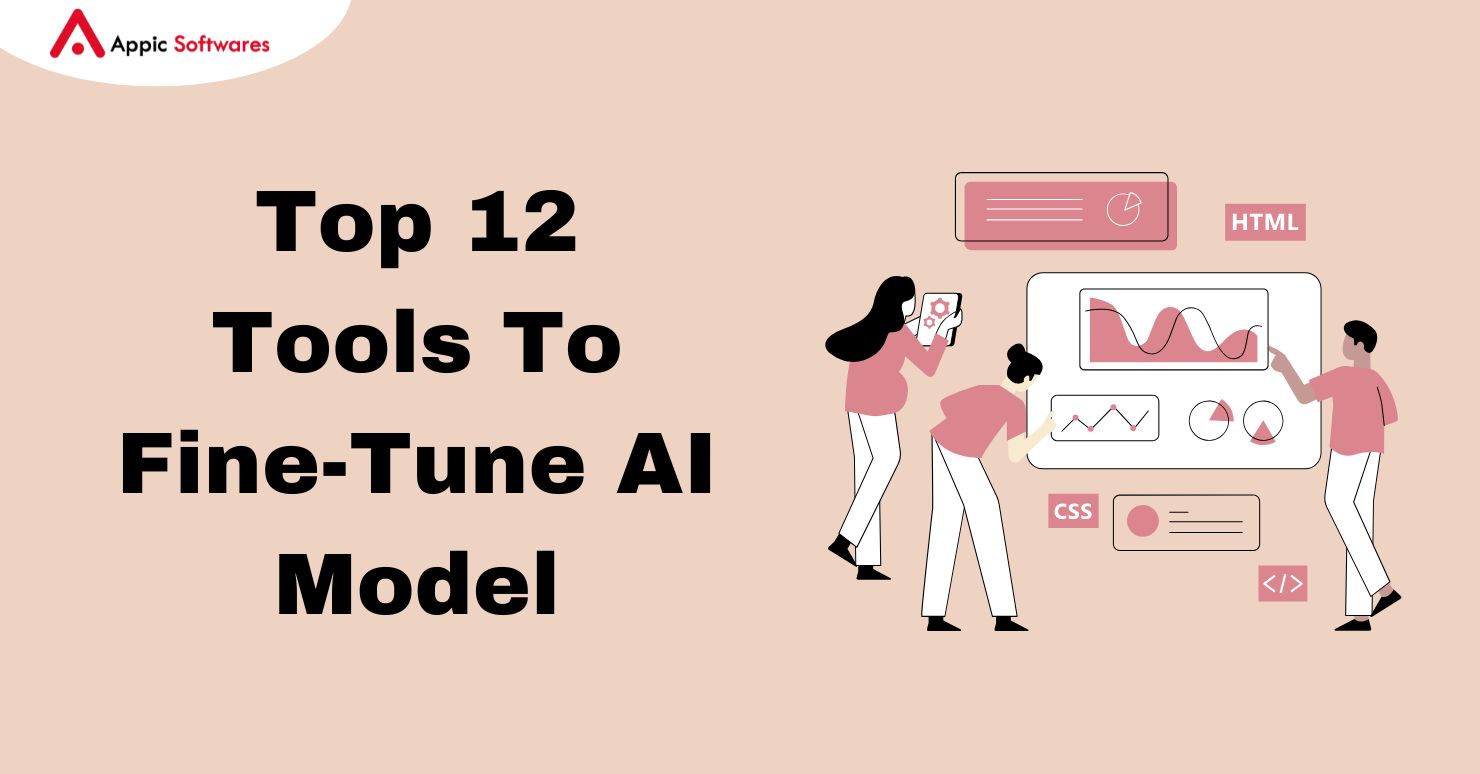
Over $390 billion is the estimated value of the worldwide AI market. Over the next five years, the value of the AI sector is expected to grow by more than five times. By 2026, the US AI market is expected to grow to $299.64 billion. Fine-tuning AI models can help you get better results for your tasks. In this article, you will learn about Tools To fine-tune AI Model and how to use them. You also see why you should fine-tune AI systems. And you see how to hire dedicated AI experts for this work. You’ll find easy tips and in-depth information here. You get clear steps you can follow.
Why To Fine-Tune an AI Model?
Fine-tuning AI models allows you to shape a general model for your specific data. It can cut errors and boost performance. You train a base model on your own samples. Then you test and adjust. You see what works and what needs to change. This gives your app more relevant answers. You can add new skills or knowledge in a niche topic. It can spot terms or names in your field. It can mirror your tone or style. And it can fit the rules you set. All this makes your model more useful. It makes sure you get the value you need.
Key Benefits of Fine-Tuning AI Model
Fine-tuning the AI model brings clear perks:
- Better accuracy. Your model learns from your data.
- Faster learning. You need less new data.
- Cost saving. You use a pre-made model. By using a pre-made model, you can significantly reduce the AI app development cost, as fine-tuning optimizes performance without requiring extensive resources from scratch.
- Brand fit. You shape the tone and style.
- Control. You set rules on output and style.
These make fine-tuning a smart move for many teams.
Best Tools To Fine-Tune AI Models
Fine-tuning is a critical step in the AI software development process, enabling models to adapt to specific needs. Fortunately, there are several top-tier tools available today to streamline this task. These tools allow you to monitor changes and select the best fit for your project. Here’s a look at some of the most effective options:
OpenAI Fine‑Tuning
OpenAI Fine‑Tuning lets you train a GPT model on your own examples. You prepare a set of prompts paired with the ideal replies. Then you upload that data to OpenAI’s API. You pick a learning rate and choose how many examples each batch will hold. Next, you start the tuning run. The system learns your style. It studies your terms. In a few minutes, you will get back a new model ID.
Key Features
- Easy data upload via API
- Custom learning rate and batch size settings
- Cloud computing with no server setup.
- Versioned model IDs for simple switching
- Built‑in logs for loss, accuracy, and token usage
- Dashboard for test metrics and comparisons
- Pay‑as‑you‑go pricing
Hugging Face Transformers
Hugging Face Transformers gives you a library of models ready for fine-tuning. You install it in Python. Then you load a base model. That could be BERT, GPT-2, GPT-3, T5, or more. You add a task head or use a prompt template. Next, you call the Trainer class. You pass your dataset. The library handles batching, learning rate schedules, and checkpoint saving. You can tune epochs and warm‑up steps in a few lines of code.
Key Features
- Wide range of pre‑trained models (BERT, GPT, T5, etc.)
- Trainer class for end‑to‑end fine-tuning
- Configurable epochs, batch size, and schedulers
- Mixed precision and multi‑GPU support
- Easy push to Hugging Face Hub with model card
- Built‑in metrics logging and visualization
- Compatible with PyTorch and TensorFlow
Weights & Biases (Wandb)
Weights & Biases helps you track experiments and monitor fine-tuning work. You add a few lines of code to log metrics as your model trains, and you see loss, accuracy, and any custom metric you choose. You can also log images, text samples, and model gradients. The web dashboard shows charts that update in real time. You can compare runs side by side.
Key Features
- Real‑time logging of loss, accuracy, and custom metrics
- Chart dashboards for run comparison
- Alerts on metric thresholds
- Checkpoint and data set versioning
- Project and team management with access control
- Integrations with Hugging Face, OpenAI, and more
- Exportable reports for stakeholders
Azure AI Studio
Azure AI Studio offers a web interface to prepare data, fine-tune, and test models. You start by selecting an existing Azure OpenAI or custom model. Then you upload your training file in CSV, JSON, or Parquet format. You map your columns to input and output fields. Next, you set the tuning options—things like learning rate, batch size, and number of epochs. You kick off the run with one click. The studio shows you build logs, run time, and cost estimates.
Key Features
- Web UI for data mapping and tuning
- One‑click run launch with cost estimate
- Detailed training and validation reports
- Batch testing and hosted API options.
- Integration with Blob Storage and Azure Functions
- CI/CD support via Azure DevOps and GitHub Actions
- Pay‑as‑you‑use compute scaling
Google Cloud Vertex AI
Google Cloud Vertex AI spans the full AI pipeline from data prep to model deployment. You import your data from BigQuery, Cloud Storage, or your local file. Next, you set budget caps and tune parameters such as learning rate and early stopping criteria.
Key Features
- Data import from BigQuery, Cloud Storage, and local files
- Choice of AutoML or custom training containers
- Flexible compute options with GPUs and preemptible VMs
- Detailed job logs, metrics, and cost tracking
- One‑click deployment for online and batch endpoints
- Auto‑scaling and drift detection
- Integration with Dataflow, Looker, and Vertex Pipelines
Anthropic Claude Fine‑Tuning
Anthropic Claude Fine‑Tuning lets you adapt Claude models to your needs. You prepare examples of user prompts and the preferred replies. You load them into a JSONL file. Then you upload that file to Anthropic’s API. You set steps, batch size, and learning rate in the request. The system queues your job in their cloud. After it runs, you get a fine-tuned Claude handle. You call that handle in your code just like base Claude.
Key Features
- Simple JSONL-based example upload
- Configurable steps, batch size, and learning rate
- Safety and bias checks are built in
- Fine-tuned model handle for easy API calls
- Version rollback support
- Console for output preview and safety scores
- Pay‑per‑use pricing
Langchain
Langchain is a framework that lets you link LLM calls, prompts, and tools into workflows. You start by installing it in Python. Then you pick a model provider—OpenAI, Anthropic, or a local model. You write sequences that call the model, then parse the output. For fine-tuning, Langchain offers helper classes. You feed it a set of examples. It builds prompt templates that call the tuned model.
Key Features
- Prompt and agent orchestration framework
- Support for multiple model providers
- Classes for building fine-tuning workflows
- RAG support with vector store connectors
- Memory modules for stateful apps
- Version tracking for model calls
- Reusable chains and templates
LlamaIndex
LlamaIndex helps you prepare data for large language models. You can feed it documents, tables, or code files. It builds an index that you query in your code. For fine-tuning, you use that index to create training examples. You pull similar documents based on a query. Then you pair them with a target response. You get consistent examples that reflect your data. LlamaIndex handles chunking, text splitting, and embedding storage.
Key Features
- Document, table, and code ingestion
- Built‑in text splitting and chunking
- Metadata filters for targeted indexing
- Integration with multiple vector stores
- Generation of training examples
- Export to JSONL or CSV for tuning
- RAG testing helpers
Chroma
Chroma is a simple vector database for storing embeddings. You generate embeddings from your data with any open tool. Then you add them to Chroma along with text or metadata. Chroma gives you fast similarity search via an API. You can test how context changes output by fetching top‑k similar items in your fine-tuning loop. This helps you build training sets that reflect real use cases. Chroma also supports filters on metadata. You can run searches like “show me the five most recent samples with label X.”
Key Features
- Fast similarity search API
- Metadata filters for targeted queries
- Collection versioning for reproducibility
- SDKs for Python, JavaScript, and Go
- Support for CPU and GPU
- Docker and Kubernetes deployments
- Query time and data metrics
Qdrant
Qdrant is a full‑featured vector database built for scale. It stores embeddings and rich metadata. You can search by distance metrics and apply complex filters. You can mix vector queries with exact filters like “status = active.” Qdrant offers real‑time syncing across nodes. It can handle millions of vectors with low latency. It also tracks usage metrics and query stats. For fine-tuning, you use Qdrant to build data sets that mirror production lookups. You fetch similar items, then pair them with your labels to form a training batch. This ensures your examples match real app behavior. Qdrant has a web UI so you can inspect your collections and queries.
Key Features
- Advanced filters combining vector and exact matches
- Real‑time syncing and low‑latency queries
- Support for millions of vectors
- Web UI for collection and query inspection
- Client libraries for major languages
- On‑prem and cloud deployment options
- gRPC‑based ingest and query
vLLM
vLLM is a high‑performance engine for running LLM inference. It optimizes memory and compute use to serve many requests at once. You fine-tune your model with another tool, then deploy it on vLLM to test it under load. vLLM batches requests and reuses computation when possible. It offers a REST API you can call from any client. You can measure latency, throughput, and GPU utilization. You can tune its internal settings, like batch size and prefetch queues. vLLM also supports quantized models for lower memory use. This helps you spot bottlenecks in your deployment. vLLM runs on Linux with Docker or directly on the host.
Key Features
- Request batching and compute reuse.
- REST API for easy integration
- Tunable batch size and prefetch settings
- Support for quantized models
- Metrics on latency, throughput, and GPU use
- Docker and native Linux deployment
- Logs for error rates and delays
BentoML
BentoML helps you package and ship machine learning models. After you fine-tune a model, you write a simple Python script that loads it. Then you add API routes for inference. You define input and output schemas. Next, you run bentoml build. This creates a deployable bundle with your code, model, and dependencies. You can containerize it with one command. Then you deploy it to AWS, Azure, GCP, or any Kubernetes cluster. BentoML also adds logging hooks. You can log each request’s payload and response. You get health checks and metrics out of the box.
Key Features
- Simple Python API for model and route definition
- Automatic build of the container with code and dependencies
- Built‑in logging, health checks, and metrics
- Support for multiple models in one service
- One‑command deploy to cloud or Kubernetes.
- Test UI for quick endpoint checks
- Consistent bundle for dev and production environments
Hire Dedicated AI Developers For AI Model Fine-Tuning
When you need deep help, you can hire AI experts. You get full support for fine-tuning AI. You also get code reviews and advice.
What to Look For
You need people who know machine learning and coding. They should know Python and major AI frameworks. They should have done fine-tuning AI work. Ask for samples of past projects. Also, check if they can explain results clearly. You want them to run tests and share reports. They should know how to log jobs. And how to track model drift. They should write clear code and docs. A good developer can set up your Tools to fine-tune the AI Model workflow fast. Finding the right talent is key. An AI development company can offer professionals skilled in machine learning, coding, and major AI frameworks like Python.
How to Hire
You can use job boards or contacts. You can post tasks on gig sites. Create a clear job post, list tasks such as data preparation, model tuning, and testing. Ask for a plan with steps and costs. Set a trial task first. Then you can scale work if that goes well. Pay by project or by hour. Use clear contracts and milestones. For projects focused on interactive or autonomous systems, an AI agent development company can bring specialized knowledge in building and fine-tuning models for agent-based applications, such as chatbots or virtual assistants.
Conclusion
Fine-tuning can make your AI work smart and fit your needs. You pick from many Tools To fine-tune AI Model options. You use OpenAI Fine‑Tuning or Hugging Face for code work. And you can wrap it all with BentoML. If you need help, hire dedicated pros. They can set up and run your job. Now you know why you should fine-tune your AI model and which tools to use. And you know how to hire help. Start your work today and see better results on your data. Appic Softwares can help you fine-tune AI models that fit your exact needs. From selecting the right tools to model deployment, we’ve got you covered.
Get in touch with us today and let’s optimize your AI solution!
FAQs
1. How much data do I need to fine-tune an AI model?
Fine-tuning typically requires fewer data samples than training from scratch. Depending on the model and task, a few hundred to a few thousand high-quality examples can be sufficient. Tools like OpenAI and Hugging Face provide clear guidelines on dataset formats and minimum sizes.
2. Can I fine-tune AI models without deep technical knowledge?
Yes, many platforms such as Azure AI Studio, OpenAI, and Google Cloud Vertex AI offer intuitive UIs and low-code options. However, for complex customizations, hiring AI experts is recommended to ensure accuracy and efficiency.
3. Is fine-tuning the same as prompt engineering?
No. Fine-tuning involves training the model on your dataset to improve performance, while prompt engineering is about crafting better input prompts to guide the model’s response without changing its internal parameters.