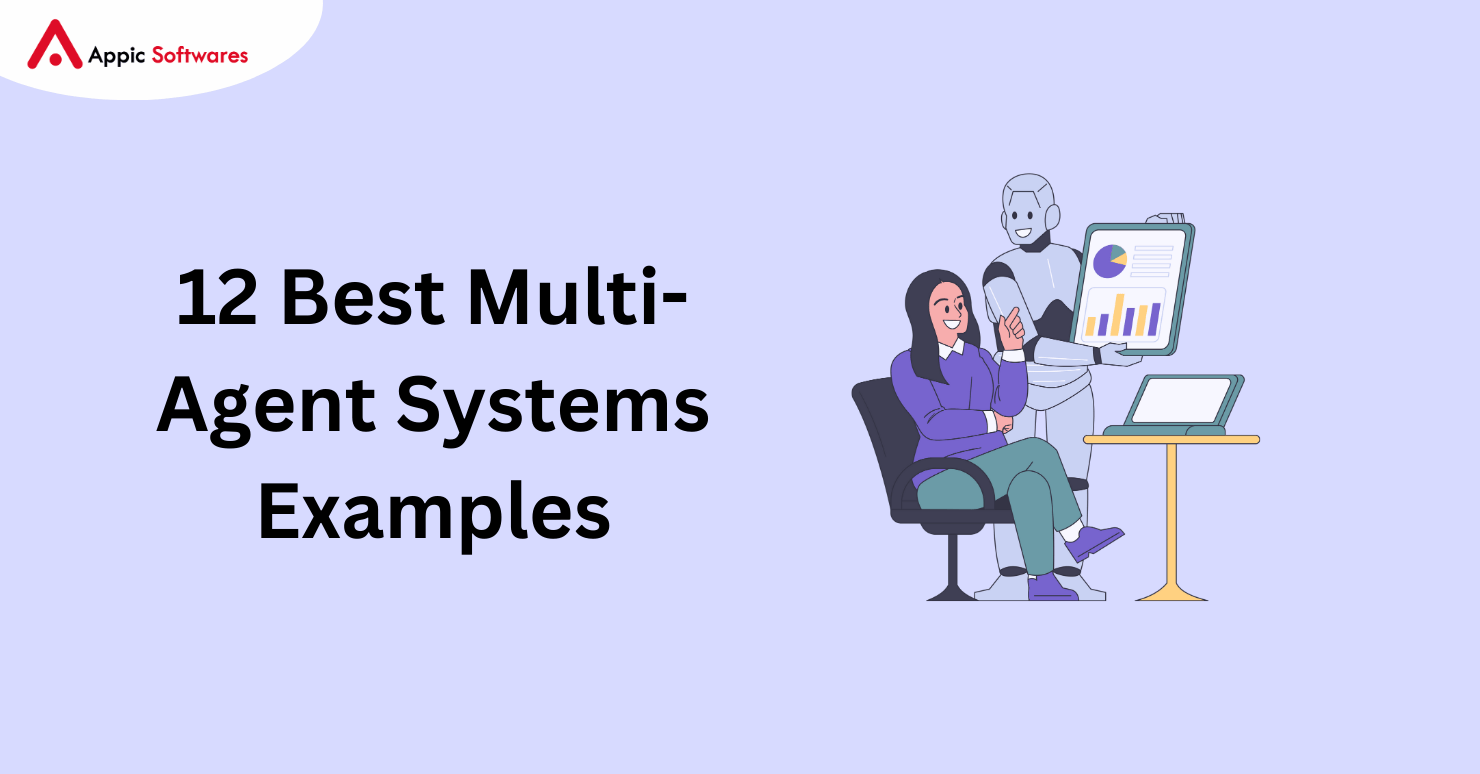
Do you know 54% of the users don’t mind interacting with AI until their problem is solved, whereas 39% of the users are comfortable with AI Agents scheduling appointments with them?
Multi-agent systems have grown as people look for smart ways to let many simple programs work together. In simple terms, these systems let different agents share work and make choices on their own. Many businesses and schools now use multi-agent systems to solve tasks quickly and with fewer errors. This article looks at multi-agent systems, gives 12 solid examples, and explains how to choose the type of multi-agent system that fits your needs. In this post, you will see many Multi Agent Systems Examples that show real use in daily work and smart gadgets.
What Are Multi-Agent Systems?
Source: https://www.ai-jason.com/
So, what exactly are Multi-Agent Systems? At their core, they’re teams of agents that join forces to tackle problems or finish jobs. These agents can be simple software that follows basic rules or a smart Multi-Agent AI System setup that learns and changes as they go. The big idea here is that these agents don’t need one leader telling them every move. Instead, they make choices on their own and work as a group to get results.
Think of it like a group of friends planning a picnic. One friend brings food, another picks the spot, and someone else handles games. They all talk and adjust plans so the picnic goes smoothly. That’s how a Multi-Agent System operates. Each agent has a role, and they share info to make everything work. This setup is called decentralized, meaning no single boss runs the show.
Communication is a huge part of this. Agents chat with each other, either by sending messages or watching what others do. For example, imagine toy robots cleaning a room. One robot finishes dusting the shelves and lets another know it’s time to vacuum the floor. This back-and-forth keeps them on track. Plus, each agent can act on its own, which we call autonomy. If one robot runs out of battery, the others keep going and figure out how to finish without it.
Multi-agent systems pop up in tons of places, like robotics, traffic control, and even video games. They shine when a job is too big or tricky for one agent alone. Take city traffic as an example, hundreds of cars need to move smoothly, and a Multi-Agent System in AI can help them talk and avoid chaos. Or picture a bunch of sensors tracking weather changes across a forest. They share data to give us a full picture.
12 Examples Of Multi-Agent Systems
Below are 12 clear examples that show how multi-agent systems work in real life. Each example stands on its own and proves the value of a team of agents.
-
Swarm Robots
Swarm robots use many small robots that work together to complete a task. These agents work as a group to cover large spaces or to pick up items. Many projects use these robots in cleaning tasks or in farming work. In one case, a group of robots cleans a field in record time. This example is a solid Multi-Agent Systems Examples case that shows how each simple part adds strength. Some projects even call this setup a Multi-Agent AI System to explain its smart work.
Use Cases of Swarm Robots
- Search and Rescue Operations
Swarm robots are highly effective in disaster response situations, such as earthquakes, floods, or collapsed buildings. These small, agile robots can navigate through debris, locate trapped survivors using thermal imaging and sensors, and relay real-time data to human rescue teams. Their ability to spread out and cover large areas quickly makes them invaluable in emergencies where time is critical. - Agriculture and Precision Farming
Swarm robots are transforming modern farming by enabling automated, high-precision agricultural tasks. They can monitor soil health, detect plant diseases, and optimize irrigation systems. Autonomous robots working in a swarm can also plant seeds, remove weeds, and apply fertilizers or pesticides selectively, reducing waste and improving crop yield. - Military and Defense
In defense applications, swarm drones are used for surveillance, reconnaissance, and combat support. Unlike traditional military drones, which rely on human operators, swarm drones work collaboratively to identify threats, perform area mapping, and execute coordinated attacks on targets. Their decentralized nature makes them resilient to communication failures or enemy countermeasures. - Space Exploration
Space agencies like NASA and ESA are exploring the potential of swarm robotics for planetary exploration. A group of autonomous robots can be deployed on the Moon, Mars, or asteroids to collect samples, map terrain, and conduct scientific experiments. Since these robots can work independently and repair their formations if some fail, they offer a robust solution for deep-space missions where human intervention is limited. - Medical Applications (Nanobots in Healthcare)
Swarm robotics extends to microscopic levels, where nanobots are being developed for medical applications. These tiny robots can travel through the human bloodstream to deliver drugs directly to affected areas, perform microsurgery, or remove harmful substances like cancer cells. This targeted approach increases treatment effectiveness while minimizing side effects. - Autonomous Transportation and Traffic Management
Swarm intelligence principles are being applied to self-driving cars and smart transportation systems. Autonomous vehicles can communicate with each other in real time to optimize traffic flow, prevent congestion, and reduce accidents. By coordinating their movements, these vehicles can react dynamically to road conditions and improve overall urban mobility. -
Self-Driving Cars
Self-driving cars can share information to keep roads safe and smooth. Each car acts as an agent that sends data about its speed and position. When cars work together, they help lower traffic and reduce accidents. This use shows strong teamwork. Many experts mention a Multi-Agent system in AI when they talk about self-driving cars. The many cars join forces to make travel safe and smooth.
Use Cases of Self-Driving Cars
- Autonomous Ride-Sharing and Transportation Services
Companies like Tesla, Waymo, and Uber are developing fleets of self-driving taxis that can operate 24/7 without a driver. These cars use multi-agent communication to coordinate pickups, reduce idle time, and optimize routes based on traffic conditions, providing cost-effective and efficient mobility solutions. - Smart Traffic Management and Congestion Control
Self-driving cars contribute to intelligent traffic systems by sharing data on speed, congestion, and road conditions with nearby vehicles and city infrastructure. By coordinating movements and adjusting speeds dynamically, they help reduce traffic jams, minimize stop-and-go driving, and optimize fuel efficiency. - Logistics and Autonomous Delivery Vehicles
Companies like Amazon and FedEx are integrating self-driving trucks and delivery robots into their supply chains. These vehicles can transport goods autonomously across long distances, reducing delivery times and operational costs. Urban delivery bots also use multi-agent coordination to navigate crowded streets and deliver packages efficiently. - Emergency Response and Safety Enhancements
Self-driving cars can improve emergency response by clearing paths for ambulances, fire trucks, and police vehicles through vehicle-to-vehicle (V2V) communication. Additionally, their AI-driven accident prevention systems can detect hazards, predict collisions, and take corrective actions faster than human drivers. - Autonomous Public Transport Systems
Many cities are experimenting with self-driving buses and shuttles for public transportation. These vehicles operate in designated lanes and leverage multi-agent coordination to ensure smooth passenger flow, reduce transit delays, and enhance accessibility in urban areas. - Platooning in Freight Transport
Self-driving trucks can form platoons, where multiple trucks drive closely together in a synchronized manner to reduce air resistance and save fuel. This technology enhances fuel efficiency, reduces emissions, and improves road safety by minimizing human error in long-haul transportation. - Elderly and Disabled Mobility Solutions
Self-driving cars offer greater independence to elderly and disabled individuals who may not be able to drive. Autonomous vehicles equipped with accessibility features can provide seamless transportation without relying on human drivers.
-
Smart Home Systems
Smart home systems let many devices work together to run a house. Lights, locks, and heaters all talk with each other. When one device senses a change, it informs others to act. Homeowners see faster responses and less waste of energy. This example fits well with Multi-Agent Systems Examples. In many smart houses, a Multi-Agent AI System is used to manage daily tasks simply.
Use Cases of Smart Home Systems
- Energy Management and Optimization
Smart home systems use AI-driven thermostats like Nest or Ecobee to regulate heating and cooling based on user behavior and external weather conditions. By coordinating with smart lighting, appliances, and solar panels, the system optimizes energy consumption, reducing electricity bills and environmental impact. - Home Security and Surveillance
Smart security systems integrate cameras, motion sensors, and smart locks to provide real-time monitoring and alerts. Multi-agent coordination allows security devices to work together—if a motion sensor detects movement, security cameras activate, and smart lights turn on to deter intruders. Additionally, AI-powered doorbells like Ring can recognize familiar faces and notify homeowners of visitors. - Automated Home Assistants
Voice assistants like Amazon Alexa, Google Assistant, and Apple Siri act as central hubs that control multiple smart devices. They coordinate tasks like playing music, setting reminders, adjusting lighting, or even managing home appliances through voice commands. These assistants continuously learn from user interactions to improve responses and automate routines. - Smart Appliances for Convenience
Smart refrigerators, ovens, and washing machines can communicate with each other to streamline household tasks. For example, a smart refrigerator can track food inventory and suggest recipes based on available ingredients, while a smart oven preheats automatically when a meal is selected. These appliances improve efficiency and reduce manual effort. - Health and Well-being Monitoring
Smart home systems integrate with wearable devices and health monitors to track vital signs, detect falls, and send alerts to caregivers or emergency services if necessary. For elderly individuals, multi-agent systems can ensure medication reminders, monitor movement patterns, and provide assistance when needed. - Home Entertainment and Customization
Multi-agent coordination allows smart TVs, speakers, and lighting systems to create immersive experiences. For example, a home theater system can adjust lighting, close curtains, and set the perfect sound level when a movie starts, creating a seamless entertainment experience. - Automated Household Chores
Smart vacuum cleaners like Roomba and robotic mops can map home layouts, detect obstacles, and work in coordination with other smart home devices. For instance, when the vacuum starts cleaning, the smart air purifier can adjust settings to remove dust particles, enhancing indoor air quality.
-
Traffic Management Systems
Traffic lights and road sensors work as a team in traffic management systems. Each device sends data about car flows and road conditions. Together, they adjust light times and reduce jams. In busy cities, this team work helps reduce delays and makes driving safer. Experts often mention a Multi-Agent system in AI when they talk about such systems. These examples show how simple agents work together to improve road safety.
Use Cases of Traffic Management Systems
- Intelligent Traffic Signal Control
Multi-agent systems optimize traffic lights by adjusting signal timings based on real-time traffic conditions. AI-driven sensors detect vehicle density and pedestrian movements at intersections, allowing signals to switch dynamically to minimize congestion. During emergencies, the system prioritizes ambulances, fire trucks, and police vehicles by altering signals to clear a direct path. Smart intersections also improve pedestrian safety by detecting movement and modifying crosswalk signals accordingly.
- Real-Time Traffic Monitoring and Congestion Management
Traffic congestion is a major challenge in urban areas, and multi-agent systems help address this by monitoring roads in real time. AI-powered surveillance cameras and sensors track vehicle movement, detect slowdowns, and provide live traffic updates. Navigation apps such as Google Maps and Waze use this data to recommend alternative routes to drivers, reducing overall congestion. In some cities, adaptive lane management allows authorities to change lane directions dynamically during peak hours, ensuring smoother traffic flow.
- Autonomous Vehicle Coordination
Self-driving cars rely on multi-agent systems to communicate with traffic infrastructure and other vehicles to navigate safely. Autonomous vehicle coordination ensures that cars maintain optimal speed, safe following distances, and efficient lane changes. The concept of vehicle platooning, where groups of autonomous trucks travel closely together, reduces air resistance, lowers fuel consumption, and improves highway efficiency. AI-based predictive modeling further enhances this coordination by forecasting congestion patterns and preemptively adjusting vehicle movements.
- Public Transportation Optimization
Multi-agent systems significantly improve the efficiency of public transportation. AI-powered transit systems dynamically adjust bus and metro schedules based on real-time passenger demand. Smart bus stops display live updates on arrival times, reducing commuter wait times. Ride-sharing services like Uber and Lyft also integrate with traffic systems to optimize driver dispatching, ensuring faster pick-ups and reduced idle time. By streamlining public transport operations, these systems enhance accessibility and convenience for commuters.
- Accident Detection and Emergency Response
Traffic management systems use AI-driven surveillance to detect accidents and respond instantly. When a collision or hazard occurs, the system automatically alerts emergency services, reducing response times. Smart road signs update dynamically to warn approaching drivers about road closures, weather hazards, or construction work. Vehicle-to-infrastructure (V2I) communication enables automated crash reporting, helping authorities take immediate action to prevent secondary accidents and ensure road safety.
- Smart Parking Solutions
Finding parking spaces in congested urban areas is a major contributor to unnecessary traffic. Multi-agent systems address this issue by using IoT-based sensors to detect available parking spots and guide drivers through mobile applications. AI-driven parking management systems optimize parking allocation, reducing vehicle movement within parking lots and enhancing overall space utilization. Some advanced systems even enable autonomous valet parking, where self-driving cars locate and park themselves in designated spots without human intervention.
- Environmental Impact Reduction
Traffic congestion leads to increased fuel consumption and emissions, but multi-agent traffic management systems help mitigate this by optimizing traffic flow. AI-driven signal control and route planning reduce vehicle idling, lowering carbon emissions. Green corridors prioritize electric and public transport vehicles, promoting sustainable mobility. Automated toll collection systems eliminate the need for manual stops, allowing smooth traffic movement and further reducing fuel wastage.
-
Financial Trading Bots
Financial trading bots work in groups to help banks and investors. These agents work in real time to watch markets and make trades. They share facts quickly to decide on the best move. This teamwork saves time and cuts errors in fast markets. Many projects use these bots as Multi-Agent System Examples. They help make smart choices in buying and selling stocks.
Use Cases of Financial Trading Bots
- High-Frequency Trading (HFT)
High-frequency trading bots use multi-agent systems to execute thousands of trades per second based on microsecond-level market fluctuations. These bots analyze price movements, liquidity levels, and market depth to identify arbitrage opportunities across different exchanges. By automating trade execution with minimal latency, they capitalize on price discrepancies that are imperceptible to human traders. Investment firms and hedge funds rely on these systems to gain a competitive edge, ensuring higher profitability with reduced manual effort.
- Portfolio Management and Risk Mitigation
Trading bots also function as intelligent portfolio managers, automatically balancing asset allocations based on risk tolerance and market conditions. They continuously monitor stock indices, cryptocurrencies, and commodities, adjusting investment portfolios in real time to minimize risks. Advanced bots use AI-powered predictive analytics to forecast market downturns and reallocate assets to safer investments. This capability is especially beneficial for institutional investors, who require stable and optimized financial strategies over extended periods.
- Market-Making and Liquidity Provision
Market-making bots play a crucial role in maintaining liquidity in financial markets by continuously placing buy and sell orders. These bots ensure that assets remain tradable by reducing bid-ask spreads and increasing transaction volumes. Crypto exchanges and stock markets use market-making bots to stabilize price fluctuations and prevent extreme volatility. By acting as intermediaries, these bots enhance market efficiency, ensuring seamless transactions for retail and institutional investors alike.
-
Virtual Personal Assistants Network
Virtual personal assistants, such as those found in phones and computers, can work together. They share work to answer questions, set reminders, and manage calendars. When one assistant asks another for data, the response is quick and clear. This teamwork shows a strong use of multi-agent systems. Many developers call this setup a Multi-Agent AI System when they program smart responses.
Use Cases of Virtual Personal Assistants Network
- Smart Office and Workflow Automation
In a corporate environment, a VPA network can coordinate between different departments and tools to automate workflow processes. For example, an AI assistant integrated with project management software can schedule meetings, assign tasks, and provide progress updates based on real-time data. It can also communicate with customer relationship management (CRM) systems to send follow-up emails, manage client interactions, and analyze business trends. By reducing manual workload and improving efficiency, virtual assistants enhance workplace productivity.
- Personalized Health and Wellness Management
A network of virtual personal assistants can revolutionize health management by integrating with wearable devices, medical records, and telemedicine platforms. For instance, one assistant might track a user’s daily physical activity, while another analyzes dietary habits and sleep patterns. If a user’s health metrics indicate potential risks, the system can proactively schedule doctor appointments or suggest lifestyle adjustments. These assistants also provide medication reminders and mental health support, ensuring comprehensive well-being management.
- Smart Home and IoT Device Coordination
In a smart home ecosystem, a network of virtual assistants can control IoT-enabled devices to create a more efficient living environment. For example, one assistant manages lighting and temperature based on occupancy patterns, while another oversees security by monitoring smart cameras and door locks. These systems can also integrate with grocery management apps to track food inventory, suggest meal plans, and automatically order essentials. By working together, virtual assistants create a highly adaptive and user-centric home experience.
-
Distributed Sensor Networks
Distributed sensor networks use many sensors spread over an area. Each sensor gathers local data like temperature or motion. The sensors share facts with a central system that makes a decision. This system can act fast when needed, such as in security alarms. In some cases, a Multi-Agent system LLM helps to process the sensor data and give a clear picture of events.
-
Warehouse Management Robots
Warehouse management robots help large stores sort and pack items. Many small robots work together to carry goods, check stock, and send updates. Each robot handles a small part of the task. When they work as a team, orders are filled quickly.
-
Disaster Response Robots
In a disaster, small robots work together to search for people and clear paths. Each robot takes a small area and sends data to a central point. Their teamwork helps save time and gives clear information to helpers. Many emergency teams use this setup to work faster and safer in dangerous sites.
-
Drone Fleet Systems
A fleet of drones can work together to take aerial photos or monitor large sites. Each drone flies on its own and sends live video to a base. When the drones work as a team, they cover a wide area quickly. Many experts call these setups Multi-Agent Systems Examples. Some teams also refer to them as a Multi AI Agent System when the drones adjust their paths based on live facts.
-
Social Media Bots
Social media bots work together to manage posts and replies on large platforms. Each bot handles simple tasks like posting updates or checking for errors. When they work in a group, the system manages large networks smoothly. Many platforms use these systems as Multi-Agent Systems Examples. The teamwork helps keep online spaces active and clear of mistakes.
-
Medical Diagnosis Systems
Medical diagnosis systems use many small programs to check patient data. Each agent reviews a part of a patient’s record. When the agents share their findings, doctors get clear advice on treatments. This teamwork is a strong example of multi-agent systems work. Many researchers also call this setup a Multi-Agent system LLM because the agents help sort and process health facts.
How To Choose The Type Of Multi-Agent System?
Picking the right Multi-Agent System isn’t hard if you know what to look for. Different jobs need different setups, so let’s break it down into simple steps. Here’s how to choose the best type for what you need.
-
Know Your Goal
Start by figuring out what you want the system to do. Are you managing traffic, running a farm, or building a game? The goal decides what the agents need to handle. For example, traffic needs agents that talk fast, while farming might need ones that check the soil. Clear goals lead to the right choice.
-
Count the Agents
How many agents do you need? Small jobs might work with just a few, like a smart home with five devices. Big tasks, like running a power grid, could need hundreds. More agents mean more power but also more planning, so match the number to the job.
-
Decide How Free Agents Should Be
Some agents follow strict steps, while others make their calls. This freedom, or autonomy, depends on the task. In a factory, agents might stick to a script. In a rescue mission, they need to think on the fly. Pick what fits your situation—tight rules or loose reins.
-
Plan the Talking
Agents need to share info, but how? Direct messages work for small groups, like robots chatting in a warehouse. Bigger systems might watch each other’s moves, like cars on a highway. Good communication keeps them in sync, so choose a way that’s simple and effective.
-
Check the Surroundings
Where will the system work? A quiet lab is easy, but a busy city or wild forest is trickier. Agents need to handle whatever comes—rain, noise, or crowds. A Python Multi-Agent System could be coded to adapt, making it a solid pick for changing spots.
-
Think About Growth
Will your system need to get bigger later? Some setups add agents easily, like sensors spreading across a park. Others don’t scale well, so plan. If growth matters, pick a system that won’t stumble when you expand it.
-
Watch the Cost
Building and running a Multi-Agent System takes money and effort. Simple ones cost less but do less. Plus, a Multi-Agent system LLM with learning power, might cost more but solve harder problems. Balance what you can spend with what you need done.
Final Words
Now you’ve learned how to pick the right type, which helps you use multi-agents smartly. As 2025 rolls closer, these systems will keep growing, bringing more surprises and solutions. So next time you see a self-driving car or a smart gadget, remember: it might just be part of a Multi-Agent System, working hard behind the scenes!
Want to chat about multi-agent systems or have a project in mind? We’re just a message away—let’s team up like agents do! Get in touch with us.
FAQs
How do swarm robots function as a multi-agent system?
Swarm robots work collectively to perform tasks such as search and rescue, environmental monitoring, and warehouse automation. They use decentralized decision-making, where each robot follows simple rules and communicates with others to achieve a larger objective without central control.
How do self-driving cars utilize multi-agent systems?
Self-driving cars communicate with other vehicles, traffic signals, and road infrastructure to navigate safely. They use AI-driven agents to analyze real-time traffic data, avoid collisions, optimize routes, and adapt to changing road conditions.
How do smart home systems represent multi-agent systems?
Smart home systems involve multiple intelligent devices, such as voice assistants, security cameras, thermostats, and lighting controls, that communicate with each other. They create an automated living environment by learning user preferences and optimizing energy consumption, security, and comfort.
What role do financial trading bots play in multi-agent systems?
Financial trading bots operate as autonomous agents that analyze market trends, execute trades, and manage portfolios. They use real-time data processing and AI-driven decision-making to optimize investment strategies, minimize risks, and maximize returns in highly dynamic financial markets.
How do multi-agent traffic management systems work?
Traffic management systems use AI-powered agents, such as smart traffic signals, vehicle sensors, and navigation systems, to optimize road usage. These agents monitor traffic flow, adjust signal timings, provide congestion updates, and facilitate emergency response coordination.
How do virtual personal assistants form a multi-agent system?
Virtual personal assistants (VPAs) such as Siri, Google Assistant, and Alexa interact with multiple AI-driven agents to manage schedules, automate tasks, and provide personalized recommendations. They integrate with smart home devices, work calendars, and communication tools to enhance productivity and convenience.
What industries benefit the most from multi-agent systems?
Industries that benefit from multi-agent systems include transportation, healthcare, finance, logistics, retail, and smart infrastructure. These systems improve automation, efficiency, and decision-making in complex, real-time environments.
What are the advantages of using multi-agent systems?
Multi-agent systems offer scalability, flexibility, and robustness in handling distributed tasks. They improve decision-making, enable real-time problem-solving, and enhance efficiency in environments where multiple intelligent entities must work together dynamically.