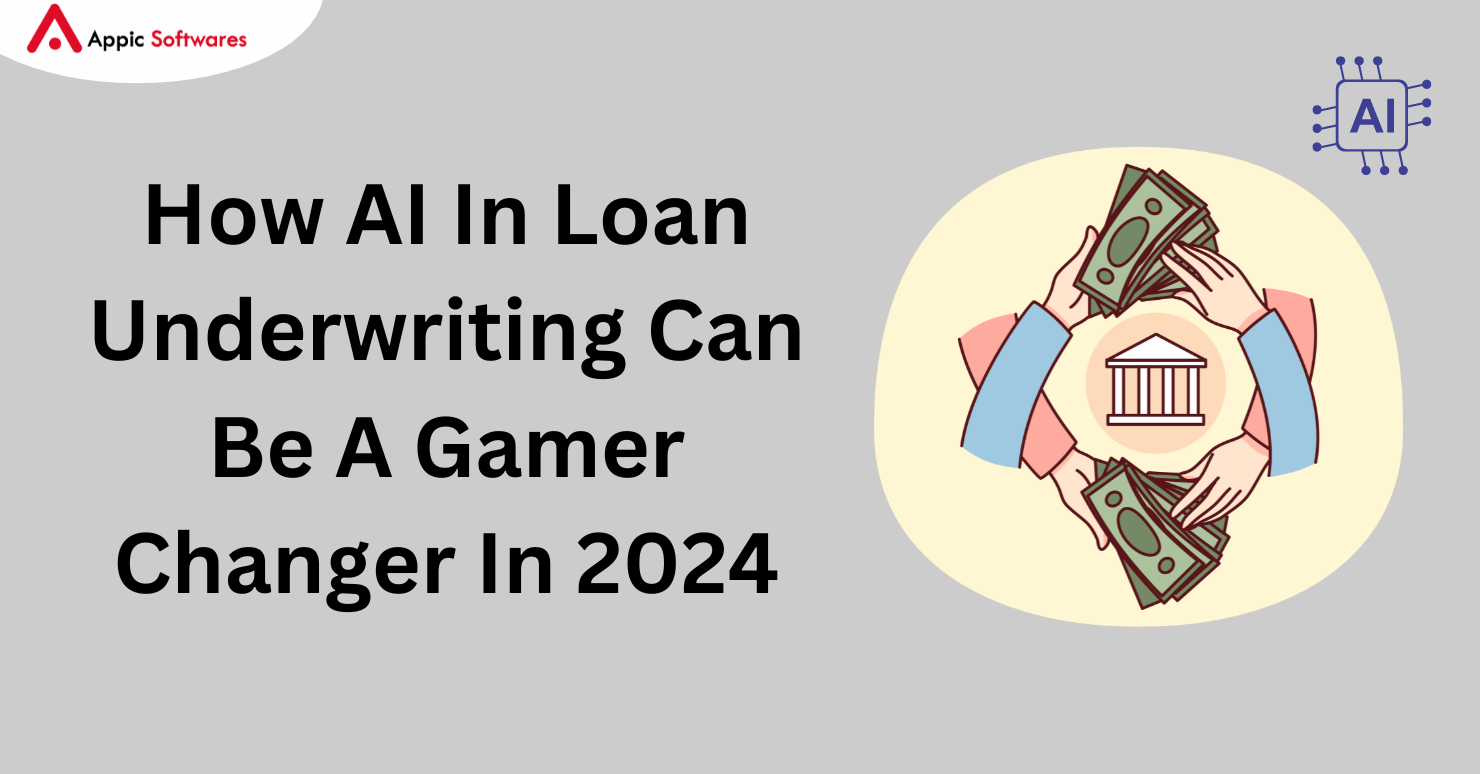
Financial institutions have a wide range of difficulties in the complex field of loan underwriting, which may hinder the speed and accuracy of the loan sanctioning procedure. Conventional underwriting techniques often struggle with laborious chores, intricate data processing, and the possibility of human mistakes, which cause delays and raise operating expenses. The industry has undergone a paradigm shift as a result of the demand for a more efficient and technologically sophisticated approach, and artificial intelligence (AI) has emerged as a disruptive force that has the potential to completely change the loan underwriting environment.
A major challenge for underwriters is the laborious task of gathering, confirming, and examining enormous volumes of borrower information. In addition to taking a long time, this manual method puts the lender at risk of missing important facts or computing risk factors incorrectly. Moreover, the dynamic character of financial markets necessitates an agile and flexible underwriting procedure, an accomplishment that traditional approaches find challenging.
AI is a technical force to be reckoned with; it uses machine learning algorithms to analyze massive datasets quickly and precisely. By automating routine processes like data collecting and risk assessment, artificial intelligence (AI) in loan underwriting drastically lowers the time and resources needed for the process. Artificial intelligence (AI)-driven underwriting systems improve prediction accuracy by continually learning from past data patterns and adjusting to changing market dynamics. This enables financial institutions to make better-informed judgments more quickly.
This paper explores how machine learning algorithms strengthen risk assessments, speed loan approval procedures, and improve data processing, highlighting the revolutionary impact of AI in loan underwriting. The essay will highlight the creative solutions artificial intelligence (AI) brings to the fore, bringing in a new age of efficiency, accuracy, and agility in loan sanctioning by illuminating the difficulties encountered by conventional underwriting techniques.
What is loan underwriting?
The process by which a financial institution determines a borrower’s creditworthiness and the level of risk involved in making a loan to them is known as loan underwriting. The lender examines several financial factors during underwriting, such as the borrower’s credit history, income, job stability, and debt-to-income ratio. The goal is to determine the likelihood that the borrower will be able to repay the loan while taking into account their financial situation. Lenders utilize underwriting to determine the terms and circumstances, including interest rates and loan amounts, and to make well-informed choices on whether to accept, alter, or refuse a loan. Ensuring safe lending practices and risk management depend heavily on the underwriting process.
Challenges and limitations in traditional loan underwriting
Under conventional loan underwriting, a borrower’s creditworthiness and ability to repay a loan are thoroughly assessed. Nonetheless, these conventional underwriting techniques come with several drawbacks. Here are a few of them:
- Time-consuming procedure: Conventional underwriting procedures may take a long time, requiring a lot of paperwork and a manual examination of financial records. This may cause delays, which would make it difficult for borrowers to get money fast.
- High cost: Traditional underwriting procedures’ manual nature raises the operational expenses of lenders. This might lead to increased interest rates or fees for borrowers and decreased profit margins for lenders, which could be a constraining factor for both parties.
- Restricted data sources: Credit scores and income statements are only two examples of the financial data that traditional underwriting techniques often depend on. This may not provide a comprehensive picture of a borrower’s financial situation and ability to repay a loan, especially for individuals with spotty credit or inconsistent income.
- Subjectivity: Traditional underwriting relies heavily on human judgment, which may lead to subjectivity and inconsistent decision-making. Biased judgments or unfavorable results might result from this.
- Risk of fraud: Since it might be difficult to properly check the legitimacy of all provided papers, manual underwriting procedures are vulnerable to fraudulent activity.
- Lack of flexibility: Conventional underwriting techniques may not be able to adjust adequately to shifting market conditions or particular financial circumstances. This rigidity may hinder the evaluation of non-traditional borrowers or those experiencing transient financial difficulties.
- Incapacity to correctly evaluate non-traditional sources of income: Non-traditional sources of income, such as rental income, gig labor, or freelancing, may be difficult for standard underwriting techniques to evaluate. This restriction may affect some people’s eligibility for loans.
- Restricted use of technology: A lot of human data input and antiquated methods are still used in many conventional underwriting procedures. Inadequate technology utilization may impair productivity, precision, and the capacity to use machine learning and sophisticated analytics.
- To improve the efficacy and efficiency of loan underwriting procedures, the financial sector is progressively investigating and using cutting-edge technologies including artificial intelligence (AI), machine learning, and alternative data sources. These developments are meant to improve efficiency, save expenses, and provide a more precise evaluation of a borrower’s creditworthiness.
An outline of artificial intelligence in loan underwriting
Understanding artificial intelligence (AI) in loan underwriting requires an awareness of how it has transformed the conventional lending environment. By using cutting-edge machine learning algorithms and data analytics, artificial intelligence (AI) is revolutionizing the underwriting process and enabling more effective and knowledgeable credit choices. Unlike conventional techniques, AI-enabled underwriting examines large datasets quickly and incorporates information from non-traditional sources like as transaction histories, social media activity, and behavioral patterns. This feature makes it possible to assess a borrower’s creditworthiness in a more detailed and nuanced manner, which is particularly helpful for those with short credit histories. AI-driven models also reduce human bias in decision-making, improve accuracy, and are flexible to shifting economic situations. By allowing for a more comprehensive evaluation of applicants, the use of AI in loan underwriting not only expedites procedures but also encourages wider financial inclusion.
Use cases of AI in loan underwriting
AI is revolutionizing several sectors, and loan underwriting is no different. These are a few significant applications of AI in loan underwriting:
Evaluation of creditworthiness
One important use of AI in loan underwriting is the way automated credit decisioning systems are changing the commercial lending industry’s traditional dependence on strong credit ratings. Modern lenders are breaking with tradition and using AI-powered alternative scoring algorithms to automatically assess a customer’s creditworthiness. Using consumer bureau data and AI-based credit scoring, this revolutionary method quickly compares credit scores to predetermined benchmarks to determine eligibility. Personalized credit offers are delivered to qualified clients; if accepted, a faster onboarding procedure includes digital client onboarding and automated KYC. The increasing need for a quicker and more responsive lending environment is met by this integration of AI, which not only speeds up credit evaluation but also guarantees quick loan disbursal. This use case demonstrates the continuous innovation and investment in commercial lending and is in line with the modern customer expectation of a quick and easy credit evaluation and disbursement process just when the customer needs it.
Improving the clientele’s experience
AI integration in banking and credit lending extends beyond conventional responsibilities, turning institutions become financial friends for their customers. Banks are using artificial intelligence (AI) to provide a more engaging client experience in addition to providing typical financial goods like loans and instruments. The first step in AI’s job is to create comprehensive, data-rich client profiles. These profiles are created by carefully going over transaction line items on current accounts or credit card bills.
Banks go beyond the conventional business-to-business exchange and into the domain of customized financial relationships. Thanks to this progress, they can now provide customized assistance on saving, big purchases, financial literacy, and critical decision support. Banks can improve their conventional services and significantly improve the lives of their clients by using AI. Digital lending platforms use data to do more than just process financial transactions; they allow banks to tell certain consumers about special offers and discounts that are linked to their credit history. In addition to creating a more engaging banking environment, this proactive strategy simplifies loan operations and creates a smooth, customized experience for clients.
streamlined the loan application procedure
Artificial Intelligence (AI) in loan underwriting is revolutionizing business lending by providing a digitally driven, smooth experience similar to personal loan applications. The full digitization of lending is still impacted by certain legal restrictions, such as mortgage loans and payments, however, there is a rising movement in the banking industry to automate at least 95% of human underwriting decisions.
Traditional banks and fintechs are giving careful consideration to digitalization when it comes to corporate lending, especially when it comes to SME credit. Instead of starting from scratch with every client interaction, banks are improving regular procedures bit by bit. For example, they are digitizing credit proposal documents and automating yearly reviews to increase productivity.
Additionally, strategic efforts are in motion to automate low-risk credit renewals to support corporate transaction approvers. This guarantees that important human resources may be allocated to complex, credit-risky transactions. Relationship managers now have immediate access to full data and risk-evaluation scores that take into account market and sentiment research, financial and industry performance, significant news, and external variables thanks to artificial intelligence’s involvement in automating data aggregation. In addition to streamlining procedures, this also gives firms the ability to make lending judgments quickly and accurately, which represents a major advancement in the field of commercial loan underwriting.
Remedial of data in loan applications
In the context of loan underwriting, addressing data quality—more especially, the precision, thoroughness, and dependability of personal, financial, and credit-related information in loan applications—is a crucial area of attention for AI technology. Taking note of this, banks and lending organizations have implemented extensive initiatives that give top priority to quality analysis, data measurement, and fixing errors that are found. On the other hand, conventional remediation procedures often prove to be laborious, resulting in considerable operational backlogs and necessitating a large amount of human resources for data cleansing.
This environment is redefined by the introduction of AI intervention and technology integration, which provide more effective methods for identifying and resolving problems on a larger scale. A certain amount of money is set aside for the automation of remediation procedures and the deployment of AI-integrated applications. To facilitate the creation of analytics-driven models that guarantee quicker and more accurate judgments throughout the data cleanup process, validated machine learning models are deployed to examine inputs. This thoughtful use of AI offers a revolutionary method for managing data quality in the context of loan applications, speeding up data remediation processes while also improving accuracy.
enhancing loan underwriting to prevent fraud
Strong fraud monitoring in loan underwriting is required due to the rise in cybercrimes during the digital revolution, and artificial intelligence (AI) has become a crucial ally in this field. With ransomware attacks, phishing schemes, identity theft, and data theft on the rise, the combination of blockchain technology with artificial intelligence (AI) is revolutionary.
Artificial Intelligence (AI) transforms identity verification for loan borrowers using blockchain-powered smart contracts, guaranteeing speed and security. By monitoring and controlling loans at every stage of their lifespan, these smart contracts reduce the likelihood of defaults, late reporting, and unreported loans. Artificial intelligence (AI)-driven solutions reduce the possibility of fraud by providing a shared control perspective over historical records, authenticating financial data and information, and facilitating shared access and consensus. This guarantees effective governance and improves underwriting process transparency. It is crucial to have a strong and long-lasting technology basis as more banks and businesses adopt an “AI-first” strategy. Rapid technical improvements come with a high danger of fraud and inadequate security. Thorough security testing is thus essential to protect against possible dangers and weaknesses before the implementation of creative AI solutions for the benefit of society as a whole.
OCR-based data extraction for loan underwriting
As a specialized answer, optical character recognition (OCR) appears. It decodes textual information inside photographs and enables a revolutionary method of data extraction. With its smooth conversion of text-containing photos into computer-readable characters, optical character recognition (OCR) is influencing financial organizations’ future strategies. Banks and commercial lending companies use optical character recognition (OCR) to read important information from hard copy documents like checks, including account details, handwritten amounts, and authorization signatures. Loan applications with a variety of documents and data experience a faster, more efficient data extraction procedure thanks to artificial intelligence technology. This is improved by deep learning, which uses OCR to extract more data points and provide more thorough results. This highlights the efficiency advantages possible via the incorporation of OCR technology and speeds up data capture and maintenance as well as decision-making in the loan underwriting process.
Using behavioral Analytics for loan underwriting
Advanced behavioral analytics is a game-changer, revolutionizing the way fintech companies and banks handle transactions and extract knowledge from main operational accounts. Advanced behavioral analytics goes beyond the painstaking classification of transactions into specific revenue and cost categories that may be achieved with operational instruments. Banks and other institutions may create streamlined financial statements, assess affordability ratios, and do real-time supplier and customer concentration assessments by using behavioral analytics. The abundance of transactional data not only offers up-to-date insights but also forms the basis for more richer and more practical data solutions, which in turn creates the framework for iterative models of data improvement. A standardized behavioral analytics model serves as an example of how AI-driven insights improve the effectiveness and accuracy of loan underwriting procedures, as well as a driver for innovation in customer experiences and the improvement of the lending process.
Financial institutions may improve efficiency, lower risks, and make better lending choices by using AI in loan underwriting. This would eventually benefit lenders as well as borrowers.
The operational advantages of AI in loan underwriting
Numerous advantages result from integrating AI into loan underwriting procedures, which redefines the efficacy, accuracy, and efficiency of the lending sector as a whole:
- Enhanced speed and efficiency: AI automates laborious underwriting procedures, greatly accelerating the decision-making process. Quick reactions to borrower applications are guaranteed by real-time data analysis capabilities, which make the lending process more flexible and effective.
- Enhanced precision and risk evaluation: Artificial intelligence-driven algorithms make use of large datasets to identify trends and evaluate risk variables, which leads to increased precision in estimating creditworthiness. Financial institutions may make more informed loan choices because of this increased accuracy, which reduces the chance of defaults.
- Personalized decision-making: Lenders can make judgments that are specific to each borrower because of AI’s customization capabilities in underwriting. To maximize the lending experience for a wide range of clients, this customization also applies to interest rates and loan conditions.
- Cost savings: AI dramatically lowers the running expenses related to underwriting procedures by automating manual work. An efficient use of resources results in a lending operation that is more affordable and long-lasting.
- adaptation to dynamic situations: Underwriting models stay flexible to changing market trends thanks to AI’s real-time adaptation to shifting economic conditions. AI’s built-in continuous learning processes provide a flexible and dynamic strategy in the face of changing economic conditions.
- Improved fraud detection: AI uses complex algorithms to spot irregularities and spot possible fraud in loan applications. This increased capacity for preventing fraud makes lending environments safer for both borrowers and financial institutions.
- Streamlined document processing: Artificial Intelligence (AI) uses Natural Language Processing (NLP) and Computer Vision to handle documents and unstructured data in loan applications more quickly. This reduces the need for human labor by improving accuracy and speeding up the processing of documents.
- Enhanced objectivity and consistency: The rule-based functioning of AI lessens the influence of human prejudice, promoting increased objectivity in loan selections. Applying preset algorithms consistently guarantees equity at every stage of the decision-making process, encouraging a more unbiased methodology.
- Scalability: AI-driven systems are scalable because they can effectively handle massive amounts of data and applications. For financial institutions managing growing loan portfolios, this scalability is especially helpful since it guarantees operational efficiency as the company expands.
- Data-driven insights: AI’s data analytics skills provide insights that can be put into practice, giving lenders important information for making well-informed decisions. Financial institutions may better understand market trends, hone their underwriting techniques, and maintain their competitive edge with the help of this data-driven insight.
- Improved customer experience: The loan process may be made more enjoyable for customers by providing tailored services and quicker processing times. Effective and transparent underwriting builds borrowers’ confidence and strengthens the bonds that bind financial institutions to their customers.
- Regulatory compliance: By ensuring adherence to regulations, AI systems may be built with a lower chance of mistakes. Automated procedures improve compliance with legal standards with greater accuracy, reducing regulatory risks for financial organizations.
In addition to solving the shortcomings of conventional approaches, the application of AI to loan underwriting brings about several advantages that raise the efficiency and client happiness of the lending sector as a whole.
AI technologies transforming loan underwriting
Artificial Intelligence (AI) technologies are essential for streamlining loan underwriting procedures and increasing their effectiveness. The following major AI technologies are often used in loan underwriting:
Artificial Intelligence (AI)
By using sophisticated algorithms and data analytics, machine learning (ML) is a key component in the modernization of loan underwriting procedures. ML algorithms are trained on large datasets for loan underwriting to identify trends, evaluate risk factors, and forecast creditworthiness. These algorithms are capable of examining a wide range of information, such as income, credit history, and financial activity, to provide a more comprehensive assessment of borrowers. Real-time decision-making is made easier by machine learning (ML), which helps financial institutions increase the efficiency of the underwriting process, increase the accuracy of risk assessment, and respond quickly to changing market circumstances. ML models improve efficiency and reactivity in the dynamic world of loan underwriting by constantly learning and developing, which helps lenders make better judgments.
Analytics that predict
A key component of contemporary loan underwriting is predictive analytics, which uses data-driven insights to improve decision-making procedures. Predictive analytics in this context makes use of statistical algorithms and historical data to forecast future trends and evaluate possible borrower risks. Predictive analytics helps financial organizations anticipate default probability, assess creditworthiness more precisely, and optimize interest rates by examining patterns and correlations within large datasets. Lenders are now able to proactively manage risks, adjust to shifting market circumstances, and make well-informed judgments thanks to technology. In the end, the use of predictive analytics in loan underwriting results in a more accurate, effective, and proactive strategy that better aligns financial institutions with the changing demands of the lending market.
Processing of Natural Language (NLP)
In the field of loan underwriting, natural language processing (NLP) is revolutionary because it makes it possible to analyze and comprehend unstructured data, like text. In this instance, NLP algorithms extract useful insights by processing and comprehending data from a variety of sources, such as social media, financial documents, and loan applications. NLP helps to speed up and increase the accuracy of underwriting procedures by automating the extraction of pertinent information. Through its ability to understand the subtleties of language and context, natural language processing (NLP) helps to improve risk assessments, facilitates more thorough due diligence, and expedites the loan underwriting decision-making process, all of which lead to a more advanced and effective method of credit evaluation.
computer vision
Although it is not used as often in loan underwriting as it is in other domains, computer vision can automate document analysis. In this situation, information may be visually interpreted and extracted from documents like bank statements, identity cards, and financial records using computer vision algorithms. With the use of this technology, physical documents may be processed and digitized more quickly and accurately while also requiring less human labor. Computer vision may improve document verification procedures, leading to more accurate and dependable borrower information evaluations, even if its use in loan underwriting is still in its infancy.
Lenders may expedite underwriting procedures, increase accuracy, reduce risks, and, ultimately, make well-informed judgments when giving credit to borrowers by using these AI technologies. When using AI for loan underwriting, it’s essential to take regulatory compliance, data privacy, and ethical issues into account.
How does AI/ML-driven automated loan underwriting work?
AI/ML-powered automated loan underwriting follows a methodical procedure that maximizes accuracy and efficiency. This is what the process usually looks like:
Data gathering
Obtaining relevant information from the borrower is the first step in the process. This includes a wide range of information, including work history, income information, credit scores, and other financial measures. In the era of digitalization, this information is effectively gathered electronically by using document management systems and often by adding electronic signatures, or e-signatures.
Preparing the data
Following the collection of the necessary data, a crucial pre-processing stage is undertaken. This stage guarantees that the data is appropriately structured and prepared for in-depth examination. It can include normalizing the dataset, cleaning up the data, and adding any missing numbers. To guarantee that the data is appropriate for analysis by the ensuing AI and ML algorithms, this first step is essential.
Data interpretation
The automated underwriting process is based on the analysis of the gathered data using AI and ML algorithms. These algorithms examine the data for trends and risk indicators, which helps them determine how creditworthy the borrower is.
Evaluation of risks
Based on data analysis, artificial intelligence (AI) and machine learning (ML) algorithms—which are often backed by financial models—calculate the borrower’s risk level. The person is given a risk score, which is a crucial component in determining whether or not they are eligible for a loan.
Making decisions
The risk score is used by the automated decision-making system to determine the loan application. This procedure may take place instantly, allowing lenders to notify borrowers of the progress of their loan applications. Robo-advisors are used in some situations to automate the process of giving borrowers financial advice and suggestions.
Verification
An automatic validation mechanism that confirms the veracity of the data given by borrowers adds an extra degree of confidence. This stage guarantees the accuracy and dependability of important elements including income and employment data.
Acceptance or rejection
In the last phase, the loan is either approved or denied by the system. This choice is based on the outputs from the decision-making mechanism as well as the combined outcomes of the data analysis. Loan approval turnaround times are shortened and efficiency is increased by this automated procedure.
Issues and worries related to loan underwriting powered by AI
While AI-driven loan underwriting has many advantages, there are several drawbacks as well that should be carefully considered. The following are some of the main issues and difficulties with AI-driven loan underwriting:
Bias and ethical concerns
It is difficult to ensure fairness in AI models because they could inadvertently reinforce prejudices existing in past data. It is feared that these biases may result in unequal loan results for certain socioeconomic or demographic groups, bringing ethical questions regarding how this may affect equity and inclusion in the lending process.
Openness and comprehensibility
One issue is that complicated AI models are opaque, which makes it difficult for stakeholders to understand the decision-making process. When there are unclear justifications for lending choices, there is a risk that the credibility of regulators, financial institutions, and borrowers may be eroded. This opacity might prevent AI-driven underwriting procedures from being widely understood and accepted.
Security and privacy of data
There is a problem with data privacy and security when large volumes of sensitive personal and financial data are used. It is feared that insufficient security might result in breaches, putting private data at risk of abuse and illegal access. This emphasizes how crucial it is to have strong data security protocols in place before using AI for loan underwriting.
Perception and trust among customers
Building confidence in AI-driven systems is a difficulty because of apprehensions about responsibility, justice, and algorithmic opacity. The worry is that people may become less trusting of AI-driven financial services, which might prevent more people from using this cutting-edge technology in lending.
Validation and resilience of the model
It may be difficult to guarantee the resilience of AI models, especially when there are situations that aren’t included in the training set. The worry is that insufficient model validation might lead to erroneous risk evaluations, which could cost lenders money. Thorough validation procedures are necessary to establish trust in the dependability of AI-powered underwriting models.
While using AI to loan underwriting presents previously unheard-of levels of efficiency and accuracy, financial institutions must recognize and resolve the issues and obstacles that this technology entails. To fully realize the transformational potential of AI-driven underwriting, it is imperative to mitigate ethical concerns, improve transparency, and prioritize data protection. This will ensure a balanced approach that harmonizes technical innovation with moral and legal requirements.
The future of AI in loan underwriting
As technology advances and financial institutions adopt cutting-edge solutions, the future of artificial intelligence in loan underwriting is full of promising opportunities. Key elements influencing the future terrain are as follows:
- Increased use of alternative data: Outside of conventional credit histories, financial institutions will employ more and more other data sources. A more complete picture of an applicant’s financial conduct may be obtained by looking at non-traditional indications such as internet activity, social media engagement, and other behaviors.
- Constant regulatory adaptation: To handle the unique difficulties posed by artificial intelligence in loan underwriting, regulatory frameworks will change. Governments and regulatory organizations will work together to develop regulations that provide a framework that balances using AI’s advantages with preserving privacy, consumer rights, and fair lending practices.
- A greater emphasis will be placed on education and awareness: As the use of AI in loan underwriting grows, a deliberate effort will be made to inform all relevant parties, including borrowers, about its use. Building confidence in AI-driven financial systems will need open communication and awareness campaigns.
- Advances in explainable AI: The problem of comprehending intricate AI models will be solved by the creation of increasingly advanced explainability strategies. Gaining the confidence of regulatory agencies as well as the general public will depend on this.
- Cross-industry cooperation: To exchange best practices and tackle shared issues related to AI in loan underwriting, financial institutions, tech firms, and regulatory agencies will work together. This cooperative strategy will promote a more reliable and moral use of AI technology.
AI loan underwriting’s future will be defined by ongoing innovation, more transparency, and a delicate balancing act between automation and human judgment. Artificial Intelligence (AI) will play a major role in creating a lending environment that is more responsible, efficient, and tailored as regulations change and technology progresses.