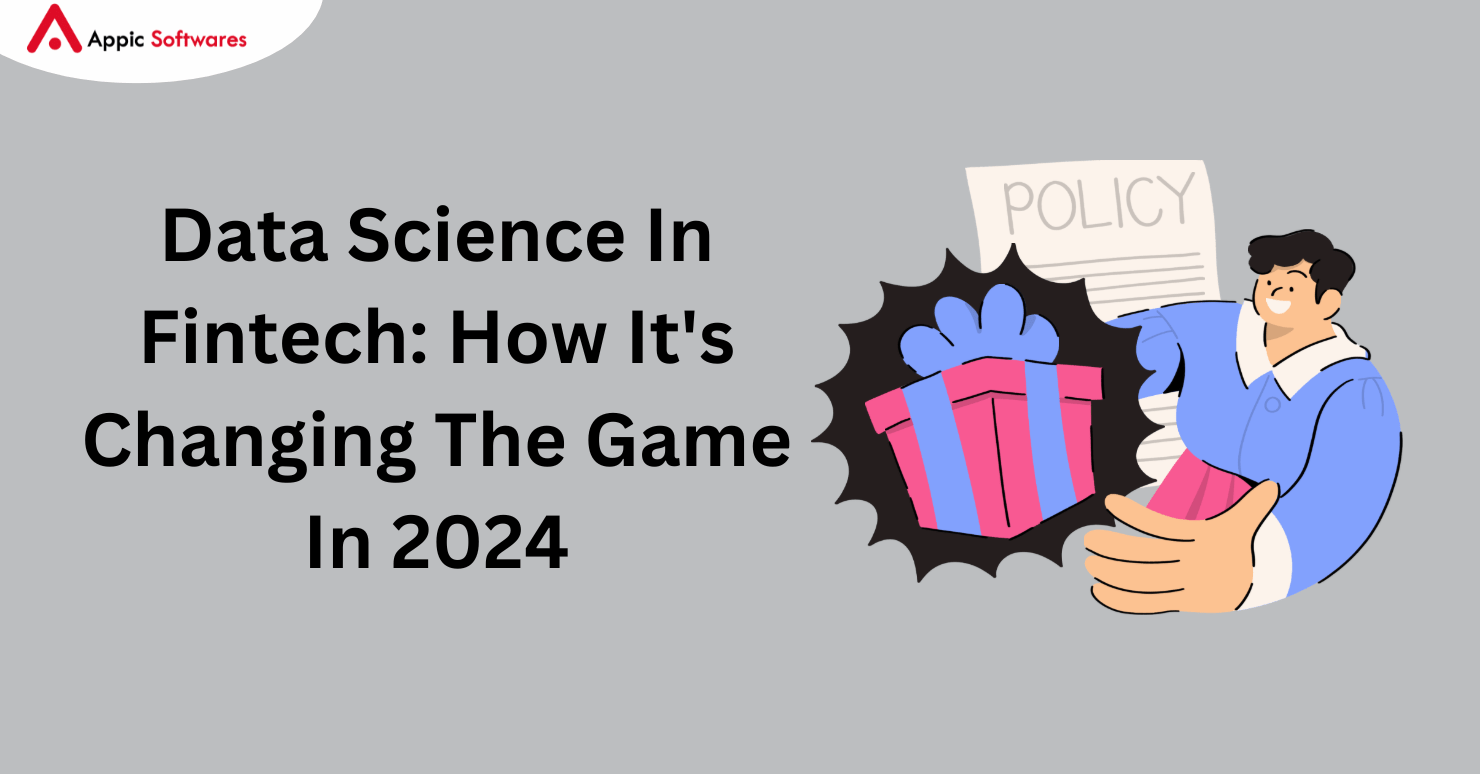
One of the sectors in the world with the quickest rate of growth is financial technology, which is completely changing the way we view money and finance. Financial services organizations now have access to an enormous amount of data, which they are using to manage risk, spur innovation, and enhance client experiences as a result of the rise of fintech. Data science is the process of extracting insights from data using advanced analytics and machine learning. It has become a vital tool for fintech organizations trying to stay ahead of the curve. By using those strategies, businesses can obtain insightful knowledge about market trends, consumer behavior, and possible hazards. This knowledge helps businesses make better decisions and provide clients with individualized financial solutions.
What is data science?
This is a fast-expanding subject that uses sophisticated computational and statistical techniques to draw conclusions and information from data. To evaluate big, complicated datasets, it integrates a variety of methods and resources from disciplines including computer science, mathematics, and statistics.
Fundamentally, data science is about identifying patterns and connections in data and then applying that knowledge to forecast or optimize results. This may entail anything from figuring out what kind of customers they are to spot fraudulent activity or forecasting market movements.
Data scientists employ a range of methods, including predictive modeling, data mining, and machine learning, to do this. To handle and analyze massive datasets, frequently in real-time, they also depend on specialized software tools and computer languages.
The role of data science in Fintech
In the financial and technology sectors, where enormous amounts of financial data are produced and evaluated daily, science is essential. Fintech companies can improve their services, products, and customer experiences by using data-driven decision-making and innovative processes and tools to extract useful insights. Let’s discuss a few of the most important applications of data science in the Fintech industry.
Identification and prevention of fraud
It is used by fintech companies to quickly detect fraudulent activity. Sophisticated machine learning algorithms examine enormous volumes of data to identify odd trends and highlight questionable transactions, thereby lowering the possibility of financial fraud.
Client information
This aids fintech businesses in better comprehending the demands, interests, and habits of their clients. Businesses may find patterns and trends, tailor their products, and enhance consumer experiences by evaluating customer data.
Evaluation of risks
Fintech technologies assess default risk and credit risk by looking at financial documents, payment histories, and credit scores. Companies can reduce financial losses and make better decisions with the help of this study.
Investing and trading
For instance, algorithmic trading makes investment decisions in real time by identifying trading opportunities using sophisticated statistical models and machine learning algorithms.
Robotic advisory
It is used by fintech companies to create robo-advisory systems, which offer automated investing advice according to a user’s risk tolerance and financial objectives. Through the examination of consumer data, such a platform may optimize portfolios and provide tailored investment advice.
Fintech and data science: How they affect the customer experience
Big data has completely changed the financial sector by enabling businesses to gain insightful knowledge and enhance the client experience in a variety of ways. In this section, we’ll go over how data science and fintech specifically relate to customer experience in fintech:
Individualization
Fintech organizations can make customized advice and personalize their offers by analyzing client data. Better client retention and an enhanced customer experience may result from this.
Analytics that predict
Businesses can more easily provide proactive recommendations and personalized experiences, increasing customer happiness, by using big data to forecast customer behavior and preferences.
Increased protection
Security is the main priority for fintech companies because of the volume of financial data they collect. Big data can be utilized to improve security measures and identify fraudulent activity, giving customers peace of mind.
enhanced risk mitigation
Fintech organizations can use it to make more accurate lending decisions and lower risk by using it to assess credit risk and evaluate default possibility.
streamlined procedures
Fintech businesses optimize their workflows, cutting down on task completion times and increasing productivity. Faster service and higher levels of customer satisfaction may result from this.
Instantaneous perceptions
Businesses receive real-time insights into the behavior and preferences of their customers, enabling them to quickly make educated decisions and adjust to the changing needs of their clientele.
Improved openness and appropriate structure
Greater transparency into a customer’s financial actions through the use of big data can increase trust and foster customer loyalty.
enhanced client support
Fintech organizations can pinpoint areas where their customer service needs to be improved by studying client data. The outcomes they should anticipate? quicker problem-solving and higher client satisfaction. Doesn’t it sound good?
Data science advantages for the fintech sector
We’ll look at some of the main advantages of data science in this area, such as real-time insights, fraud detection, and personalized experiences. We’ll also talk about how data science promotes consumer loyalty, enhances customer experience, and fosters corporate expansion.
Better judgment
By using the power of data analysis, businesses may make well-informed decisions based on the examination of large datasets, giving them a competitive advantage. They can achieve greater commercial results and boost profitability with this data-driven strategy. Through the process of extracting important insights from large datasets, businesses can gain insights into developing patterns, comprehend client behavior, and adjust their plans accordingly. Equipped with this understanding, companies may make well-informed decisions regarding product creation, advertising campaigns, and resource distribution, which will ultimately result in enhanced financial outcomes and a more robust market position.
Improved risk mitigation
Because data science can detect fraudulent activity in real-time, it can also aid in the prevention and detection of fraud. Customers are shielded from possible harm and the chance of financial fraud is decreased as a result. In conclusion, data science offers instantaneous perceptions of consumer behavior and inclinations, enabling fintech enterprises to promptly make knowledgeable choices and adjust to evolving client requirements.
Enhanced effectiveness
Additionally, it aids businesses in automating manual chores and streamlining their operations. As a result, businesses increase productivity and efficiency by requiring less time and resources to accomplish tasks.
Individualization
One important use of data science in the finance sector is customer data analysis. Businesses can provide their clients with customized advice and experiences by evaluating customer data. This degree of customization has the potential to boost client retention and loyalty. Fintech organizations can leverage data science to discern consumer preferences and behavior patterns, so enabling them to provide tailored solutions and services that align with their clientele’s requirements. Businesses may stand out in a crowded market and create enduring relationships with their clients by being able to tailor their products.
Identification and prevention of fraud
With the use of complex algorithms and machine learning methods, data science can examine vast volumes of data rapidly and spot unusual or worrisome patterns. Fintech businesses can promptly detect fraudulent actions and take action to prevent future harm to their clients and businesses. This lowers the possibility of financial fraud while also fostering client confidence and trust, all of which are essential for a fintech company’s long-term success.
Instantaneous perceptions
Fintech companies can quickly respond to customer demands and spot patterns thanks to these real-time insights. Fintech organizations can maintain their competitive edge and deliver exceptional client experiences by utilizing data science. The long-term success of any fintech business depends on higher client satisfaction and loyalty, which might result from this.
enhanced client satisfaction
Businesses can boost customer happiness and loyalty by employing data science to tailor goods and enhance customer service. This improves the consumer experience as a whole.
Data Science for Fintech: A summary of the methods employed
In the fintech sector, data science approaches are becoming more and more crucial since they give businesses a competitive edge and enable them to extract insights. Below, we outline some of the most important data science methods utilized in fintech.
Analytics that predict
This is speculating about future events based on historical facts. Predictive analytics is used in the fintech sector to evaluate credit risk, spot possible fraud, and make investment choices.
Machine learning
In this one, the focus is on teaching algorithms to identify patterns and formulate predictions. In addition, it is employed in client segmentation, fraud detection, and credit scoring.
Processing of natural language (NLP)
NLP is the study and application of computer comprehension and analysis of human language. consumer care chatbots, compliance monitoring, and sentiment analysis of consumer comments are a few examples of uses.
Information display
Information is displayed visually using this method, for as through graphs and charts. Trend analysis and simplifying difficult information for decision-makers are two uses for data visualization.
Network examination
Analyzing the connections between things in a network is required for this. Financial transaction trends and possible fraud can be found via network analysis.
group examination
The idea behind this strategy is to combine related data points into groups. Customers can be divided into groups according to their financial preferences and behavior using cluster analysis.
Analysis of time series
Finding trends and patterns in data across time is the goal of time series analysis. Time series analysis is a useful tool in fintech for predicting financial trends and evaluating market volatility.
Analysis of regression
Finding the association between a dependent variable and one or more independent variables is the goal of this technique. Regression analysis can be used to determine the variables that influence financial results, such as investment returns and creditworthiness.
Data science in Fintech: Possible use cases
The article’s most fascinating section is this one! Continue reading to see if your company would benefit greatly from merging some of the use cases from data science and finance.
Identification and prevention of fraud
Data science is used to find possible fraud, including money laundering and credit card fraud. By training machine learning models to identify fraudulent behavior patterns, businesses can intervene before substantial harm is caused.
Evaluation of credit risk
It can also be used to estimate default risk and evaluate creditworthiness. This can lower risk and enable fintech companies to make better-informed loan decisions.
Customized advertising
Fintech organizations may enhance customer engagement and retention by employing data science techniques to examine client data and generate tailored recommendations and offers.
Management of investments
This helps reduce risk and maximize investment returns by analyzing market trends and helping decision-makers make well-informed investments.
Risk Control
Additionally, possible hazards are analyzed and their effects on a company’s operations are evaluated using data science. As a result, companies develop more successful risk management strategies.
Client support
Chatbots, the newest innovation in customer care, are driven by data science and enable businesses to assist clients more quickly and effectively.
Observance
Transactions can be tracked and possible infractions of compliance can be found using data science. This can assist fintech businesses in making sure their operations are compliant with applicable laws and regulations.
Personalized banking goods
Investment portfolios and insurance policies are two examples of customized financial products that can be made with data science. Consequently, this enables businesses to offer customized products and services that cater to the unique requirements of each client.
Conclusion
the 2024 guide on Data Science in Fintech highlights its transformative impact on advanced analytics, risk management, and customer insights. As a game-changer in the financial technology sector, understanding its applications and strategies is crucial for businesses seeking to harness the full potential of data science.
Fintech is always changing, and making an app like an e-wallet requires a lot of careful planning and the newest technology. Using Appic Softwares as a guide gives you a strategy plan to get through the tough parts, which encourages innovation and makes it possible to create groundbreaking fintech apps in 2024 and beyond.
So, What Are You Waiting For?
FAQs
Q. What is the role of data science in fintech?
A: Data science plays a critical role in fintech by analyzing large datasets to detect fraud, predict customer behavior, automate credit scoring, enhance risk management, and improve financial product recommendations.
Q. Which data science technologies are most commonly used in fintech?
A: Key technologies include machine learning, natural language processing (NLP), predictive analytics, AI algorithms, big data tools like Hadoop and Spark, and cloud-based data infrastructure.
Q. How does data science improve fraud detection in fintech?
A: Data science uses pattern recognition and anomaly detection algorithms to identify unusual transactions in real-time, reducing fraud risks and enhancing security across payment and banking platforms.