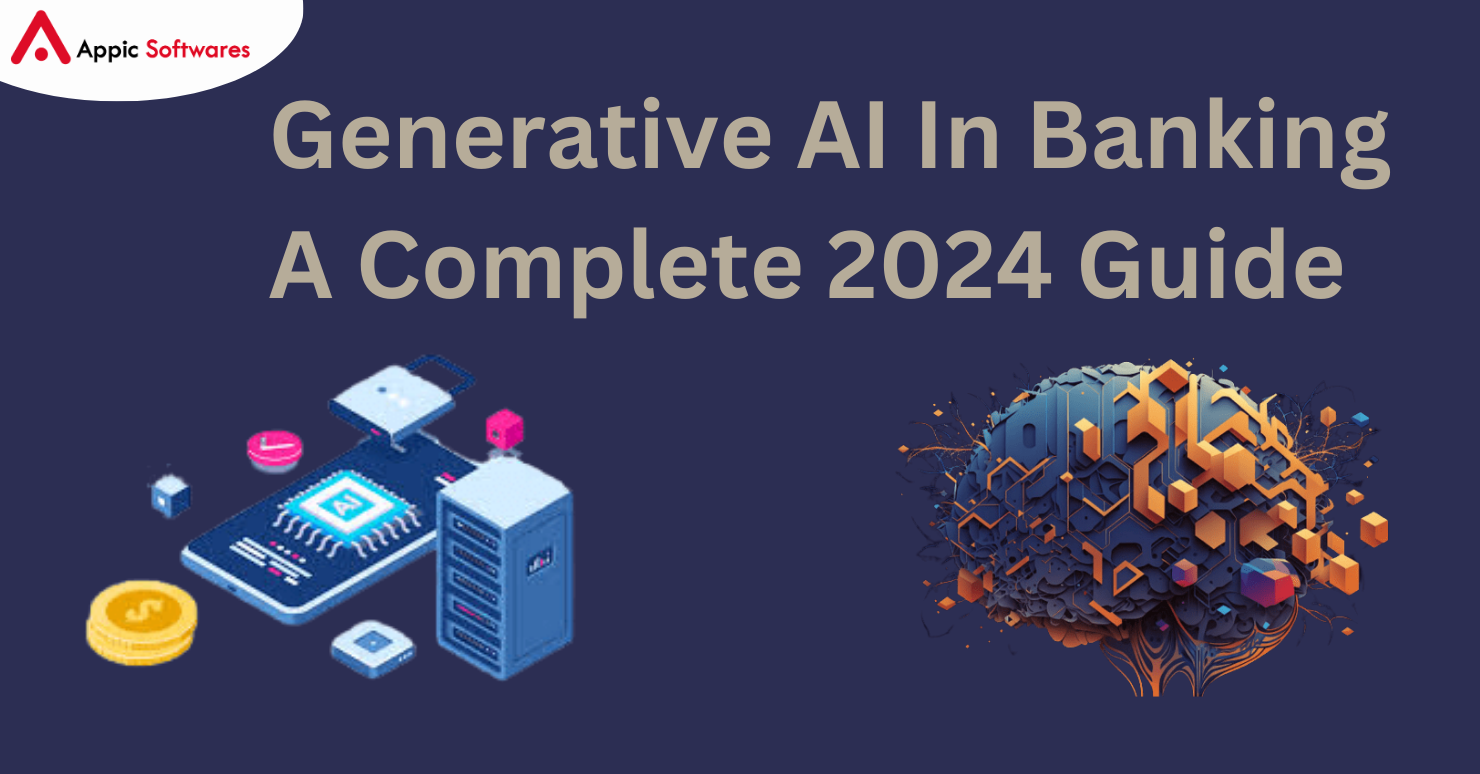
Technology has always disrupted the financial sector, and generative AI is the most recent disruptive force to appear. Generative AI is a cutting-edge machine learning system that can create original material and insights from vast quantities of data analysis. By using financial data at scale, it enables bank management to evaluate risks, offer individualized services, make well-informed decisions, and do much more. Check out this blog to learn more about generative AI in banking.
According to McKinsey, generative AI has the potential to save the banking industry up to $340 billion a year, depending on its adoption. Technology increases banking operations’ efficiency by lowering operational expenses and minimizing human error. More significantly, banks may now engage with customers personally without depending on human staff, thanks to generative AI development services.
The Evolution of AI Is Changing The Financial Industry
In a well-established market, there aren’t many distinguishing elements among financial institutions. The development of AI completely upends that race. Banks have an excellent opportunity to use AI to gain a competitive edge, offer appealing products, and grow their clientele. In a poll, 77% of bankers concurred that an organization’s capacity to survive in the banking sector depends on its ability to fully utilize AI.
The development of cloud infrastructure, storage options, and security technologies enables banks to put policies into action that have a broad effect. The ramifications of adopting AI, especially big language models, will, in my opinion, primarily focus on improving the consumer experience. For instance, Deutsche Bank employs AI to provide customers with investment portfolio management assistance by generating pertinent recommendations based on established risk indicators.
Generic AI enables conversational banking, which makes it simpler to access a variety of financial services and products. These technologies are powered by a machine learning model that has been taught to comprehend and communicate in natural human languages. As a result, banks can increase the range of products they provide while preserving the accuracy and accessibility of their information for various regional audiences.
The automated elements that AI adds to the traditional banking procedure will also help banks. AI enables banks to respond to consumer questions and address problems around the clock via a variety of channels. In this approach, AI helps human support staff by providing answers to frequently asked inquiries, freeing them up to concentrate on more complicated issues.
Applications of Generative AI in Banking
There are many opportunities for personal engagement in the banking sector. And this offers generative AI a ton of chances to make meaningful improvements. I provide several real-world examples below.
Chatbots
The majority of banks already use chatbots to answer client inquiries. Bankers may use big language models that can have human-like conversations thanks to generative AI. Customers may obtain fast assistance by asking questions like “How much is my balance” or “Change my billing address” rather than having to navigate through a menu of options.
Portfolio Administration
Banks may now provide consumers with more granular portfolio strategy recommendations thanks to generative AI. The deep learning algorithm uses a lot of economic data to train initially. Bankers then use the AI system to forecast future trends based on a variety of varying financial variables, such as inflation, political dynamics, and currency rates, to create a suitable portfolio. This creates a more welcoming and private banking atmosphere by not asking clients to publicly reveal their financial situations.
Compliance Supervision
Banks operate in a highly regulated sector and are under continual pressure to adhere to strict standards. This entails keeping an eye on transactional activity, compiling pertinent data, and timely presenting it to the appropriate departments. The load of compliance management will be reduced by using an AI system educated on these procedures. For instance, before authorizing an account, bankers utilize generative AI to examine client data and make sure they abide by the Know Your Customer (KYC) Act.
Forecasting and Analysis of Finance
Banks have to plan and have a solid position in the face of changing market conditions, just like other businesses. They can do simulations, forecast economic trends, and modify their positions using generative AI. Banks, for instance, may use AI to anticipate the pace of inflation over the medium term and modify interest rates accordingly.
Advisor for Finances
Banks may now engage with each consumer equally and individually thanks to generative AI. Before recommending items that the consumer might find interesting, the deep learning model examines their past purchases, risk tolerance, and spending patterns. Both sign-up rates and client retention are boosted by this.
AI-Powered Fraud Identifiers
Banks are under regulatory pressure to protect clients’ interests and stop fraudulent activities as a result of the widespread data breaches. Large amounts of financial transactions may be used to teach generative AI to spot unusual patterns and trigger instant alarms. This enables banks to stop questionable transactions and preserve their clients’ confidence.
Management of Loan Scores
When deciding whether to approve or deny a loan application, bankers consider several factors. Generative AI analyzes the applicant’s past financial transactions and current information to help with credit scores. For instance, by evaluating the applicant’s income, age, employment, residence, and other credit indicators, you may train the machine learning model to forecast the possibility of a default.
Automation of Operations in the Back Office
To run back-office functions like document scanning, employee identification verification, and networking infrastructure security, banks make significant staff investments. Operational personnel bear less of the workload when generative AI is incorporated into the workflow. For instance, they may scan, analyze, and organize physical documents in safe cloud storage using NLP software.
Generating Financial Reports
Machine learning models that can display organized data are the foundation of generative artificial intelligence. This enables the automatic generation of financial statements on demand by banking AI systems. Customers can request personalized income or cash flow reports, for instance, and the AI can quickly combine these into files.
Upkeep of Legacy Software
Some banks continue to operate using software written in antiquated programming languages. Developers construct the code using generative AI and the underlying big language models, rather than starting from scratch. This lowers human mistake rates and increases coding productivity when transferring software to a newer programming framework.
AI’s Advantages For The Banking And Finance Sector
After incorporating AI into both customer-facing and back-end operations, banks have already experienced significant gains. Included in them are:
Improved Judgment
Bankers, fund managers, and other financial players use AI-enabled insights to support their decisions. In erratic market situations, they employ AI algorithms to minimize risks and optimize possibilities.
Individualized Interactions With Clients
Consumers no longer have to wait a long time for assistance or receive generic product pitches. Rather, they receive timely attention and always have access to customized information via websites, apps, and other digital platforms.
Enhanced Effectiveness
Financial organizations can expand operations and overcome obstacles preventing manual procedures with the help of AI technology. By automating repetitive and resource-intensive processes using AI technology, banking employees can provide greater value to their consumers.
Stronger Security
Financial institutions use AI to safeguard their clients’ data. In addition, AI enables banks to detect and thwart fraudulent activity in real-time, keeping them flexible in the face of cyberattacks.
Enhanced Risk Mitigation
Using its analysis of the large amount of available financial data, AI helps banks better reduce risks. Bankers use predictive insights to safeguard assets, overcome obstacles, and seize market opportunities rather than make snap decisions.
AI-Supported Adherence To Regulations
Banks regularly evaluate their compliance with regulations using AI. AI technologies help banks cut expenses and stay out of trouble by automating jobs that bank officials used to do.
Enhanced Privacy
With AI, customers feel more secure and at ease while discussing financial possibilities. After the session, they can ask for the full deletion of all conversational data and opt not to provide any personal information.
Why Generative AI Is Limited in Banking and Finance?
The potential benefits of generative AI for banks and lending institutions are undeniable. However, the financial use cases of generative AI need the industry to take particular care and handle certain issues.
Data Integrity
Access to substantial amounts of superior training data is necessary for banks to scale their generative AI applications. Deep learning models, also known as foundational models, need to be trained before they can be used for tasks unique to banking. The final AI system will not function as intended if the training data is not sufficient in terms of relevance, accuracy, completeness, or size.
Security And Privacy
A significant quantity of data must be moved and stored over the network infrastructure to train generative AI models. Financial limitations might prevent banks from using particular types of data for deep learning model training. When generative AI systems are being trained or implemented, banks also need to consider data vulnerabilities to safeguard the privacy of their clients.
Prejudice
A problem known as bias arises when there is insufficient data for the model to be trained on. A biased model cannot guarantee accurate results. For instance, if an application doesn’t have enough data for a particular demographic, an AI credit scoring system may reject it. These choices are unjust and might damage the bank’s standing.
Numerical Accuracy
For the time being at least, generative AI is not intended for use in mathematical computations. When calculators conduct mathematical operations on preexisting operations, they adhere to certain restrictions. In the meanwhile, fresh responses are produced by generative AI models; these may or may not be accurate. Therefore, it makes sense to implement safety measures, such as human approval, to stop inaccurate data from having an impact on banking operations.
Generative AI’s Future in Banking
Bank operations and client interactions will change as a result of generative AI. With ChatGPT, we can already observe how generative AI is affecting a wider range of customers.
By typing particular prompts, users of ChatGPT may write computer codes, compose lyrics, tell stories, and much more. Similar use cases will also be seen in the banking sector, although they will be repurposed for financing activities.
When generative AI is deployed throughout the organization, bankers and clients will profit from its efficiency and customization. However, banks must first have a sound plan for implementing AI.
How Can Banks Change To Become Leaders in AI?
To fully utilize generative AI, banks need to make sure they can expand machine learning technology throughout the whole business. Rather than continuing to operate in silos, banks need to combine several divisions and provide employees with the knowledge, attitudes, and abilities needed to use artificial intelligence. To keep current with evolving AI technology, for instance, banks reskill their workforce or employ AI specialists.
Then, to enable AI systems, banks need to reevaluate their infrastructure and technological prowess. A significant quantity of data must be securely stored to train and use generative AI models. Banks also need to think about whether their current technological skills are enough and whether they can integrate their own AI capabilities with outside offerings.
Banks use generative AI models embedded in their infrastructure to facilitate decision-making across several domains. These steps ought to be practical, including accurate risk assessment and ongoing AI model improvement. For instance, they use AI to enhance manual or labor-intensive procedures like loan approval or customer service.
For banks to truly benefit from AI, they must reconsider their approaches to consumer interaction. Rather than concentrating on certain items, banks have to reconsider how AI may be utilized to enhance client value. For instance, Tally helps users manage credit card payments and quickly pay off debt by using AI algorithms.
Advice on Applying Generative AI in Banking
Though there is hope, there are still several obstacles in the way of deploying generative AI in banking use cases. I offer various strategies for easing these kinds of adjustments.
Determine which particular banking procedure stands to gain the most from generative AI. To enhance customer service, for instance, you may train an AI chatbot. Later, you can extend the technology to important use cases such as credit scoring.
Make sure the right data protection measures are in place to preserve client privacy and adhere to industry standards. For instance, generative AI protects against misuse by encrypting data in case it is accidentally disclosed.
The caliber of training datasets has a major impact on how well the generative AI model performs. Thus, ensure that the data are large enough to represent the desired demographics and that they are labeled correctly.
Since generative AI is still developing, it should be used with caution when doing exact arithmetic computations. Install the necessary safeguards to prevent inaccurate results from having an impact on consumers.
A generative AI model works by calculating in response to cues. Use particular consumer financial data, such as prior transactions, financial objectives, and risk tolerance, to trigger the model and get relevant results when utilizing it to customize the banking experience.
Conclusion
Banking will no longer provide consumers with impersonal, inefficient services thanks to generative AI. If executed properly, it has the potential to significantly reduce costs for financial institutions as well. Generative artificial intelligence (AI) plays an enormous role in the banking sector, whether it is used to detect fraudulent transactions or augment customer assistance with chatbots.
However, there are still difficulties in using generative AI widely, especially in a highly regulated sector. This is where Appic Softwares AI experience is useful. We have assisted businesses such as Plai in incorporating generative AI into their current offerings. We have an experienced team of in-house Fintech App Developers who can help you in building a Generative AI Fintech Application.
So, What Are You Waiting For?
FAQs
Q. What is generative AI in banking?
Generative AI in banking refers to the use of AI models like ChatGPT or custom LLMs that can generate content, automate conversations, analyze financial data, and support decision-making. It’s transforming how banks interact with customers and manage operations.
Q. How is generative AI different from traditional AI in banks?
Traditional AI focuses on automation and data analysis, while generative AI can create new content like emails, reports, or chatbot replies and personalize services in real time. It’s more conversational, creative, and context-aware.
Q. What are the key applications of generative AI in banking?
Generative AI can be used for virtual customer support, fraud detection explanations, personalized financial advice, document generation, risk modeling, and automating back-office tasks.