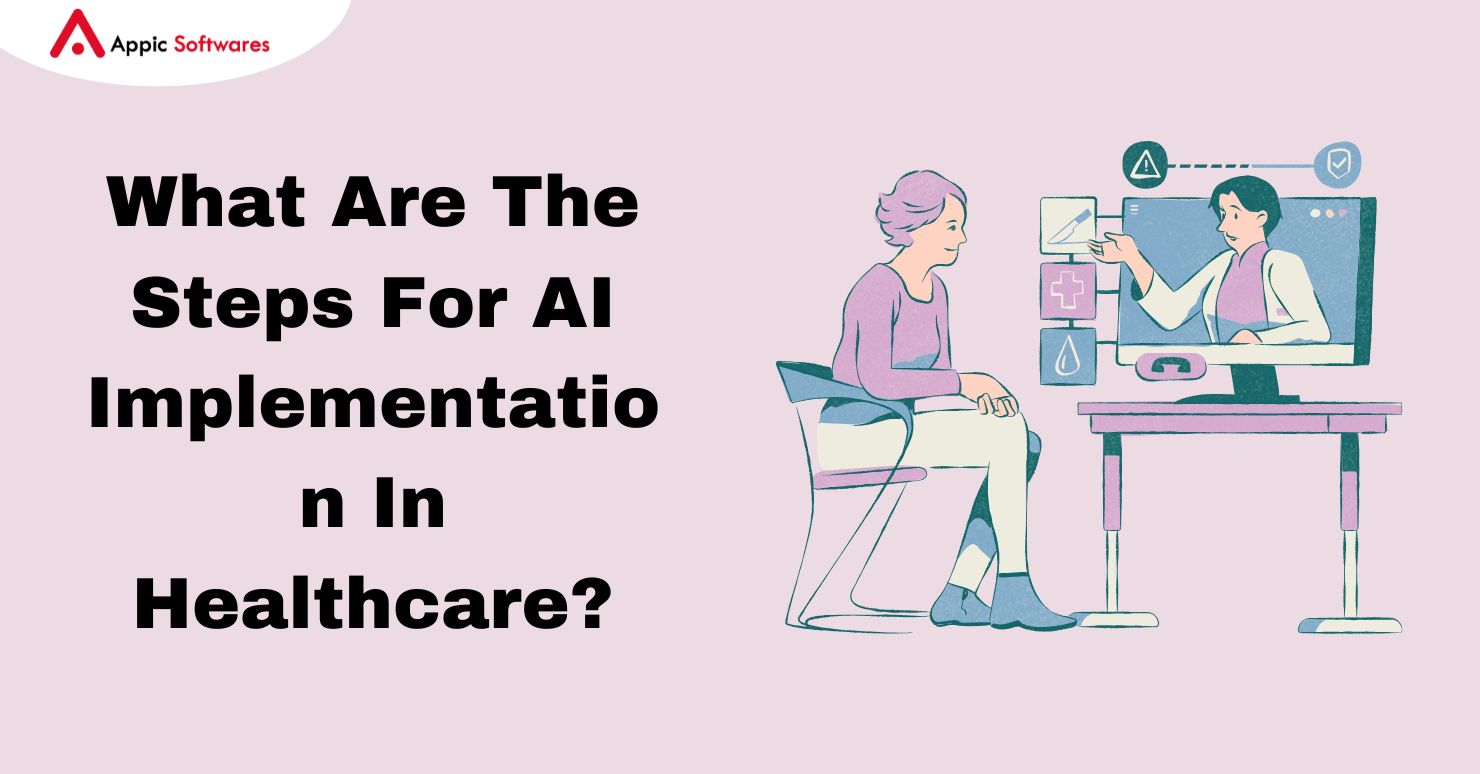
AI implementation in healthcare means using smart software to help doctors, nurses, and staff. It can learn from data. It can spot patterns in scans and records, speed up tasks, and cut mistakes. By 2026, the US healthcare industry might save $150 billion annually thanks to important clinical health AI applications.
The steps for AI implementation in healthcare start with a clear goal. Then they move to data care. Teams must test the software. They must train staff. They must watch the results. This blog covers the benefits of AI implementation in healthcare. It then shows a step-by-step process to implement AI in healthcare.
What Are The Benefits Of AI Implementation In Healthcare?
AI implementation in healthcare can bring fast, clear gains for patients and teams. The right plan and care keep work safe and fair.
-
Better And Faster Diagnosis
AI can scan images like X‑rays and MRIS in seconds. It can mark spots that look odd. It can learn from past cases to point to a rare illness. Good AI implementation in healthcare can reduce errors and speed up work. Teams can catch a disease at an early stage. They can start treatment sooner.
-
Faster Treatment And Care
AI tools can sort lab results and notes in minutes. They can flag tests that need urgent review. They can guide care plans. It can predict which patient needs care first. And they can send alerts to nurses. This cuts wait time. It helps staff focus on the sickest patients.
-
Lower Cost
AI can cut waste in tests and scans. It can speed up report prep. It can auto‑fill parts of the record. The implementation of AI in healthcare cuts the time staff spend on billing and claim checks. It can cut staff hours on routine tasks. So the cost per patient can drop. While there is an initial AI app development cost, the long-term savings from improved efficiency and fewer errors make it a worthwhile investment, lowering the overall cost per patient.
-
Personalized Patient Plans
AI can group patients by age, history, and risk. It can track responses to drugs and note side effects. It can adjust a plan if the patient needs a change. And it can send simple reminders for meds and visits. This makes the plan fit each person.
-
Data Review And Trend Spotting
AI can sift through thousands of records at once. It can spot a rise in a symptom or count of a side effect, flag a drug interaction, and group data from many clinics. This helps track local outbreaks or side effects fast. It can guide public health teams to act.
Step-By-Step Process For AI Implementation In Healthcare
The step-by-step process for AI implementation in healthcare has clear stages. It moves to data care. It ends with live use and review. Each stage needs the right team and tools.
-
Define The Clinical Need
First, list key goals. For example, you may want faster image review or better risk prediction. You may want to cut chart errors or auto‑fill fields and must set a clear aim. You must know what success looks like and link it to patient safety and value.
-
Gather Quality Data
Next, collect patient records, scans, reports, and logs. You need clean data and must remove errors and gaps. Also, you must label images and notes so the AI can learn. When implementing AI in healthcare, you need good data. Data from many sources can boost accuracy.
-
Choose The Right Technology
Then, pick a software or build a model. Select software or develop a custom model, using open-source tools, vendors, or partnering with an AI development company for a tailored solution. You must check that the tool meets health rules. Look for models made for scans or for notes. Check encryption and data controls. Keep the tool simple and clear.
-
Train And Test The Model
Next, feed the data to the model. Let it learn to spot patterns. This involves the AI software development process, where iterative training refines accuracy. Test it on new data it has not seen. Check if it marks the right areas in an image. Check if it flags the right risk level in a chart. Track its error rate. You aim for high accuracy and low false flags.
-
Validate Safety And Rules
Then, review the results with clinicians. Let doctors and staff test the tool. Get their feedback. Check if the tool meets local health rules. Check if it meets privacy laws. Update the tool if it shows bias or error.
-
Deploy In A Real Setting
Next, install the tool in the clinic or hospital system. Link it to the record system. Train staff on its use. Show them how to read alerts. Show them how to report errors or biases. Give quick guides and live support.
-
Monitor And Update
Finally, watch the tool in live use. Track its success on key goals. Track any error or bias over time. Update the code or model as you get more data. Review every few months. Make sure it still meets safety rules.
What Are The Applications Of AI In Healthcare?
The applications of AI implementation in healthcare start with image analysis. They move to risk checks, chat help, drug search, and task work. Each use can boost care quality and cut waste.
Image And Scan Analysis
AI can help doctors read scans. It can spot nodules, fractures, or blockages in X‑rays, CT scans, and MRIS. Then it marks spots that need review. In studies, AI found lung nodules as well as a human reader. This cuts review time in half and lowers errors. It also works around the clock and sorts scans by urgency. That way, critical cases get flagged fast and staff can treat them sooner. AI tools can track changes over time, measuring things like tumour size.
They can read eye scans to catch retinopathy early or skin images for odd moles. They even review pathology slides in labs and rank cases for human review. By linking to patient notes, AI adds context to each finding. Plus, the model can update itself as new data arrives, making it more accurate over time.
Predictive Risk Assessment
AI can look at a patient’s data age, history, lab tests, and notes, and spot the risk of a crisis. It then gives a simple score for issues like heart attack, sepsis, or readmission. Clinicians use this score to act early. If a score is high, they can check the patient more often. If it’s low, they can plan a safe discharge. This cuts readmissions and lowers costs. It also helps staff focus on those at most risk. AI Agent in Healthcare spots trends across many patients, like if a drug raises a certain risk or if a lab value signals decline.
It can send real‑time alerts to phones or dashboards. Teams review these alerts and adjust the care plan. Over time, the model learns from each case and grows more precise, so the score fits each person better.
Virtual Health Assistants
AI chat tools can talk to patients. They answer common questions, set up visits, and remind patients to take meds. They check on someone after a hospital stay and free nurses for more skilled work. For example, a chat tool might text “Did you take your pill today?” If the patient says no, it alerts the care team or sends a how‑to video link.
These tools work any time, even at night, and can speak many languages. They learn from each chat and keep a log that syncs with the main record system, so no info is lost. They also screen patient needs: urgent issues trigger a call, while routine matters book a regular visit. This makes care smoother and more patient‑friendly. AI chat tools, often built by specialised AI agent development companies, interact with patients, answering questions, scheduling visits, and sending reminders.
Drug and Therapy Search
AI scans medical journals, trial reports, and drug databases in seconds. It sorts trials by date, size, and result quality. Then it extracts details on side effects and success rates. Next, it links each drug to patient traits like age, weight, and history. For example, it can match a drug dose to a patient with kidney issues. It also checks for drug interactions by reading a patient’s current meds and flags any risky mix. It logs all findings in a clear table and shows links to the key studies.
Also, AI spots gaps in current treatments. It can run meta‑analyses in minutes instead of months. It can guide trial design by finding similar past studies and their outcomes. This tool gives researchers clear leads on the next steps. It helps clinicians pick the right therapy for each patient. And it speeds up the path from lab to bedside, so new treatments reach patients faster.
Admin Task Automation
AI fills chart fields by reading notes and lab results. It finds missing data points and adds them automatically. It sorts bills and claims, matching codes to services. Then it sends alerts if it spots errors or mismatches. Next, AI tracks staff hours and duty logs in real time. It logs who works when and flags overtime. It also auto‑routes lab orders to the right department.
Moreover, AI speeds up registration by pre-filling patient info. It checks each claim against payer rules and highlights any issues, batches and sends hundreds of claims at once, and runs daily audits to spot coding errors. It can even draft simple referral letters for review. This cuts routine work from hours to minutes. It frees nurses and admin staff for patient‑facing tasks. Plus, it keeps records neat and up to date. And it helps the whole team work more smoothly each day.
Conclusion
AI implementation in healthcare brings big gains. It can lift care quality, cut costs, spot risk, and boost safety. This guide on AI implementation in healthcare shows the benefits. It lays out a clear step-by-step process. It lists key uses in scans, risk checks, chat help, drug search, and admin work. When implementing AI in healthcare, you follow the steps above to reach real gains.
Partner with experts who understand both technology and patient care. Whether you’re looking to implement AI for diagnostics, risk prediction, or task automation, the right solution starts with the right app development company like Appic Softwares.
Contact us today to begin your AI healthcare journey—boost efficiency, cut costs, and deliver smarter care.
FAQs
1. What is AI implementation in healthcare?
AI implementation in healthcare means adding smart software to help with patient care and administrative tasks. It lets machines learn from data. Then they spot patterns in scans, records, and tests. This process aims to make care faster and safer. It also cuts routine work so staff can focus on patients.
2. How do I start implementing AI in healthcare at my clinic?
First, set a clear goal. For example, you might want faster image review or fewer chart errors. Next, gather clean data like scans and lab results. Then pick a tool that fits your needs and meets health rules. After that, train and test the model on your data. Finally, roll it out in a small pilot. If it works, expand step by step.
3. What data is needed for the implementation of AI in healthcare?
You need good‑quality data. This includes patient records, lab tests, and scans. It also needs clear labels (for example, marking a tumour in an image). Clean data shows the AI what to learn. The more varied the data, the better the model can handle real cases.
4. How do we protect patient privacy during AI implementation?
Use strong encryption when you store or share data. Limit who can see patient files. Keep logs of every access. Follow laws like HIPAA or GDPR if they apply. Also, run regular audits. And only feed the AI data it needs to learn, not extra personal details.
5. How long does it take to see benefits from AI implementation in healthcare?
A small pilot can show value in 3 to 6 months. You may spot faster scans or fewer errors sooner. For a full roll‑out across a hospital, plan for 12 to 18 months. This lets you refine the tool, train more staff, and prove real gains in care quality and cost.