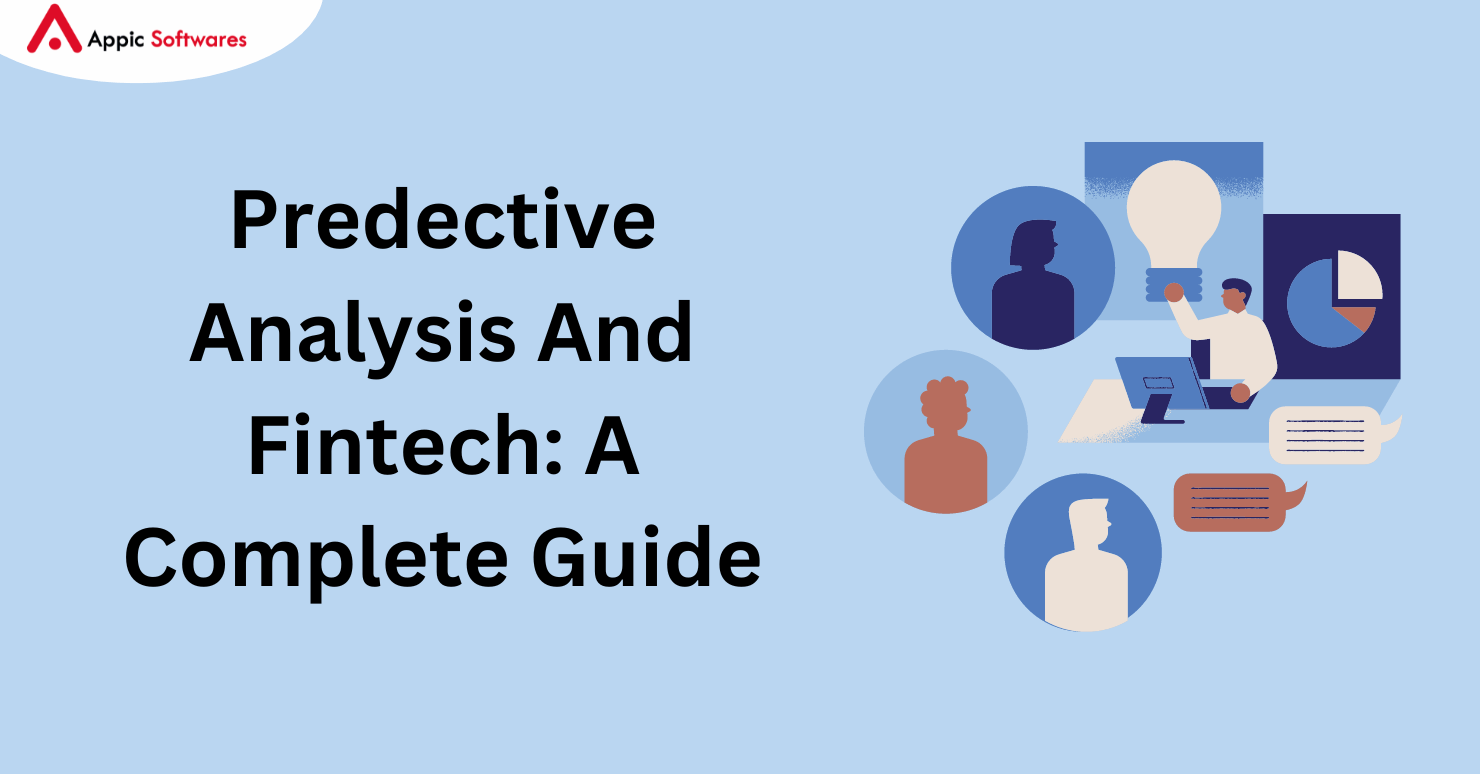
In the constantly changing world of financial technology (FinTech), data is now considered the new currency. It changes the industry and spurs innovation. As digital transactions, internet banking, and mobile payments proliferate quickly, an incredible volume of data is produced every second.
But it’s not just about the amount of data; it’s also about how financial institutions and corporations use it to their advantage to make wise decisions and obtain insightful knowledge.
The amount and variety of information that permeates both the physical environment and the world economy is enormous. It comes from a variety of sources, including credit card transactions, digital apps, web activity, sensors, and satellite imagery.
But unless raw data is joined with other data and transformed into information to improve decision-making, it is of limited value. By placing sales figures, for instance, into the framework of historical data or market patterns, the data acquires significance and offers insightful information. Abruptly, these numbers may show increase or decrease in relation to benchmarks or due to particular tactics.
FinTech organizations must invest in data analytics and the integration of AI-supported predictive analytics as the volume of data created from financial transactions continues to rise. In order to fully utilize these enormous datasets and facilitate sophisticated data analysis, businesses frequently turn to the experience of AI development services. especially those that use machine learning and algorithmic methods to find and extract patterns and inform decision-making.
- Introduction to AI in FinTech
- Advanced Data Analytics for improved decision-making
- How is credit risk management managed by banks?
- Detecting Fraud with AI and ML
- Detecting Fraud with AI and ML
Introduction to AI in FinTech
The Mordor Intelligence analysis estimates that the Fintech AI market would be valued $42.83 billion in 2023. By 2028, it is anticipated to reach $49.43 billion. In addition, it is projected to expand at a compound annual growth rate (CAGR) of 2.91% from 2023 to 2028.
This demonstrates how banking and other financial institutions can use predictive analytics to obtain insightful knowledge about future trends, consumer behavior, and market dynamics in order to make proactive, well-informed, and smart business decisions.
Furthermore, the importance of technology—particularly AI, blockchain, cloud computing, and data and predictive analytics tools—in achieving efficiency and spurring business innovation in the financial sector is highlighted in the recently released Mckinsey report, “Autonomy of AI: Staying on the forefront of AI in banking.” With the help of these technologies, banking institutions can provide remarkably efficient digital services to millions of individual and corporate clients. Their advantage in catering to a large audience is further enhanced by the cheap cost of IT operations and maintenance per account.
By utilizing data analytics and cloud computing, the financial services sector can create better risk models. It results in the previously unthinkable procedure of quickly assessing financial risks and approving loan products for customers.
Financial firms can also develop a profitable and economically sustainable company model by effectively handling credit requests and keeping expenses and returns in check. The highlighted instances demonstrated how adopting technology-driven solutions can change conventional banking procedures and financial processes, increasing the efficiency and accessibility of financial services for a larger audience.
Advanced Data Analytics for improved decision-making
Enhanced Decision-Making with Advanced Data Analytics
FinTech companies may create a holistic data strategy that includes prescriptive, predictive, and descriptive analytics by putting AI-driven insights into practice. Each of these analytical methodologies gains greater capabilities and data confidence with the application of machine learning and artificial intelligence.
Financial institutions are currently adopting analytics automation tools and predictive modeling to automate data processing. The finance team’s data strategy may include any or all of the following, depending on the business perspective:
Using descriptive analytics, one may turn raw data—like sales numbers, counts, and customer information—into useful knowledge that can help in decision-making. Analyzing historical data gives fintech companies insights into prior performance and patterns.
Predictive analytics forecasts future events and results by utilizing cutting edge technology such as artificial intelligence, machine learning, and big data. Predictive analytics helps companies to forecast future trends, consumer behavior, and market dynamics by finding patterns and connections in data.
Prescriptive analytics advances the analysis by offering astute suggestions and the best ways to proceed in order to attain the intended results. Prescriptive analytics uses algorithms and a variety of data inputs to help businesses solve problems and make data-driven decisions.
Prescriptive and predictive analytics provide deep insights into the problems facing the FinTech industry. They offer recommendations on the best ways to deal with them. In a market that is changing quickly, these analytics approaches help firms maximize performance, increase efficiency, and seize opportunities.
Real-time analytics is another kind of analytics that is very important to the banking and finance industry. The process of analyzing data and statistical models and producing insights in real-time or almost real-time is known as “real-time analytics.” It entails watching over and analyzing data streams as they come in, giving FinTech companies the ability to make decisions and take action right away.
FinTech Gains from AI-Driven Insights
Natural language processing (NLP), voice-script analysis, virtual agents, computer vision, facial recognition, blockchain, robotics, and behavioral analytics are some of the so-called “edge technologies” that fall under the category of AI-powered ones. Through a variety of means, including engagement channels, intelligent advice, and quicker processing, these sophisticated capabilities are essential in improving customer experience and loyalty. They also make customized offerings with accurate underwriting possible. Not to mention, they promote operational efficiency along the whole value chain. They include monitoring, record keeping, customer service, and more.
A McKinsey analysis states that financial institutions may automate more than 20 decision-making processes across multiple client journeys by prioritizing AI/ML use cases. Notably, banks can improve important areas by utilizing AI and analytics skills more and more.
acquiring customers, making credit judgments, keeping an eye on receivables, fostering stronger bonds with them, and providing astute servicing.
Predictive capabilities in AI and machine learning have several advantages for the financial industry. These advantages include:
- Enhanced Customer Acquisition: Banks may provide outstanding customer experiences and highly personalized communications to customers at every stage of the customer acquisition process by utilizing end-to-end automation and powerful analytics. They have a competitive advantage thanks to this strategy, which boosts their client acquisition rates.
- Enhanced Customer Lifetime worth: By proactively and persistently interacting with clients on a variety of goods and services, the client-bank relationship is reinforced, hence raising the client’s lifetime worth.
- Enhanced Operational Efficiency: Banks can drastically reduce their operating expenses by fully embracing automation, particularly in the document processing, review, and decision-making phases throughout acquisition and servicing.
- Reduced Credit Risk: By using more sophisticated methods to screen prospective clients and identify behaviors that point to a higher risk of fraud and default, banks can successfully reduce credit risks and create a more stable and secure financial environment.
Additionally, by using AA/ML models that can analyze large and diverse datasets in near real-time, banks can effectively evaluate potential new clients for credit services, set reasonable loan limits and rates, and improve their visibility into the future results of cash inflows and outflows with cash flow forecasting features.
Banking industry applications of predictive analytics
One aspect of advanced analytics is predictive analytics, which uses data mining, statistical modeling, historical data, and machine learning to predict future events.
Predictive analytics is commonly used by finance leaders to identify patterns in data that may be used to identify possible dangers and opportunities. Frequently associated with big data and data science, modern businesses struggle with an abundance of data that spans from log files to pictures and videos dispersed throughout many organizational repositories. Thus, by utilizing deep learning and machine learning algorithms, data scientists can successfully identify patterns and predict future events by extracting these insightful knowledge from information reservoirs. The foundation of this predictive process is formed by well-known statistical techniques including decision trees, neural networks, and logistic and linear regression models. These techniques produce initial predictive learnings that drive subsequent predictive insights and financial decisions. We’ll look at a few examples of predictive analytics’ application in financial services below.
Risk assessment and credit scoring in finance
Banks assess prospective borrowers’ creditworthiness using analytics and a predictive model. Predictive models analyze past data, credit ratings, financial behavior, and other pertinent aspects to determine the probability of loan default or repayment, allowing banks to make well-informed lending decisions.
How is credit risk management managed by banks?
Banks use a variety of strategies to efficiently control credit risk. They set specific lending requirements, which could include requiring borrowers to have a certain minimum credit score. They also keep a regular eye on their loan portfolios, assessing changes in the creditworthiness of their customers and making adjustments as needed.
Lenders use a variety of data from the borrower, the lender, and outside sources such as credit agencies to determine the risk cost. Among the techniques used are credit ratings and credit risk analysis models, which allow lenders to project the expected loss based on variables including exposure at default, loss-given default, and default likelihood.
By using AI-powered credit risk analysis, lenders can reduce risks and improve the overall quality of their loan portfolio by making better decisions about loan applications, spotting subtle patterns, and more accurately predicting probable defaults.
How can you increase your revenue significantly by optimizing credit scoring?
We covered practical uses of AI and how to turn your credit score into a profitable powerhouse in a free webinar on the usage of AI in the banking and financial industries. A case study of NextBank’s mobile banking is presented in the webinar.
Detecting Fraud with AI and ML
Financial institutions can quickly identify fraudulent activity by using predictive analytics. Banks are able to protect their reputation and consumer assets by identifying suspicious transactions and stopping fraudulent activity through ongoing analysis of transactional data and customer behavior patterns.
Five best practices to guarantee the proper use of AI and ML in managing financial crime risk were outlined by the Wolfsberg Group in 2022. The Wolfsberg Group is an alliance of thirteen international banks that works to produce frameworks and guidelines for addressing financial crime risks.
These procedures involve laying out precise guidelines for the usage of AI tools and putting governance measures in place to reduce the possibility of abuse while taking algorithmic bias and data theft into account. It emphasizes the necessity of using AI’s capability proportionately and doing regular risk assessments to weigh advantages like fraud detection and risk-based alert prioritization. Furthermore, sufficient design and technological know-how are necessary to comprehend the workings of AI, specify goals, account for limitations like bias, and provide explainability for researchers.
In addition, the paradigm emphasizes ethical checks and compliance alignment along with accountability and oversight throughout the lifecycle of AI, especially when employing vendor-provided AI. Maintaining openness and guaranteeing a strong audit trail need AI systems to give explicit explanations for risk detection choices in order to strike a compromise between the demands of secrecy and transparency placed on them by regulators.
Defending bank customers against possible fraud attempts: a NextBank case study
The progressive financial firm NextBank saw the potential of a safe and easy-to-use mobile application. NextBank developed a mobile app that satisfies strict security standards and offers a smooth banking experience because it is dedicated to giving its customers unmatched security and convenience.
NextBank used a multi-layered strategy that included industry best practices and the most recent security protocols. By putting in place rigorous authentication protocols and end-to-end encryption, they gave users confidence and encouraged them to transfer money with confidence.
In order to adhere to the stringent OWASP Application Security Verification Standards, the Nextbank development team selected an affordable, safe banking application. The highest standards of data security and protection were therefore guaranteed. The case study from NextBank shows how they go above and beyond to shield their customers from possible fraud attempts.
AI-powered customer segmentation and personalisation in finance
Customers are categorized using predictive analytics according to their needs, preferences, and behaviors. Banks can provide tailored financial goods and services, improve client experiences, and foster customer loyalty by comprehending different customer segments. Nonetheless, it is the duty of banks and financial institutions to develop methods that accomplish various segmentation objectives while abiding by established legal terms and compliance guidelines.
Financial institutions can benefit from efforts in segmentation and personalization across marketing and sales channels by assembling information from many campaigns and touchpoints to form a coherent story. Secondly, producing customized blog posts that are aimed at specific clientele groups according to their product interests and usage. The last step is to divide up the consumer base according to the stages of their purchasing cycle and then adjust marketing to each stage of the trip. These strategies use data-driven content development and marketing analytics to improve consumer experiences and build stronger bonds.
Examples include proactive fraud detection, tailored financial advise, and the analysis of consumer data to provide individualized customer support. Additionally, AI can aid in the automation of repetitive operations, boosting productivity and cutting expenses for banks all the while delivering quicker customer service. Finally, conversational banking can be facilitated by AI. When it comes to streamlining user interactions and providing multilingual financial services, it is unmatched.
Forecasting Churn and Retention of Customers
Banks can identify clients who are likely to close their accounts or churn by using predictive algorithms. Numerous variables can be used by the churn prediction algorithm to provide predictive analytics in the financial sector. Customer journeys, price information, transactions, economic variables, customer behavior aspects, and consumer demographics and psychographics are some of the significant factors.
By acting proactively and providing customized incentives or individualized offers, banks can decrease attrition rates and retain key customers.
Investment opportunities and an examination of market trends
Financial institutions can forecast changes in market conditions and analyze market trends with the aid of predictive analytics. These insights help with portfolio adjustments, capitalizing on new possibilities, and making strategic investment decisions.
Furthermore, through the analysis of past data, industry trends, and customer behavior, predictive analytics enables financial organizations to spot investment opportunities. Organizations can choose their investments with data-driven decision-making by comprehending the dynamics of different financial instruments and asset classes. In order to increase returns, they can also optimize their portfolios and take advantage of new trends.
Opportunities for Upselling and Cross-Sell
Banks can find chances for upselling and cross-selling to their current clientele by using predictive analysis. Banks are able to suggest appropriate financial products and services by analyzing consumer data and past purchases. and consequently boost sales and client involvement.
Optimization of Portfolios
Predictive models suggest the best asset allocations for enhanced investment portfolios based on risk appetite, past market data, and client objectives. The optimization of a portfolio offers significant benefits to managers and investors. In order to achieve a desired risk-return trade-off on the efficient frontier, it seeks to maximize returns while skillfully controlling risk. Client satisfaction is increased as a result of the high returns per unit of risk.
Second, diversity is emphasized by portfolio optimization, which lowers unsystematic or non-priced risks and shields investors from the negative effects of underperforming assets. Even in volatile market conditions, investors can stay comfortable with a well-diversified portfolio. Managers can also spot market opportunities before others do thanks to active portfolio management and market data research. Additionally, it enables them to take advantage of these chances to maximize returns for their investors.
Adherence to Regulations
Regulatory compliance is ensured by banks with the help of predictive analytics. Banks that analyze large amounts of data can stay out of trouble and comply with regulations. They make use of this information to spot possible compliance issues and prepare ahead of time.
By classifying, categorizing, and indexing vast amounts of data and documents, AI-powered solutions can effectively organize them. This feature is especially helpful for adhering to regulations. Financial organizations can quickly recognize and keep an eye on particular transactions, clients, or patterns of behavior thanks to it. Furthermore, subjectivity is eliminated from data structures by AI, guaranteeing uniformity across departments and making comparisons simple. This considerably lowers the possibility of mistakes or omissions in compliance reports.
Financial Forecasting with Predictive Models
Financial forecasting has become a dynamic, data-driven discipline thanks to predictive models, which have given firms access to previously unobtainable information. Professionals in business and finance can’t live without these models. In an uncertain financial environment, they aid in revenue forecasting, credit risk assessment, and client understanding.
The application of AI in financial forecasting and decision-making Industry executives have taken notice of AI’s capacity to analyze enormous amounts of data, find complex patterns, and produce predictions. Experts highlight how artificial intelligence (AI) might improve financial forecasting by delivering more accurate and timely insights. Furthermore, AI plays a bigger part in assisting humans in making decisions. In cases with a lot of data and complexity, AI can greatly enhance decision outcomes when paired with human knowledge.
Financial institutions obtain a competitive edge by utilizing predictive analytics driven by artificial intelligence. Forecasts powered by AI offer vital information about consumer behavior, supplier networks, market trends, and risk management. Additionally, they facilitate quick and informed decision-making. AI’s analytical powers combined with human experience bring in a new era of efficiency and strategic advantage. Additionally, it drives the banking and finance industries toward success and long-term expansion.