cleaning, and entry, AI lowers the possibility of mistakes. Data entry by hand may be avoided using Natural Language Processing (NLP), which extracts information from documents and reports.
arduous predictions and examination
- Challenge: Time-consuming activities like data analysis, forecasting, and scenario testing are often a part of traditional financial modeling.
- AI solution: These procedures are accelerated using AI. Rapid and accurate prediction-making is made possible by machine learning models, which speed up scenario analysis and decision-making.
Traditional metrics and restricted data sources
- Challenge: Conventional models could overlook important information because they depend too heavily on a small number of financial measures and data sources.
- AI solution: A wider variety of data sources, including unconventional data like news articles and social media sentiment, may be included by AI. This adds depth to the study and offers a more complete picture of the state of the market.
- The challenge of real-time analysis: Real-time insights are critical in the rapidly changing financial markets, yet they are sometimes difficult to get using traditional methods.
- AI solution: Real-time data analysis by AI-powered algorithms enables prompt response to shifting market circumstances, risk assessment, and opportunity seizing.
Complexity of risk management
- Challenge: Because markets are dynamic, successfully managing financial risks is a difficult endeavor.
- AI solution: In real-time, AI models can recognize and evaluate a variety of financial hazards, including credit, market, and operational risk. They help proactive risk management and provide early warnings.
- The challenge of complying with intricate financial standards and reporting requirements may be laborious and prone to errors.
- AI solution: AI reduces the possibility of regulatory infractions by automating compliance procedures, assuring accurate and timely reporting.
- Lack of customization Challenge: Conventional models could provide universal answers without taking into account each person’s preferences or risk tolerance.
- AI solution: By offering individualized portfolio management and investing advice based on each client’s unique objectives and risk tolerance, AI-powered robo-advisors improve the client experience.
- Problem with portfolio optimization: It might be difficult to manually build diversified portfolios, which can result in an asset allocation that is not ideal.
- AI solution: Using sophisticated algorithms, AI-powered portfolio optimization models build and manage portfolios to optimize risk-adjusted returns according to personal preferences.
Applications of AI in financial modeling
Data analysis and processing
AI is essential for improving data analysis and processing in financial modeling. Large amounts of financial data, including historical records, market data, and news sentiment, may be processed quickly by it. AI performs in-depth data analysis using machine learning algorithms, spotting trends, patterns, and anomalies that can be difficult to see with more conventional analytical techniques. This thorough data analysis greatly improves financial model accuracy, which in turn makes data-driven and more informed decision-making processes possible.
Automating repetitive activities
The potential of artificial intelligence to automate repetitive and time-consuming operations that are a part of financial modeling is one of its core features. These jobs involve, among other things, report creation, data input, and data purification. AI lowers the possibility of human mistakes in financial models by automating these tedious tasks, freeing up finance experts’ time and skills to focus on more crucial areas of financial research.
Analytics that predict
The predictive powers of AI are revolutionizing financial modeling. Machine learning algorithms can anticipate financial market movements, stock prices, and economic indicators with high accuracy. As a result, finance professionals may now access more trustworthy projections, supporting more informed financial planning and investment choices.
Processing of Natural Language (NLP)
According to an Nvidia study of over 200 finance-based businesses in America and Europe, the most popular AI use cases were found to be natural language processing and huge language models (26% of the replies). Thus, it is clear that NLP is becoming a key driver of innovation in the banking industry. AI is now able to read and understand textual data, such as earnings call transcripts, reports, and financial news, thanks to NLP technology. AI can evaluate market sentiment and its possible influence on investments by extracting important information and sentiment from text-based sources. Decision-makers may benefit from the analysis and incorporation of textual data into financial models, which provides useful information.
Evaluation and control of risks
In financial modeling, AI’s real-time risk assessment skills are invaluable. Credit risk, market risk, and operational risk are just a few of the financial hazards that AI models are quick to recognize and evaluate. Proactive risk management and prompt decision-making to minimize prospective losses are made possible by this real-time risk assessment.
Sensitivity analysis and scenario analysis
Artificial Intelligence simplifies scenario and sensitivity analysis by automating the testing of several situations. AI-driven models can quickly modify important financial model parameters to evaluate possible results in various scenarios. This automation makes it easier to assess how changes in certain factors affect financial outcomes, enabling a more thorough investigation.
Fraud protection and detection
To improve security in financial modeling, artificial intelligence is essential. Fraud detection systems powered by artificial intelligence examine transaction data to spot questionable trends and possible fraudulent activity. By supporting security measures, these technologies protect financial institutions’ customers from fraudulent activity.
Reporting requirements and compliance
AI-driven automation ensures compliance with intricate financial rules and reporting requirements by streamlining compliance procedures. AI ensures regulatory compliance more effectively by automating these operations, lowering the possibility of regulatory infractions and related fines.
Individualized financial guidance
Personalized portfolio management and investing advice are offered by AI-powered robo-advisors, taking into account each client’s unique risk tolerance and objectives. By providing more affordable options to conventional wealth management services, these solutions increase the accessibility of individualized financial advice for a wider group of investors.
Elements and components of a financial model
Important parts and pieces of a financial model might be:
- Historical data: To comprehend previous performance and trends, financial models sometimes begin with a review of historical financial data, such as income statements, balance sheets, and cash flow statements.
- Financial models are predicated on several assumptions regarding variables and circumstances in the future. Aspects like operational costs, inflation, interest rates, and market circumstances may be included in these assumptions.
- Financial statements: To provide a complete picture of the financial performance and status of the organization under analysis, the model usually includes projected financial statements such as income statements, cashflow charts, and equity change statement charts.
- Financial models are often used to forecast future revenues, taking into account variables like as pricing strategies, market demand, and increase in sales.
- Cost projections: They also anticipate future costs for things like taxes, interest, operational costs, and capital expenditures.
- Cash flow analysis: To evaluate the availability of money for several objectives, including debt repayment, investment, and dividend distribution, financial models compute cash flows.
- Scenario analysis: To assist decision-makers prepare for potential possibilities, financial models may include many scenarios that examine various probable outcomes under different situations.
- Risk assessment: To measure and control financial risks, sophisticated financial models may include risk assessment instruments like Monte Carlo simulations.
- Graphs and charts: The outcomes of the financial model might be simpler to understand and convey when visual aids like graphs and charts are used.
- Financial models often include valuation variables such as payback time, internal rate of return (IRR), and net present value (NPV). When evaluating the viability and profitability of investments, these measures are essential.
Who builds financial models?
Typically, experts with backgrounds in accounting, finance, and related professions create financial models. The following are a few positions and people in the business who work on creating financial models:
Finance and investment banking
Financial analysts are in charge of developing models for analysis in the fields of corporate finance, asset management, investment banking, and consultancy.
Investment bankers: These professionals develop models to assess businesses, acquisitions, mergers, and other financial transactions.
Finance for corporations
Professionals in corporate finance: They create models for capital allocation, forecasting, budgeting, and assessing investment prospects within businesses.
Teams that provide financial planning and analysis (FP&A): They concentrate on financial modeling for strategic planning and budgeting.
Exclusive ownership
Private equity analysts use intricate models to assess possible investments, carry out due diligence, and keep an eye on portfolio firms.
Portfolio managers: Based on risk and return analysis, portfolio managers make investment choices by using models to optimize asset allocation, evaluate portfolio performance, and make adjustments.
Consulting Management Consultants: Develop models, such as scenario modeling, market analysis, and cost evaluation, to aid in strategic decision-making.
Other industries
Financial planners: They assist customers with retirement planning, investment strategy evaluation, and goal achievement via the use of models.
Real estate analysts design models to analyze investment returns in real estate development and investment, as well as to examine the viability of properties and estimate values.
Risk managers: As the name implies, risk managers use models to evaluate and reduce risks associated with money, including market, credit, and interest rate concerns.
The people who develop mathematical models for trading strategies, risk management, and derivatives pricing are known as quantitative analysts or quants.
Governmental organizations: They predict tax receipts, plan public infrastructure projects, and assess the policy effect using financial models.
Each of these experts builds financial models to support analysis, planning, and decision-making, which is essential to their respective fields.
How does AI for financial modeling benefit the private equity and investment sector?
better judgment while making investments
Predictive analytics driven by AI can forecast changes in economic data, market activity, and stock prices with high accuracy. With the ability to identify trends, patterns, and unrealized opportunities rapidly, these technologies may help investors make more strategic and astute selections. By adding data-driven insights to human judgment, better investment choices may lead to higher returns, reduced risks, and a stronger position in the market.
Effective due diligence
Efficiency in the due diligence processes is one aspect of AI integration in the investment industry that sets it apart. By automating the collection and analysis of financial data, firm performance measures, and regulatory compliance, artificial intelligence (AI) accelerates the evaluation of potential investments. This automation reduces the chance of human mistakes, which increases accuracy while also saving a ton of time. Furthermore, AI can keep an eye on news, social media, and other external factors continually, providing crucial changes that might affect investing decisions. Effective due diligence thus makes it possible for investors to make decisions swiftly and efficiently using the resources at their disposal.
Portfolio streamlining
AI-powered financial models take into account a variety of significant factors, including investor risk tolerance, specific investment objectives, and market conditions. Investing in AI may enable investors to attain a level of asset allocation accuracy that was previously unattainable via the use of its massive processing power and data analysis capabilities. As a result, portfolios are precisely adjusted to react to changing market dynamics in addition to being customized to each investor’s unique tastes and goals. As a result of the enhanced possibility for higher returns and reduced risk, AI-powered portfolio optimization has emerged as a crucial tool for investors trying to maximize their financial plans in a competitive and dynamic investment landscape.
Analysis of behavior
Through monitoring news trends, sifting through social media data, and attentively examining investor sentiment, AI systems can extract new insights from market emotions and investor behavior. This data-driven knowledge makes it much simpler to make wise financial choices. By assessing the state of the market and identifying emerging trends or concerns, investors may proactively adjust their approach. This facilitates quick responses and aids in taking advantage of opportunities or avoiding possible risks. Finally, in today’s fast-paced and data-rich financial scene, investors may make more informed and flexible investment choices thanks to behavioral analysis using AI, which provides investors with a powerful tool to measure the mood of the market in real-time.