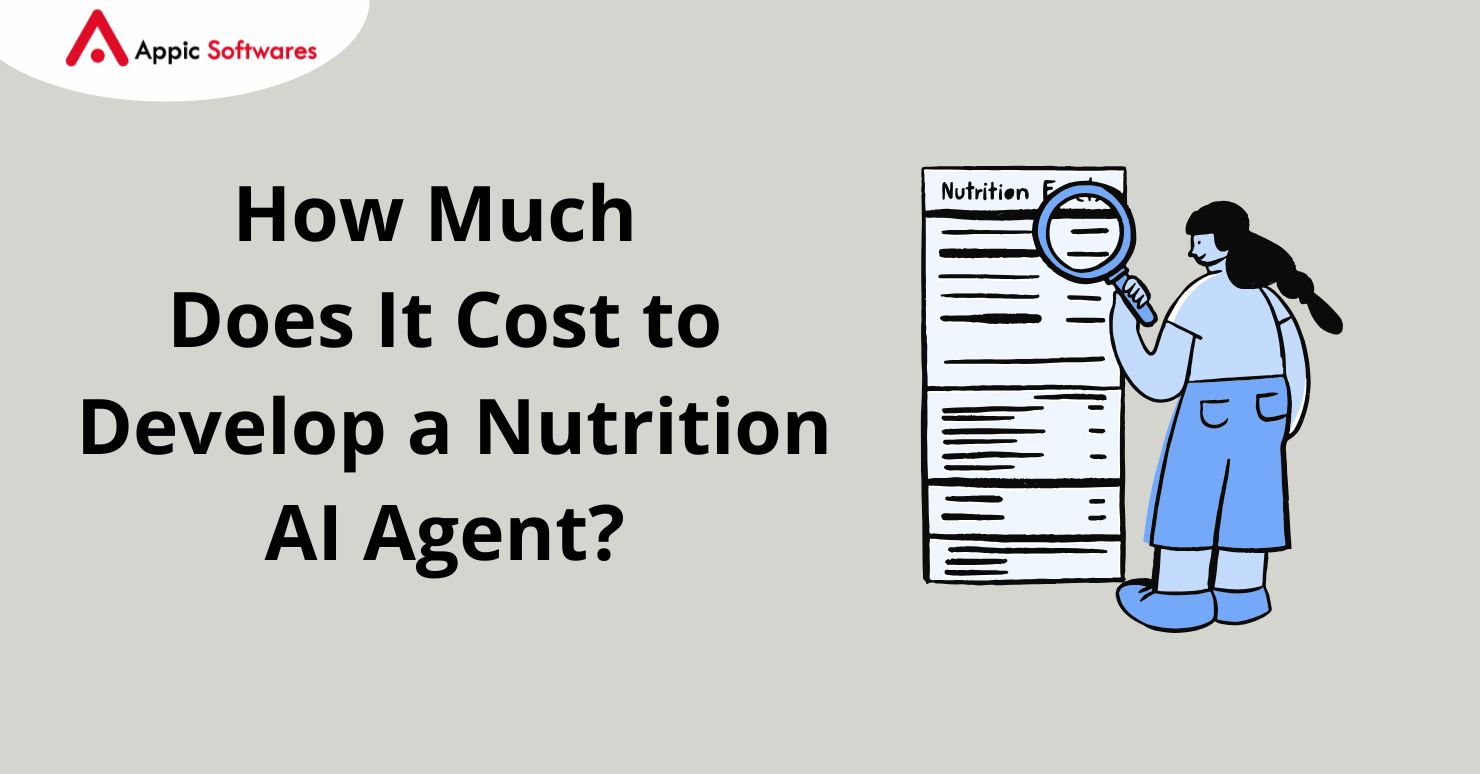
The application of artificial intelligence (AI) in health and nutrition is one of the most trending topics today. The global AI market for personalized nutrition grew from $2.96 billion in 2023 to $3.66 billion in 2024, with a CAGR of over 20%. In 2022, the AI in the personalized nutrition market size was $1.6 billion. Today, thanks to tools that are based on artificial intelligence (AI), people can get individualized diet recommendations, monitor their food consumption, and have instant access to their nutritional requirements. Yet, the businesses interested in creating a Nutrition AI Agent wonder one thing: What is the cost of developing a Nutrition AI Agent? Keep reading to learn about that and more about nutrition AI agents, like uses of AI agents and different types of AI agents.
What Are AI Agents?
The first thing one needs to know before going into the costs is what AI agents are and their role in nutrition.
AI agents are autonomous or semi-autonomous systems that can accomplish tasks by processing data, making decisions, and learning from interactions with the user. These agents use machine learning (ML), NLP, deep learning, and data analytics to increase the effectiveness of their functionality.
Types Of AI Agents
Intelligent systems called AI agents can sense their surroundings and know what is happening in them, analyze the data gathered, and carry out activities meant to reach particular goals. They are employed in applications including automation, decision-making, and predictive analysis as well as artificial intelligence, where they are rather important. Based on their functionality, learning capacity, and degree of autonomy, artificial intelligence agents can be classified. The following are the key kinds of artificial intelligence agents and their functions in the bigger artificial intelligence ecosystem:
1. Reactive AI agents
The most basic kind of artificial intelligence is reactive. Working in real time, these agents have no memory and cannot learn from past experiences; they are activated by specific inputs.
They are based on the rules and logic that have been predefined for them, and thus they are very efficient for the situations that require a direct response in a structured environment.
Illustrations Of Reactive AI Agents
- IBM’s Deep Blue, which defeated world chess champion Garry Kasparov, employed a rule-based approach to examine all possible moves but did not learn from prior games.
- Email spam filters utilize pre-defined criteria to find and remove undesirable emails based on keywords, sender reputation, and formatting patterns.
- The most fundamental facial recognition software does not improve its accuracy with time; it can match faces to photographs in a database.
- The most fundamental facial recognition software does not improve its accuracy with time; it can compare faces to images in a database.
2. Model-Based (Deliberative) Artificial Intelligence Agents
Model-based AI agents can build a mental model of their environment, and depending on this model, they can consider all the potential answers before selecting one of them. To solve problems in complicated systems, these agents employ search algorithms, prediction, and reasoning.
Examples of AI agents
- Self-driving automobiles can negotiate their way to destinations through roads, people, and traffic laws and norms.
- Many contemporary games use model-based artificial intelligence to create smart non-player characters (NPCs) who react dynamically to the player’s activities.
- Industries run RPA systems using rule-based decision-making to automate mundane activities.
- Industries apply rule-based decision-making in RPA systems to automate repetitive tasks, hence creating robotic process automation (RPA).
3. Cognitive artificial intelligence agents
Cognitive AI agents are those bots able to imitate human thought processes. By means of sophisticated machine learning techniques, these agents examine past data, spot trends, and enhance decision-making.
Cognitive AI systems are different from reactive agents in that they gain knowledge and improve their performance with time.
Cognitive AI Agent Examples
- AI chatbots (ChatGPT, Google Bard): These conversational artificial intelligence agents give increasingly appropriate and natural replies over time by learning from past interactions.
- Recommendation systems: Netflix, Amazon, and Spotify. These tools examine user activity to recommend movies, merchandise, or music customized to individual tastes.
- Fraud detection with artificial intelligence: Banks and financial institutions utilize cognitive artificial intelligence to identify fraudulent transactions depending on past data and changing fraud tendencies.
- Banks and financial institutions: They use cognitive artificial intelligence to identify fraudulent transactions depending on historical data and changing fraud patterns.
4. Autonomous agents In AI
Operating autonomously, autonomous artificial intelligence agents make choices and carry out tasks outside of direct human supervision. Adapting to changing surroundings, they always hone their algorithms and get better with time. These agents are often sent into high-risk or high-efficiency settings like robotics and financial markets.
Examples Of Autonomous Artificial Intelligence Agents
- Using AI-powered decision-making, self-driving cars (Tesla Autopilot, Waymo) negotiate traffic, identify obstructions, and change routes in real time.
- AI-powered drones run surveillance, deliver packages, and monitor agricultural fields under autonomous control.
- AI-driven stock trading bots examine financial markets, carry out trades, and maximize portfolios free of human supervision.
- AI-driven stock trading bots monitor financial markets, carry out trades, and maximize portfolios without human intervention.
5. AI Agents Based On Goals
Goal-based artificial intelligence agents are meant to reach a certain goal by analyzing several possible actions and choosing the most efficient path. To find the optimal approach to fulfill a pre-defined goal, these agents use search algorithms, decision trees, and optimization methods.
Examples Of Goal-Based Artificial Intelligence Agents
- These AI-driven robots optimize cleaning routes and maneuver around barriers to efficiently cover an entire area.
- Strategy-based games use goal-driven artificial intelligence to challenge players with smart opponents that dynamically change tactics.
- By means of delivery route and inventory control optimization, artificial intelligence technologies enable businesses to cut costs and increase efficiency.
- AI technologies are helping businesses cut costs and increase efficiency using delivery routes and inventory control.
6. AI Agents Based On Utility
By considering several elements, utility-based artificial intelligence agents surpass basic goal attainment to find the most desirable result. These agents pick the one with the greatest projected benefit from among many actions given numerical values.
Examples Of Utility-Based Artificial Intelligence Agents
- AI-powered medical diagnosis systems evaluate patient history, test data, and symptoms to recommend the best feasible treatment plans.
- AI-driven investment platforms examine risk tolerance, market conditions, and financial goals to offer the best investment ideas.
- Weather prediction models: High-accuracy weather pattern forecasts are produced by these artificial intelligence systems, examining several variables like temperature, humidity, and wind speed.
- These artificial intelligence systems forecast weather patterns with great accuracy by analyzing several variables, including temperature, humidity, and wind speed.
7. Learning AI Agents
Learning AI agents increase their effectiveness by constantly learning from experiences, adjusting to changes, and honing their knowledge base. To improve decision-making, these agents use supervised learning, unsupervised learning, and reinforcement learning.
Learning AI Agent Examples
- Developed by DeepMind, the AlphaGo system became a Go master using game analysis and skill development via reinforcement learning.
- AI systems in cybersecurity systems detect emerging threats through their ability to learn from past attacks.
- Autonomous robots in manufacturing use AI to optimize production processes by learning operational data to enhance efficiency.
Benefits Of AI Agents In Nutrition
AI Agents are transforming nutrition delivery systems for individuals and businesses alike. Machine learning together with data analytics and real-time monitoring, enables AI-powered nutrition solutions to deliver individualized guidance and improved food selection and lifestyle support. The implementation of AI technology in nutrition delivers various advantageous outcomes, which can be summarized as follows:
Personalized Diet Plans
AI agents gather different types of personal health data, which include age, weight, body composition, dietary restrictions, allergies, fitness targets, and medical conditions, to develop customized eating plans. AI-driven nutrition plans provide continuous dietary recommendations that match a person’s current needs because they adjust their suggestions using real-time feedback that exceeds generic diet programs.
Based on micronutrient deficits, an AI nutrition assistant creates tailored high-protein diet plans for sportsmen as well as low-carb eating plans for diabetics and balanced vegetarian diets. The AI-generated meal plans provide dietary suggestions using both personal taste preferences and scientific nutritional understanding.
Nutritional Insights In Real Time
AI-driven nutrition applications offering instant feedback on users’ food choices enable them to simply follow a balanced diet. These apps analyze meals using image recognition, barcode scanning, and natural language processing; they then compute their nutritional values, projected calorie count, macronutrient breakdowns (carbs, protein, fats), and micronutrient breakdowns (vitamins, minerals) in real time.
A user may take a picture of their meal to let an artificial intelligence program exactly identify nutritional content. While their eating habits improve from real-time data analysis, users can make better food choices during their everyday activities. By connecting food intake to exercise activities, AI-based nutritional analysis interacts with wearable fitness trackers to create health links.
Grocery Help That Is Automated
When confronted with many processed foods and high-calorie goods in stores, choosing healthy food products becomes difficult. The AI-powered grocery assistant generates tailored shopping lists to fit your dietary goals.
AI systems connect their food selection with health targets by examining user dietary needs and wellness goals. AI-based meal planners provide weekly grocery lists with food components supporting heart health or muscle gain, or weight loss. On websites recommending whole grain bread instead of white bread and plant-based protein sources for vegetarians, users can discover healthier substitutes.
AI agents combined with online shopping systems and delivery services let consumers plan their food needs and get fresh, healthy products at home.
Risk Prevention And Health Monitoring
By identifying the early warning indicators of nutritional problems and lifestyle diseases, artificial intelligence agents are absolutely vital in preventive healthcare. Before diseases like obesity, diabetes, high blood pressure, and cardiovascular disease occur, AI systems identify health concerns by analyzing eating patterns in conjunction with biometric data and medical history.
Artificial intelligence technology-based health monitoring apps detect salt intake to warn users of hypertension risks. Examining blood sugar trends helps to suggest modifications to carbohydrate consumption for those with diabetes risk factors. These artificial intelligence technologies help people create preventative lifestyle decisions for lifetime health preservation during their lives.
Artificial intelligence combined with genetic testing services provides consumers with in-depth knowledge about how genetic characteristics affect metabolic rates, dietary sensitivity, and nutrient absorption capacity. Using individualized biological profiles, the tailored preventive healthcare strategy generates exact food suggestions for every individual.
Use Cases Of Nutrition AI Agent
AI agents are revolutionizing the nutrition industry by enabling personalized, efficient, and real-time health and dietary management. Here are some of the most impactful applications:
1. Nutritional Apps Driven By AI
AI-powered nutritional apps such as MyFitnessPal and Lifesum use machine learning algorithms and data analytics to offer users a highly customized health experience. These apps analyze users’ dietary habits, health goals, and activity levels to provide:
- Personalized Meal Suggestions: Based on preferences, dietary restrictions (like vegan, keto, or gluten-free), and health goals.
- Smart Diet Assessment: AI evaluates the quality of a user’s diet and provides insights on nutrient intake, deficiencies, or imbalances.
- Intelligent Food Tracking: By learning from a user’s past entries and preferences, AI makes logging food quicker and more accurate over time.
These applications help users stay consistent and make informed decisions about their diet and lifestyle.
2. Virtual AI Nutritionists
Platforms like Noom offer AI-driven health coaching by combining behavioral psychology with nutritional science. These AI agents function as virtual dieticians, providing support that goes beyond basic recommendations:
- Understanding Behavioral Patterns: The AI monitors user interactions, mood patterns, and decision-making tendencies to personalize motivational strategies.
- Customized Advice: Users receive timely tips, encouragement, and alerts tailored to their emotional state and lifestyle habits.
- Interactive Support: AI chatbots simulate real conversations, making it easier for users to ask questions about food choices, portion sizes, or emotional eating.
This creates a more holistic approach to nutrition and wellness by addressing both physical and psychological factors.
3. AI-Based Food Recognition
AI models utilizing deep learning and computer vision allow for the identification and classification of food items through photos. This functionality is particularly useful for seamless food tracking:
- Image-Based Food Logging: Users can snap a photo of their meal, and the AI identifies the food, estimates portion sizes, and calculates nutritional values.
- Automated Nutrient Analysis: The AI breaks down each component of the meal to assess calorie count, macro and micronutrients.
- Enhanced Accuracy Over Time: With each user input, the system learns and improves its recognition capabilities, making it more precise and user-friendly.
This makes food tracking effortless and removes the need for manual entry.
4. AI-Powered Wearables
Wearables like Fitbit, Apple Watch, and other health trackers have integrated AI nutrition agents to offer real-time insights and personalized recommendations:
- Real-Time Dietary Guidance: Based on heart rate, activity levels, and caloric burn, the AI suggests meals or snacks to balance energy needs.
- Health Monitoring & Alerts: Users receive alerts if they’re not meeting hydration goals, consuming too much sugar, or not getting enough protein.
- Integrated Wellness Tracking: These wearables sync with nutritional apps to provide a unified dashboard of health metrics including sleep, activity, and diet.
This integration ensures that dietary guidance is responsive to a user’s current physiological state.
Cost To Develop Nutrition AI Agents
Factors Influencing AI Agent Development Cost
Several elements affect the total cost of creating an AI agent:
- AI Complexity: Complex AI models require more expense during development.
- Data Collection & Training: The cost of acquiring and processing vast datasets.
- Technology Stack: Advanced tech stacks require higher development costs.
- Integration with APIs: The integration of health APIs, food databases, and wearable devices incurs additional expenses.
- User Interface & Experience: AI-powered apps that provide a smooth user experience and interface need experienced designers to develop them.
Estimated Development Costs
AI Solution | Estimated Cost Range |
Basic Chatbot | $5,000 – $20,000 |
Advanced AI Meal Planner | $10,000 – $40,000 |
AI Image Recognition for Food | $150,000 – $60,000 |
Full-Scale AI Nutrition Assistant | $10,000 – $80,000 |
AI Agent development for businesses becomes more affordable by establishing a partnership with an AI agent development company.
What Is The Right Tech Stack For Nutrition AI Agent Development?
Development success depends heavily on the selection of appropriate agentic AI frameworks and technical systems. Some recommended technologies include:
Category | Technologies/Tools |
Programming Languages | Python, JavaScript |
ML Frameworks | TensorFlow, PyTorch, Scikit-learn |
NLP Tools | OpenAI GPT, Dialogflow |
Databases | MySQL, MongoDB, Firebase |
Cloud Platforms | AWS, Google Cloud, Azure |
API Integrations | Nutritionix API, Fitbit API, Apple Health API |
Considerations And Solutions Of AI Agents In Nutrition
Creating an AI agent demands complex solutions to overcome development obstacles.
1. Data Privacy And Security
User health data security stands as a fundamental requirement. AI developers need to implement HIPAA compliance together with encryption and secure authentication measures.
2. AI Model Accuracy And Bias
AI models provide accurate and unbiased nutrition recommendations when they receive continuous training with diverse datasets for assurance.
3. User Engagement And Retention
AI nutrition agents enhance user retention through gamification combined with AI-powered reminder systems and personalized insights.
Conclusion
Multiple elements affect the cost to create a Nutrition AI Agent, including AI complexity as well as technology choices. Companies that want to implement AI Agent Development Services must determine their precise needs and funding capabilities. The right development strategy can generate an effective and profitable AI solution that ranges from simple chatbots to comprehensive AI-powered nutrition assistants.
Are you seeking an AI agent development company? Appic Softwares is the best choice for your AI nutrition project; it demands professional developers who can turn your concept into reality.
FAQs
1. What is a Nutrition AI Agent?
A Nutrition AI Agent is a smart digital system designed to offer personalized dietary recommendations based on various personal health factors such as age, weight, medical history, lifestyle, and eating habits. By analyzing this information, the AI agent helps users make informed food choices, manage health conditions, and develop better eating patterns. These agents are often integrated into mobile apps or web platforms, making it easy for users to access real-time nutritional advice and meal planning tools.
2. What tools are used to develop a Nutrition AI Agent?
To develop a Nutrition AI Agent, developers use a combination of artificial intelligence (AI), machine learning (ML), natural language processing (NLP), and computer vision technologies. These tools enable the agent to understand user inputs, interpret dietary needs, and even recognize food items through images. Backend technologies like Python, Node.js, or Firebase power the system’s functionality, while APIs from databases like USDA or Nutritionix provide detailed nutritional data.
3. How much does it cost to build a Nutrition AI Agent?
The cost of building a Nutrition AI Agent typically ranges from $10,000 to $80,000. A basic version with limited features like manual food logging and simple recommendations may cost between $10,000 and $20,000. A mid-range solution with added functionalities such as personalized plans, integration with wearable devices, and automated food recognition can range from $20,000 to $50,000. For a fully-featured, AI-driven agent with real-time analytics, multilingual support, and advanced personalization capabilities, the development cost may go up to $80,000